Selection of Pseudo-Annotated Data for Adverse Drug Reaction Classification Across Drug Groups.
International Joint Conference on the Analysis of Images, Social Networks and Texts (AIST)(2021)
摘要
Automatic monitoring of adverse drug events (ADEs) or reactions (ADRs) is currently receiving significant attention from the biomedical community. In recent years, user-generated data on social media has become a valuable resource for this task. Neural models have achieved impressive performance on automatic text classification for ADR detection. Yet, training and evaluation of these methods are carried out on user-generated texts about a targeted drug. In this paper, we assess the robustness of state-of-the-art neural architectures across different drug groups. We investigate several strategies to use pseudo-labeled data in addition to a manually annotated train set. Out-of-dataset experiments diagnose the bottleneck of supervised models in terms of breakdown performance, while additional pseudo-labeled data improves overall results regardless of the text selection strategy.
更多查看译文
关键词
adverse drug reaction
AI 理解论文
溯源树
样例
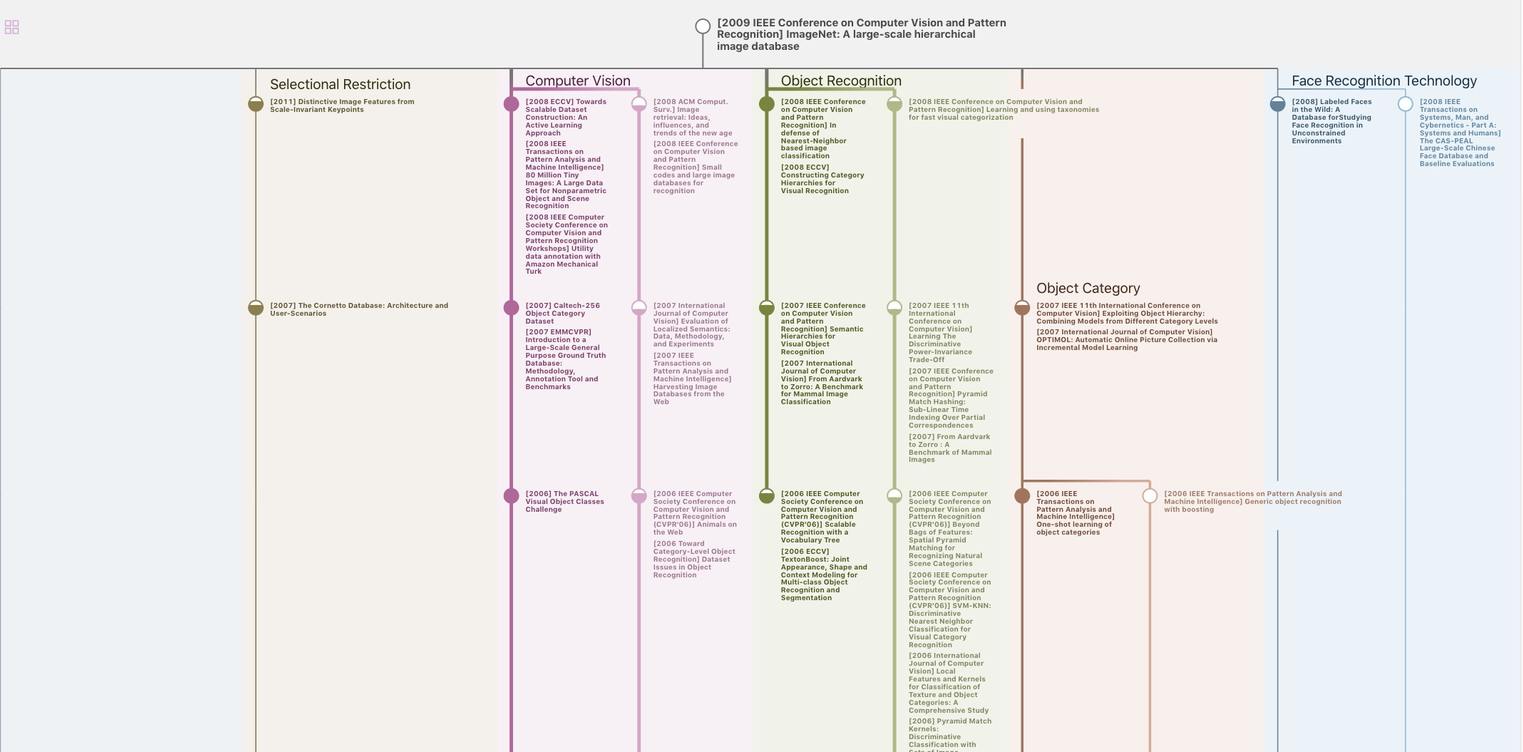
生成溯源树,研究论文发展脉络
Chat Paper
正在生成论文摘要