Self-Supervised Learning for Semi-Supervised Temporal Language Grounding
IEEE TRANSACTIONS ON MULTIMEDIA(2023)
摘要
Given a text description, Temporal Language Grounding (TLG) aims to localize temporal boundaries of the segments that contain the specified semantics in an untrimmed video. TLG is inherently a challenging task, as it requires comprehensive understanding of both sentence semantics and video contents. Previous works either tackle this task in a fully-supervised setting that requires a large amount of temporal annotations or in a weakly-supervised setting that usually cannot achieve satisfactory performance. Since manual annotations are expensive, to cope with limited annotations, we tackle TLG in a semi-supervised way by incorporating self-supervised learning, and propose Self-Supervised Semi-Supervised Temporal Language Grounding (S(4)TLG). S(4)TLG consists of two parts: (1) A pseudo label generation module that adaptively produces instant pseudo labels for unlabeled samples based on predictions from a teacher model; (2) A self-supervised feature learning module with intermodal and intra-modal contrastive losses to learn video feature representations under the constraints of video content consistency and video-text alignment. We conduct extensive experiments on the ActivityNet-CD-OOD and Charades-CD-OOD datasets. The results demonstrate that our proposed S(4)TLG can achieve competitive performance compared to fully-supervised state-of-the-art methods while only requiring a small portion of temporal annotations.
更多查看译文
关键词
Temporal language grounding,Semi-supervised learning,Contrastive learning
AI 理解论文
溯源树
样例
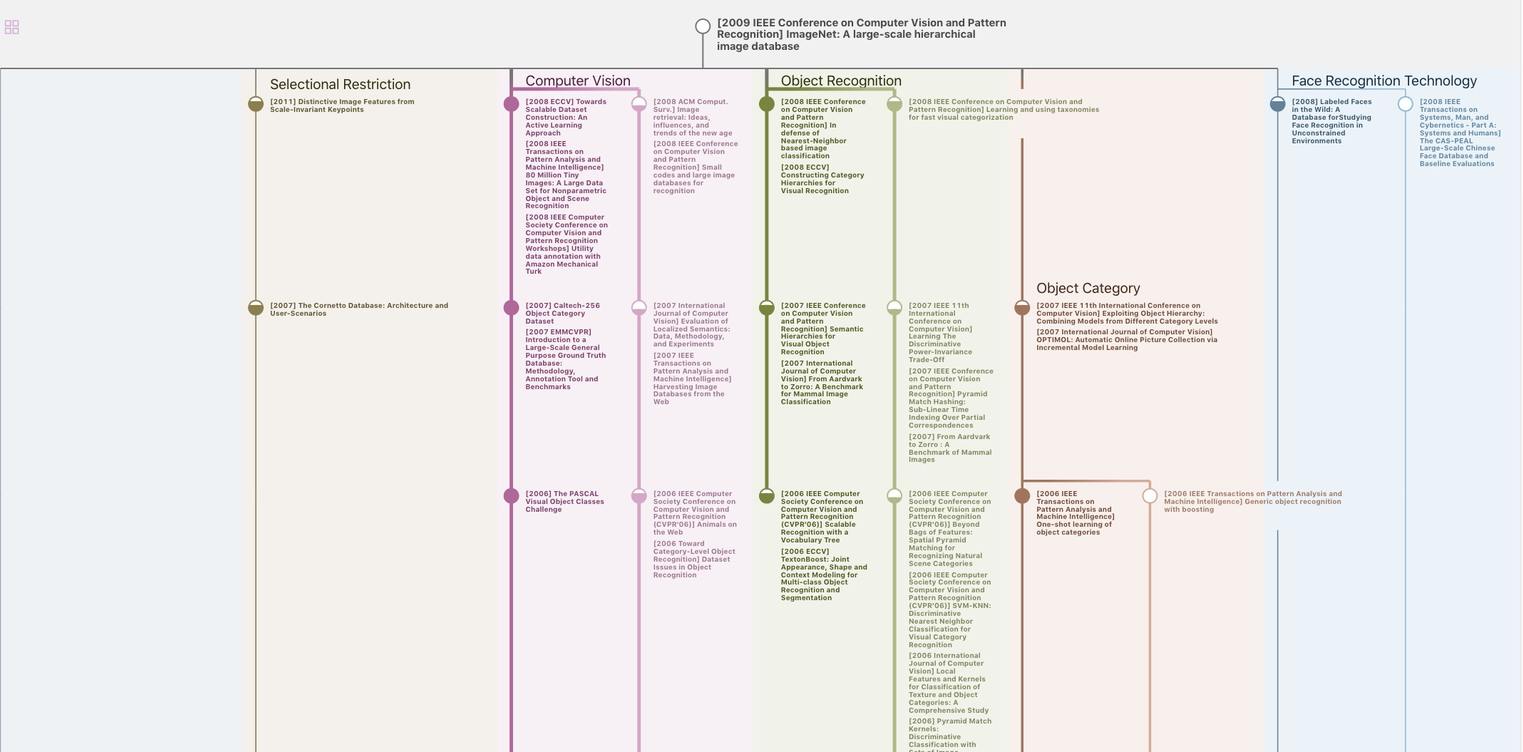
生成溯源树,研究论文发展脉络
Chat Paper
正在生成论文摘要