Joint Feature-Space and Sample-Space Based Heterogeneous Feature Transfer Method for Object Recognition Using Remote Sensing Images with Different Spatial Resolutions
SENSORS(2021)
摘要
To improve the classification results of high-resolution remote sensing images (RSIs), it is necessary to use feature transfer methods to mine the relevant information between high-resolution RSIs and low-resolution RSIs to train the classifiers together. Most of the existing feature transfer methods can only handle homogeneous data (i.e., data with the same dimension) and are susceptible to the quality of the RSIs, while RSIs with different resolutions present different feature dimensions and samples obtained from illumination conditions. To obtain effective classification results, unlike existing methods that focus only on the projection transformation in feature space, a joint feature-space and sample-space heterogeneous feature transfer (JFSSS-HFT) method is proposed to simultaneously process heterogeneous multi-resolution images in feature space using projection matrices of different dimensions and reduce the impact of outliers by adaptive weight factors in the sample space simultaneously to reduce the occurrence of negative transfer. Moreover, the maximum interclass variance term is embedded to improve the discriminant ability of the transferred features. To solve the optimization problem of JFSSS-HFT, the alternating-direction method of multipliers (ADMM) is introduced to alternatively optimize the parameters of JFSSS-HFT. Using different types of ship patches and airplane patches with different resolutions, the experimental results show that the proposed JFSSS-HFT obtains better classification results than the typical feature transferred methods.
更多查看译文
关键词
heterogeneous feature transfer, classification of remote sensing images, transfer learning, negative transfer
AI 理解论文
溯源树
样例
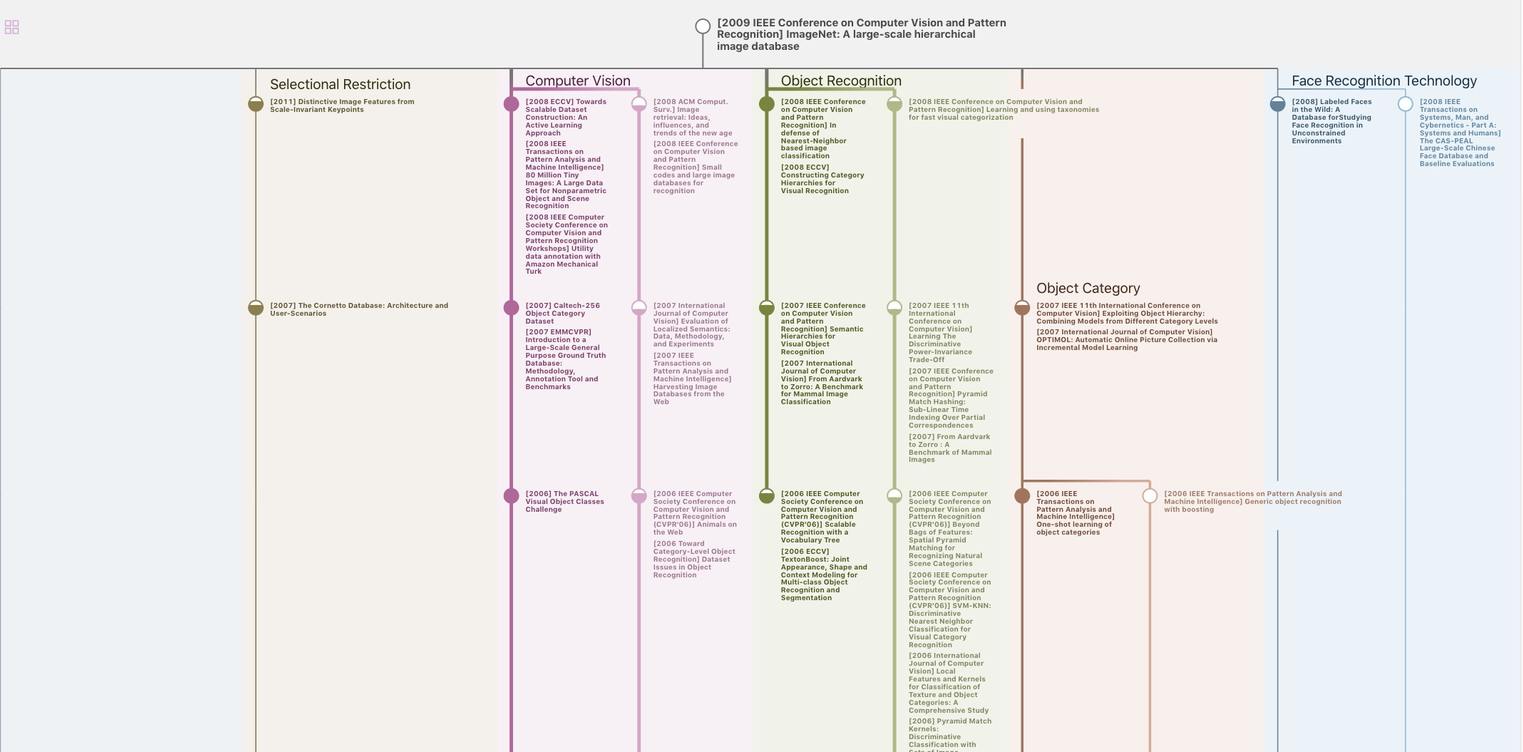
生成溯源树,研究论文发展脉络
Chat Paper
正在生成论文摘要