Regularised orthogonal machine learning for nonlinear semiparametric models
ECONOMETRICS JOURNAL(2022)
摘要
This paper proposes a Lasso-type estimator for a high-dimensional sparse parameter identified by a single index conditional moment restriction (CMR). In addition to this parameter, the moment function can also depend on a nuisance function, such as the propensity score or the conditional choice probability, which we estimate by modern machine learning tools. We first adjust the moment function so that the gradient of the future loss function is insensitive (formally, Neyman orthogonal) with respect to the first-stage regularisation bias, preserving the single index property. We then take the loss function to be an indefinite integral of the adjusted moment function with respect to the single index. The proposed Lasso estimator converges at the oracle rate, where the oracle knows the nuisance function and solves only the parametric problem. We demonstrate our method by estimating the short-term heterogeneous impact of Connecticut's Jobs First welfare reform experiment on women's welfare participation decision.
更多查看译文
关键词
Conditional moment restrictions, high-dimensional sparse estimation, machine learning, M-estimation, Neyman orthogonality, single index models
AI 理解论文
溯源树
样例
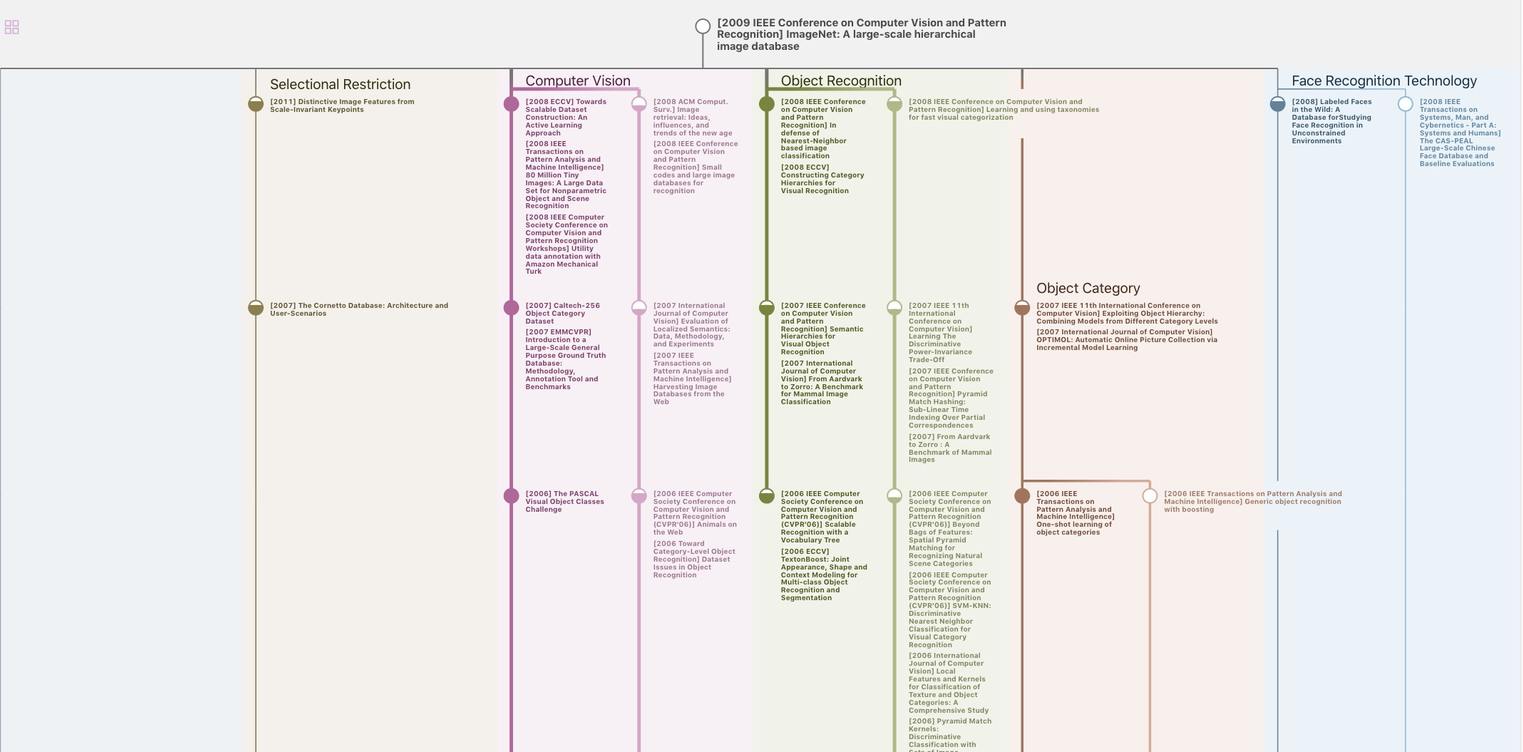
生成溯源树,研究论文发展脉络
Chat Paper
正在生成论文摘要