Is causal reasoning harder than probabilistic reasoning?
REVIEW OF SYMBOLIC LOGIC(2024)
摘要
Many tasks in statistical and causal inference can be construed as problems of entailment in a suitable formal language. We ask whether those problems are more difficult, from a computational perspective, for causal probabilistic languages than for pure probabilistic (or "associational") languages. Despite several senses in which causal reasoning is indeed more complex both expressively and inferentially we show that causal entailment (or satisfiability) problems can be systematically and robustly reduced to purely probabilistic problems. Thus there is no jump in computational complexity. Along the way we answer several open problems concerning the complexity of well-known probability logics, in particular demonstrating the there exists R-completeness of a polynomial probability calculus, as well as a seemingly much simpler system, the logic of comparative conditional probability.
更多查看译文
关键词
probability,causation,logic,complexity,ETR
AI 理解论文
溯源树
样例
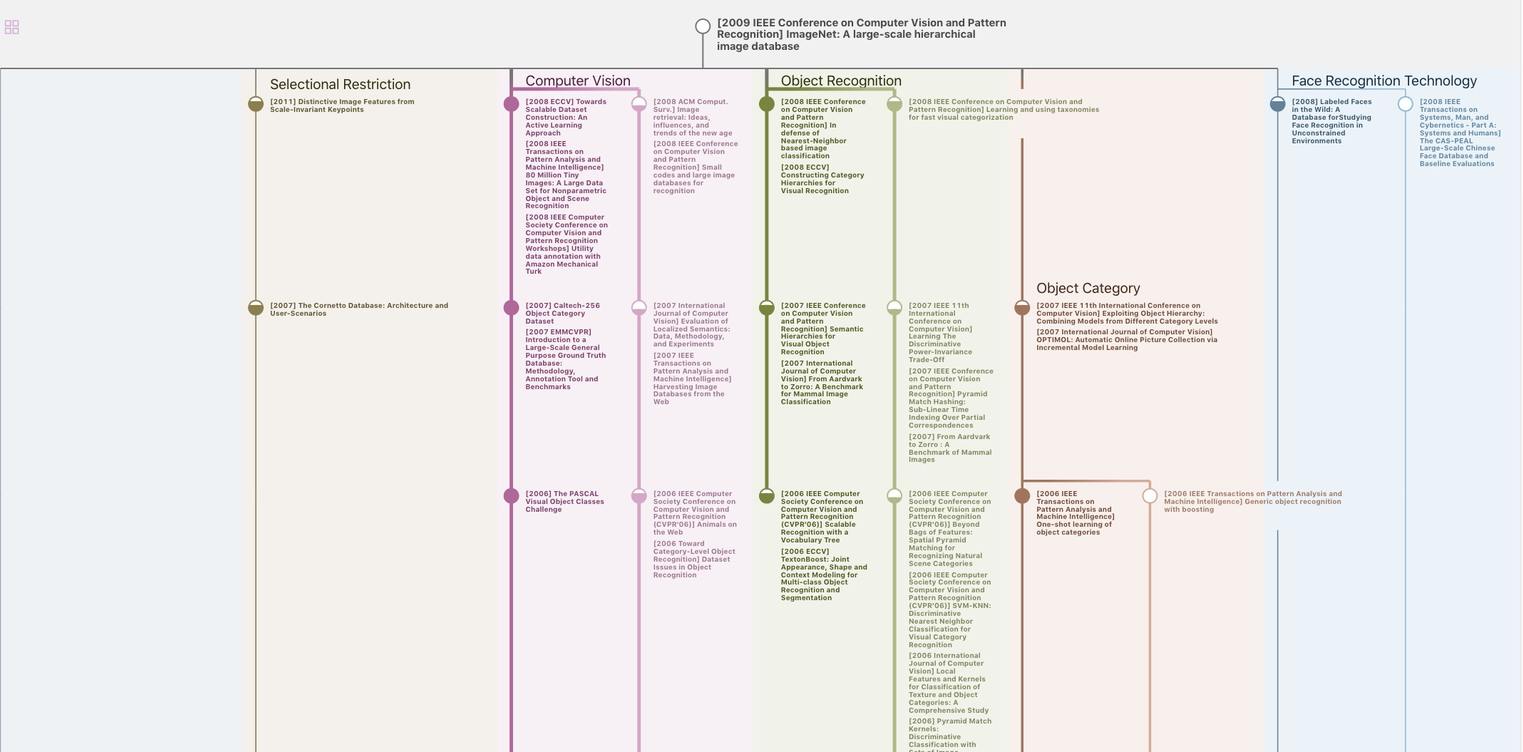
生成溯源树,研究论文发展脉络
Chat Paper
正在生成论文摘要