Fairness Measures for Machine Learning in Finance
user-5fe1a78c4c775e6ec07359f9(2021)
摘要
The authors present a machine learning pipeline for fairness-aware machine learning (FAML) in finance that encompasses metrics for fairness (and accuracy). Whereas accuracy metrics are well understood and the principal ones are used frequently, there is no consensus as to which of several available measures for fairness should be used in a generic manner in the financial services industry. The authors explore these measures and discuss which ones to focus on at various stages in the ML pipeline, pre-training and post-training, and they examine simple bias mitigation approaches. Using a standard dataset, they show that the sequencing in their FAML pipeline offers a cogent approach to arriving at a fair and accurate ML model. The authors discuss the intersection of bias metrics with legal considerations in the United States, and the entanglement of explainability and fairness is exemplified in the case study. They discuss possible approaches for training ML models while satisfying constraints imposed from various fairness metrics and the role of causality in assessing fairness. TOPICS:Big data/machine learning, legal/regulatory/public policy, performance measurement Key Findings ▪ Sources of bias are presented and a range of metrics is considered for machine learning applications in finance, both pre-training and post-training of models. ▪ A process of using the metrics to arrive at fair models is discussed. ▪ Various considerations for the choice of specific metrics are also analyzed.
更多查看译文
关键词
Fairness measure,Performance measurement,Big data,Pipeline (software),Principal (computer security),Process (engineering),Computer science,Machine learning,Intersection (set theory),Financial services,Finance,Artificial intelligence
AI 理解论文
溯源树
样例
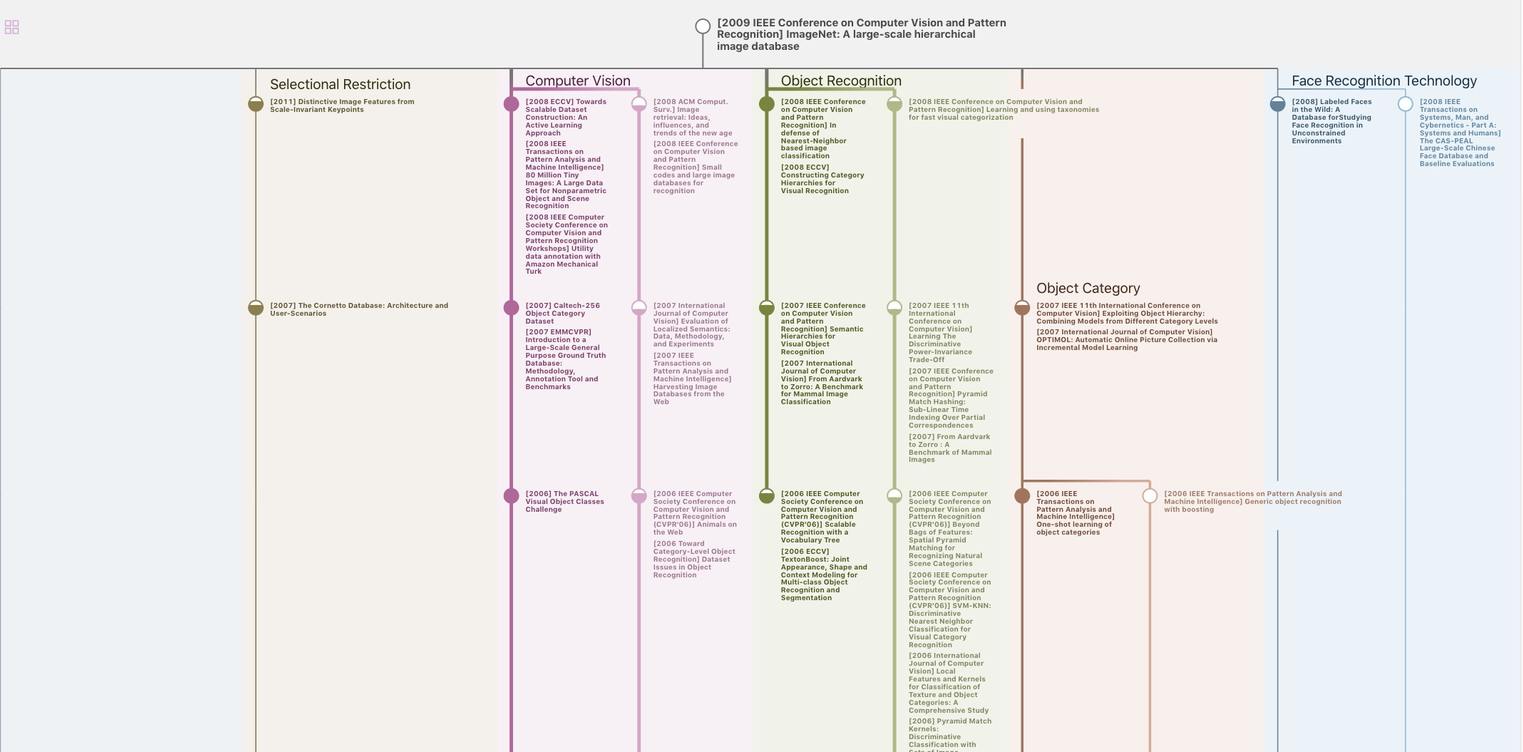
生成溯源树,研究论文发展脉络
Chat Paper
正在生成论文摘要