Regularized Bayesian best response learning in finite games
arxiv(2023)
摘要
We introduce the notion of regularized Bayesian best response (RBBR) learning dynamic in heterogeneous population games. We obtain such a dynamic via perturbation by an arbitrary lower semicontinuous, strongly convex regularizer in Bayesian population games with finitely many strategies. We provide a sufficient condition for the existence of rest points of the RBBR learning dynamic, and hence the existence of regularized Bayesian equilibrium in Bayesian population games. These equilibria are shown to approximate the original Bayesian equilibria for vanishingly small perturbations. We also explore the fundamental properties of the RBBR learning dynamic, which includes the existence of unique continuous solutions from arbitrary initial conditions, as well as the continuity of the solution trajectories thus obtained with respect to the initial conditions. Finally, as application the the theory we introduce the notions of Bayesian potential and Bayesian negative semidefinite games and provide convergence results for such games.
更多查看译文
关键词
bayesian best response,learning
AI 理解论文
溯源树
样例
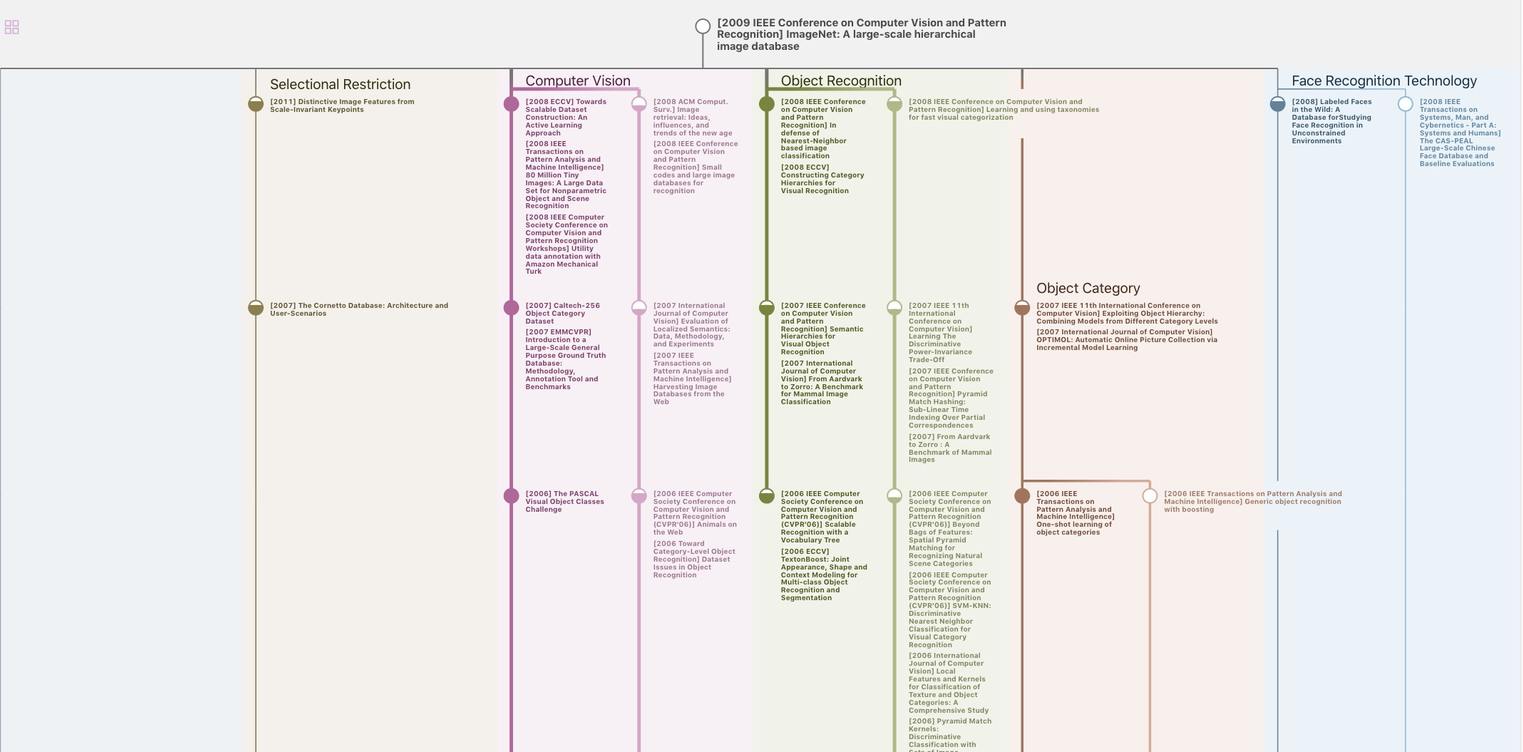
生成溯源树,研究论文发展脉络
Chat Paper
正在生成论文摘要