iLabel: Interactive Neural Scene Labelling
arxiv(2021)
摘要
Joint representation of geometry, colour and semantics using a 3D neural field enables accurate dense labelling from ultra-sparse interactions as a user reconstructs a scene in real-time using a handheld RGB-D sensor. Our iLabel system requires no training data, yet can densely label scenes more accurately than standard methods trained on large, expensively labelled image datasets. Furthermore, it works in an 'open set' manner, with semantic classes defined on the fly by the user. iLabel's underlying model is a multilayer perceptron (MLP) trained from scratch in real-time to learn a joint neural scene representation. The scene model is updated and visualised in real-time, allowing the user to focus interactions to achieve efficient labelling. A room or similar scene can be accurately labelled into 10+ semantic categories with only a few tens of clicks. Quantitative labelling accuracy scales powerfully with the number of clicks, and rapidly surpasses standard pre-trained semantic segmentation methods. We also demonstrate a hierarchical labelling variant.
更多查看译文
关键词
labelling
AI 理解论文
溯源树
样例
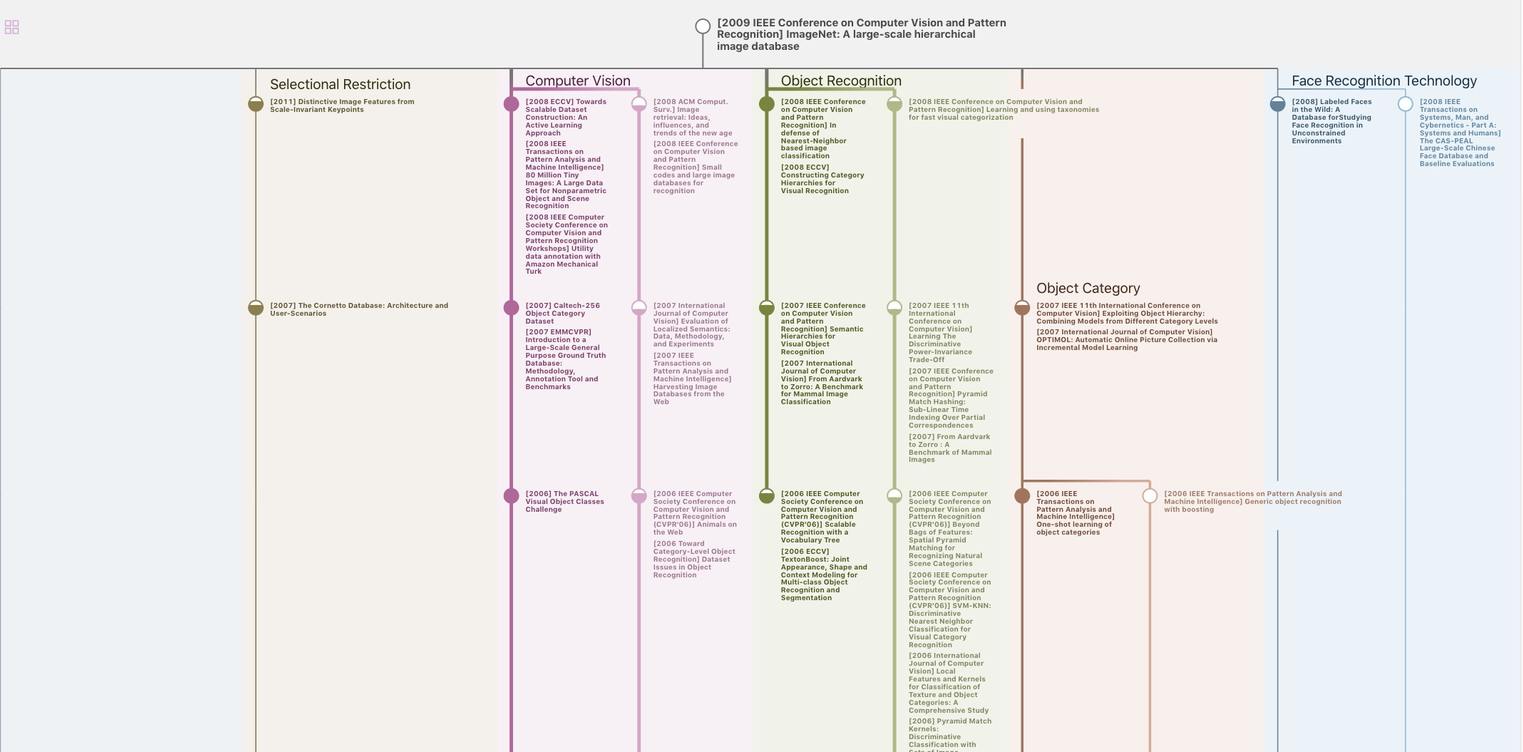
生成溯源树,研究论文发展脉络
Chat Paper
正在生成论文摘要