Combating Unknown Bias with Effective Bias-Conflicting Scoring and Gradient Alignment
arxiv(2022)
摘要
Models notoriously suffer from dataset biases which are detrimental to robustness and generalization. The identify-emphasize paradigm shows a promising effect in dealing with unknown biases. However, we find that it is still plagued by two challenges: A, the quality of the identified bias-conflicting samples is far from satisfactory; B, the emphasizing strategies just yield suboptimal performance. In this work, for challenge A, we propose an effective bias-conflicting scoring method to boost the identification accuracy with two practical strategies -- peer-picking and epoch-ensemble. For challenge B, we point out that the gradient contribution statistics can be a reliable indicator to inspect whether the optimization is dominated by bias-aligned samples. Then, we propose gradient alignment, which employs gradient statistics to balance the contributions of the mined bias-aligned and bias-conflicting samples dynamically throughout the learning process, forcing models to leverage intrinsic features to make fair decisions. Experiments are conducted on multiple datasets in various settings, demonstrating that the proposed solution can alleviate the impact of unknown biases and achieve state-of-the-art performance.
更多查看译文
关键词
unknown bias,gradient alignment,bias-conflicting
AI 理解论文
溯源树
样例
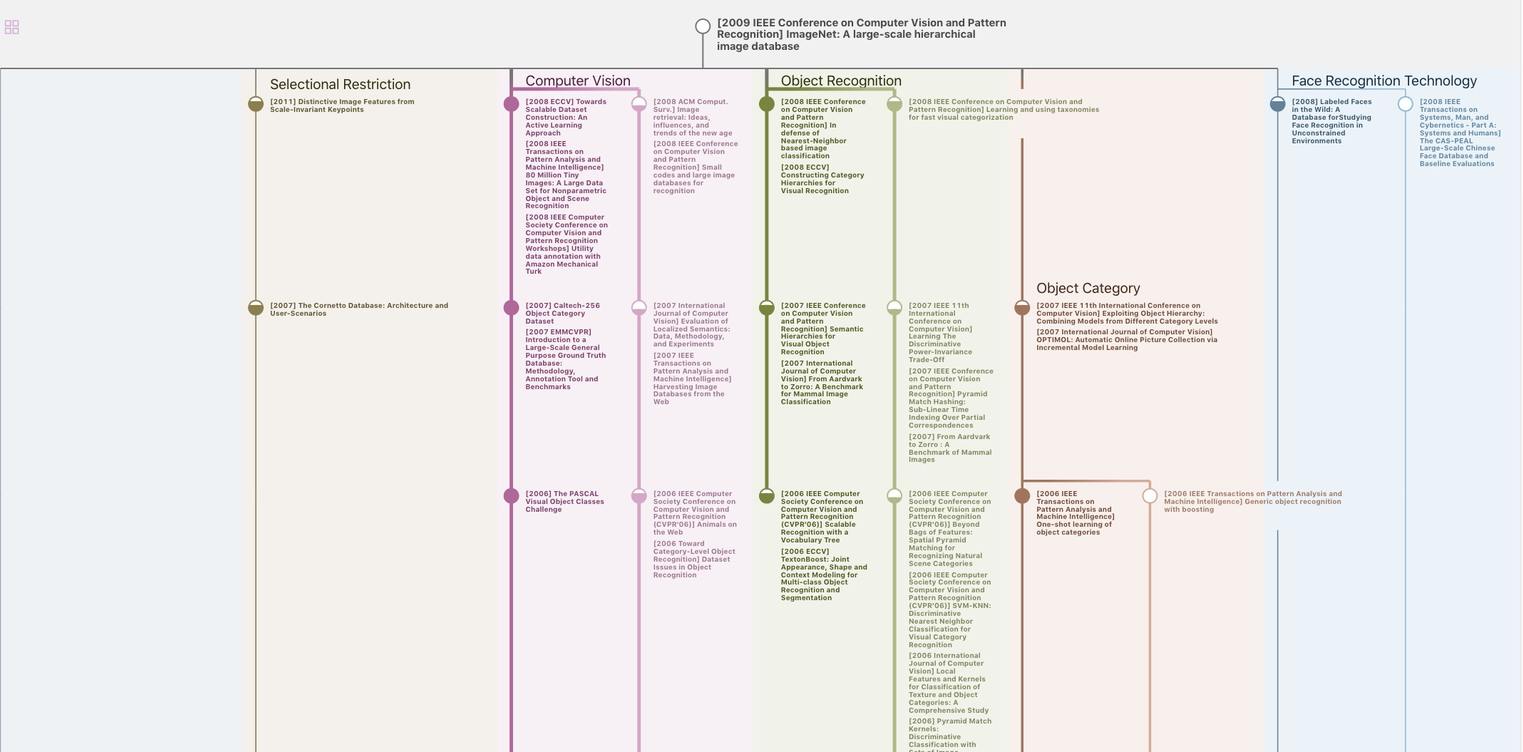
生成溯源树,研究论文发展脉络
Chat Paper
正在生成论文摘要