The Computational Drug Repositioning Without Negative Sampling.
IEEE/ACM transactions on computational biology and bioinformatics(2023)
摘要
Computational drug repositioning technology is an effective tool to accelerate drug development. Although this technique has been widely used and successful in recent decades, many existing models still suffer from multiple drawbacks such as the massive number of unvalidated drug-disease associations and the inner product. The limitations of these works are mainly due to the following two reasons: firstly, previous works used negative sampling techniques to treat unvalidated drug-disease associations as negative samples, which is invalid in real-world settings; secondly, the inner product cannot fully take into account the feature information contained in the latent factor of drug and disease. In this paper, we propose a novel PUON framework for addressing the above deficiencies, which models the risk estimator of computational drug repositioning only using validated (Positive) and unvalidated (Unlabelled) drug-disease associations without employing negative sampling techniques. The PUON also proposed an Outer Neighborhood-based classifier for modeling the cross-feature information of the latent facotor. For a comprehensive comparison, we considered 6 popular baselines. Extensive experiments in four real-world datasets showed that PUON model achieved the best performance based on 6 evaluation metrics.
更多查看译文
关键词
Algorithms,Drug Repositioning,Computational Biology
AI 理解论文
溯源树
样例
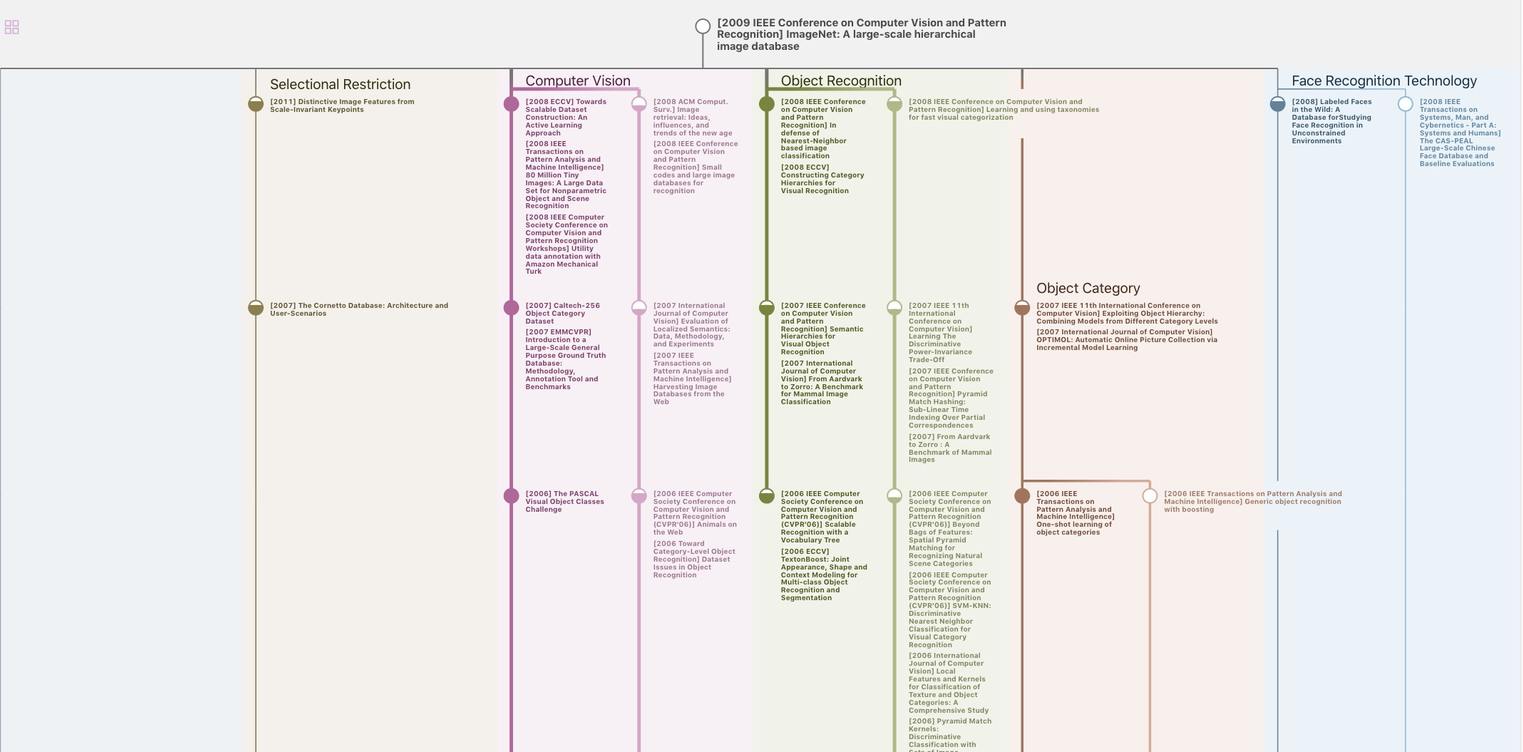
生成溯源树,研究论文发展脉络
Chat Paper
正在生成论文摘要