GPR1200: A Benchmark for General-Purpose Content-Based Image Retrieval
MULTIMEDIA MODELING (MMM 2022), PT I(2022)
摘要
Even though it has extensively been shown that retrieval specific training of deep neural networks is beneficial for nearest neighbor image search quality, most of these models are trained and tested in the domain of landmarks images. However, some applications use images from various other domains and therefore need a network with good generalization properties - a general-purpose CBIR model. To the best of our knowledge, no testing protocol has so far been introduced to benchmark models with respect to general image retrieval quality. After analyzing popular image retrieval test sets we decided to manually curate GPR1200, an easy to use and accessible but challenging benchmark dataset with a broad range of image categories. This benchmark is subsequently used to evaluate various pretrained models of different architectures on their generalization qualities. We show that large-scale pretraining significantly improves retrieval performance and present experiments on how to further increase these properties by appropriate fine-tuning. With these promising results, we hope to increase interest in the research topic of general-purpose CBIR.
更多查看译文
关键词
Content-based image retrieval, Image descriptors, Feature extraction, Generalization, Retrieval benchmark, Image datasets
AI 理解论文
溯源树
样例
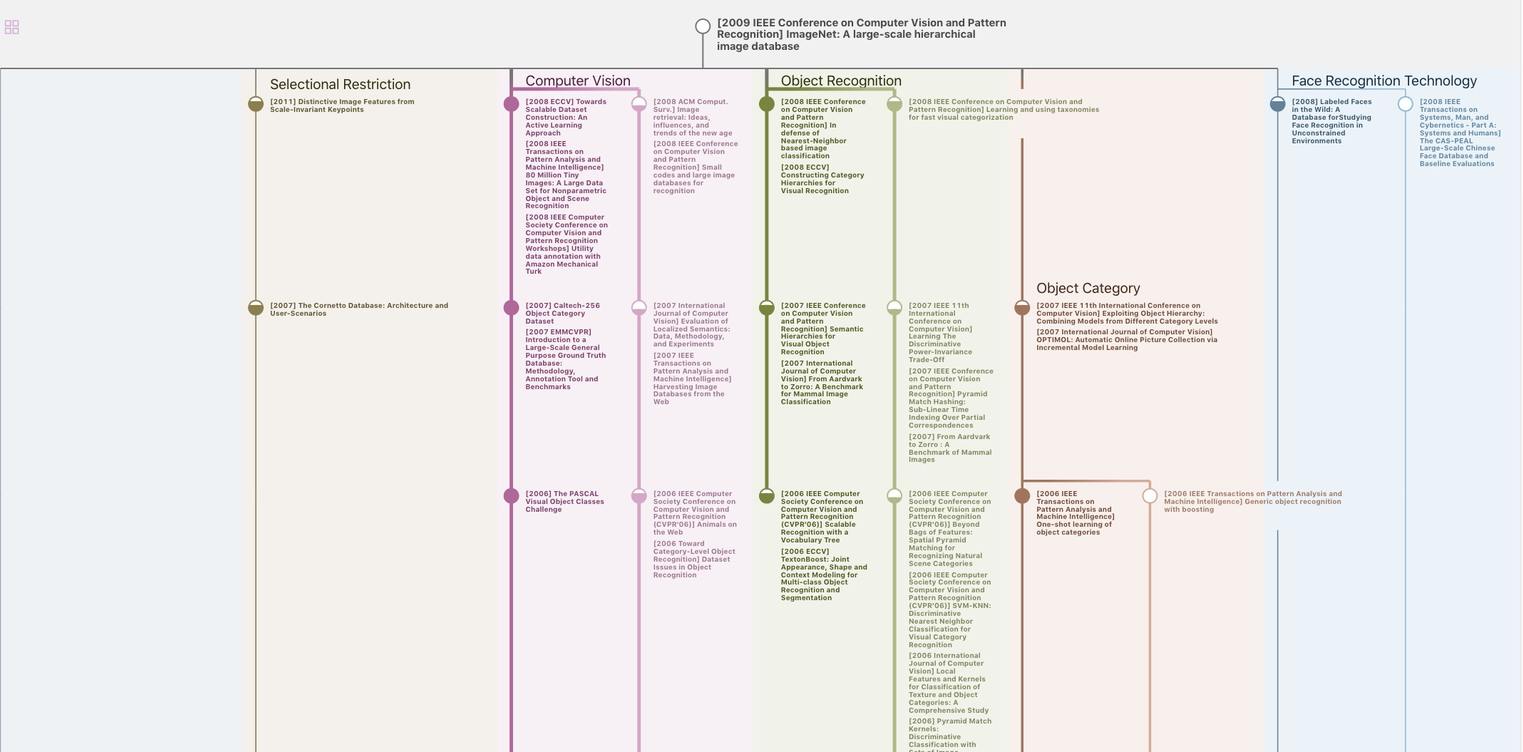
生成溯源树,研究论文发展脉络
Chat Paper
正在生成论文摘要