GeoNeRF: Generalizing NeRF with Geometry Priors
IEEE Conference on Computer Vision and Pattern Recognition(2022)
摘要
We present GeoNeRF, a generalizable photorealistic novel view synthesis method based on neural radiance fields. Our approach consists of two main stages: a ge-ometry reasoner and a renderer. To render a novel view, the geometry reasoner first constructs cascaded cost volumes for each nearby source view. Then, using a Transformer- based attention mechanism and the cascaded cost volumes, the renderer infers geometry and appearance, and ren-ders detailed images via classical volume rendering techniques. This architecture, in particular, allows sophis-ticated occlusion reasoning, gathering information from consistent source views. Moreover, our method can eas-ily be fine-tuned on a single scene, and renders com-petitive results with per-scene optimized neural rendering methods with a fraction of computational cost. Ex-periments show that GeoNeRF outperforms state-of-the- art generalizable neural rendering models on various syn-thetic and real datasets. Lastly, with a slight modification to the geometry reasoner, we also propose an alter-native model that adapts to RGBD images. This model di-rectly exploits the depth information often available thanks to depth sensors. The implementation code is available at https://www.idiap.ch/paper/geonerf.
更多查看译文
关键词
Image and video synthesis and generation, 3D from multi-view and sensors, RGBD sensors and analytics
AI 理解论文
溯源树
样例
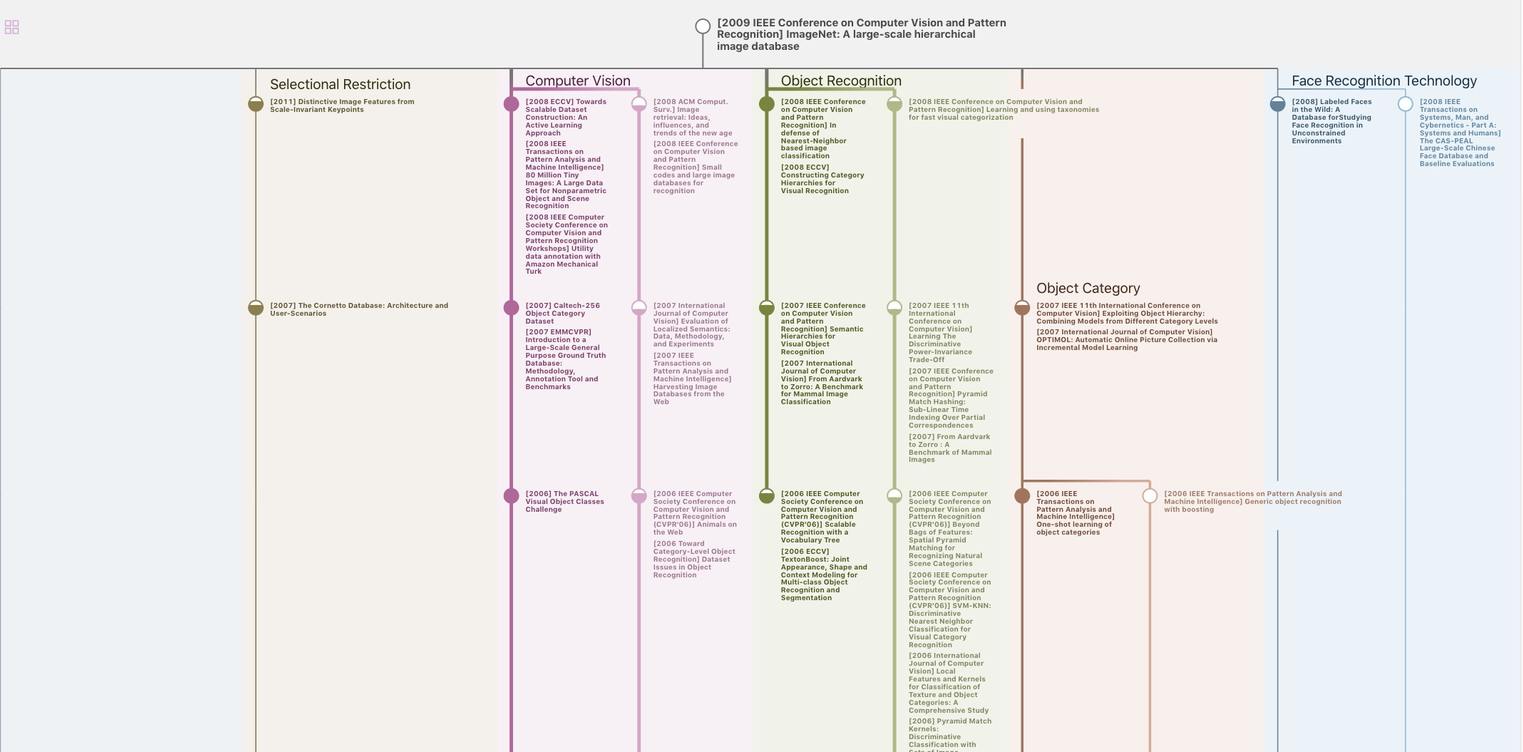
生成溯源树,研究论文发展脉络
Chat Paper
正在生成论文摘要