Improving Monocular Depth Estimation by Semantic Pre-training
2021 IEEE/RSJ INTERNATIONAL CONFERENCE ON INTELLIGENT ROBOTS AND SYSTEMS (IROS)(2021)
摘要
Knowing the distance to nearby objects is crucial for autonomous cars to navigate safely in everyday traffic. In this paper, we investigate monocular depth estimation, which advanced substantially within the last years and is providing increasingly more accurate results while only requiring a single camera image as input. In line with recent work, we use an encoder-decoder structure with so-called packing layers to estimate depth values in a self-supervised fashion. We propose integrating a joint pre-training of semantic segmentation plus depth estimation on a dataset providing semantic labels. By using a separate semantic decoder that is only needed for pre-training, we can keep the network comparatively small. Our extensive experimental evaluation shows that the addition of such pre-training improves the depth estimation performance substantially. Finally, we show that we achieve competitive performance on the KITTI dataset despite using a much smaller and more efficient network.
更多查看译文
关键词
monocular depth estimation,pre-training
AI 理解论文
溯源树
样例
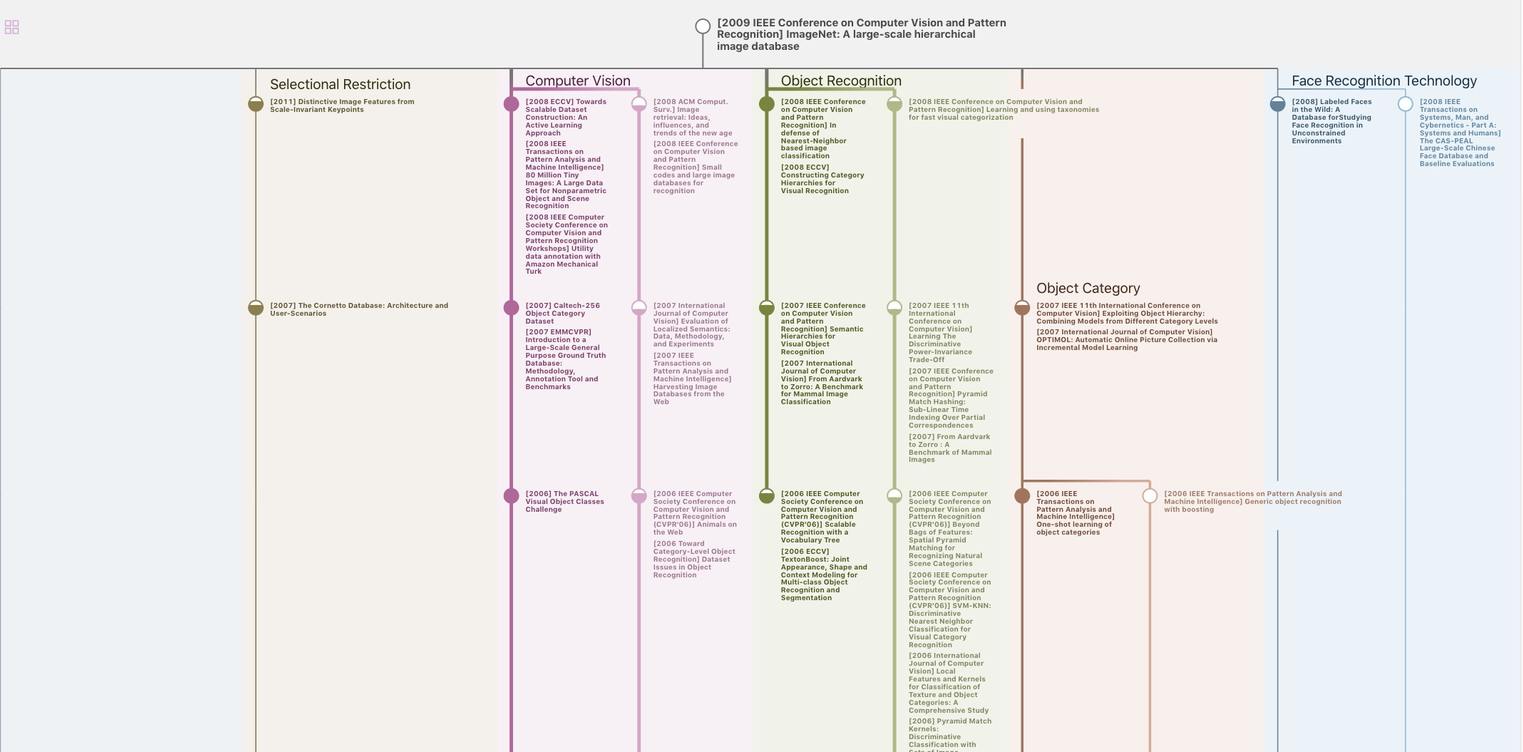
生成溯源树,研究论文发展脉络
Chat Paper
正在生成论文摘要