Implementing In Vitro Bioactivity Data to Modernize Priority Setting of Chemical Inventories
ALTEX-ALTERNATIVES TO ANIMAL EXPERIMENTATION(2022)
摘要
Internationally, there are thousands of existing and newly introduced chemicals in commerce, highlighting the ongoing importance of innovative approaches to identify emerging chemicals of concern. For many chemicals, there is a paucity of hazard and exposure data. Thus, there is a crucial need for efficient and robust approaches to address data gaps and support risk-based prioritization. Several studies have demonstrated the utility of in vitro bioactivity data from the ToxCast program in deriving points of departure (PODs). ToxCast contains data for nearly 1,400 endpoints per chemical, and the bioactivity concentrations, indicative of potential adverse outcomes, can be converted to human-equivalent PODs using high-throughput toxicokinetics (HTTK) modeling. However, data gaps need to be addressed for broader application: the limited chemical space of HTTK and quantitative high-throughput screening data. Here we explore the applicability of in silico models to address these data needs. Specifically, we used ADMET predictor for HTTK predictions and a generalized read-across approach to predict ToxCast bioactivity potency. We applied these models to profile 5,801 chemicals on Canada's Domestic Substances List (DSL). To evaluate the approach's performance, bioactivity PODs were compared with in vivo results from the EPA Toxicity Values database for 1,042 DSL chemicals. Comparisons demonstrated that the bioactivity PODs, based on ToxCast data or read-across, were conservative for 95% of the chemicals. Comparing bioactivity PODs to human exposure estimates supports the identification of chemicals of potential interest for further work. The bioactivity workflow shows promise as a powerful screening tool to support effective triaging of chemical inventories.
更多查看译文
关键词
evidence-based risk assessment,high-throughput screening,high-throughput toxicokinetics,new approach methodologies,read-across
AI 理解论文
溯源树
样例
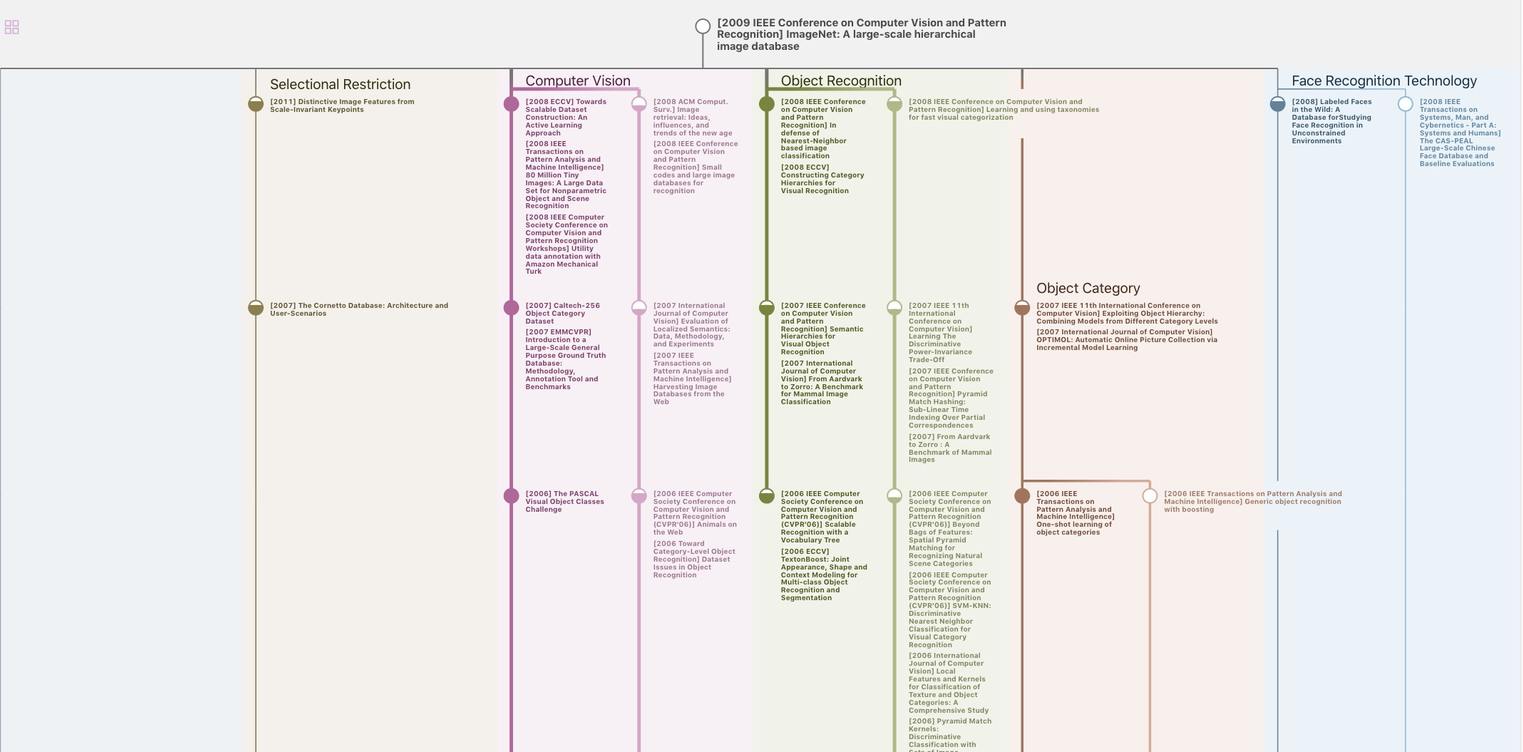
生成溯源树,研究论文发展脉络
Chat Paper
正在生成论文摘要