Optimizing the human learnability of abstract network representations.
Proceedings of the National Academy of Sciences of the United States of America(2022)
摘要
Precisely how humans process relational patterns of information in knowledge, language, music, and society is not well understood. Prior work in the field of statistical learning has demonstrated that humans process such information by building internal models of the underlying network structure. However, these mental maps are often inaccurate due to limitations in human information processing. The existence of such limitations raises clear questions: Given a target network that one wishes for a human to learn, what network should one present to the human? Should one simply present the target network as-is, or should one emphasize certain parts of the network to proactively mitigate expected errors in learning? To investigate these questions, we study the optimization of network learnability in a computational model of human learning. Evaluating an array of synthetic and real-world networks, we find that learnability is enhanced by reinforcing connections within modules or clusters. In contrast, when networks contain significant core-periphery structure, we find that learnability is best optimized by reinforcing peripheral edges between low-degree nodes. Overall, our findings suggest that the accuracy of human network learning can be systematically enhanced by targeted emphasis and de-emphasis of prescribed sectors of information.
更多查看译文
关键词
complex networks,graph learning,maximum entropy
AI 理解论文
溯源树
样例
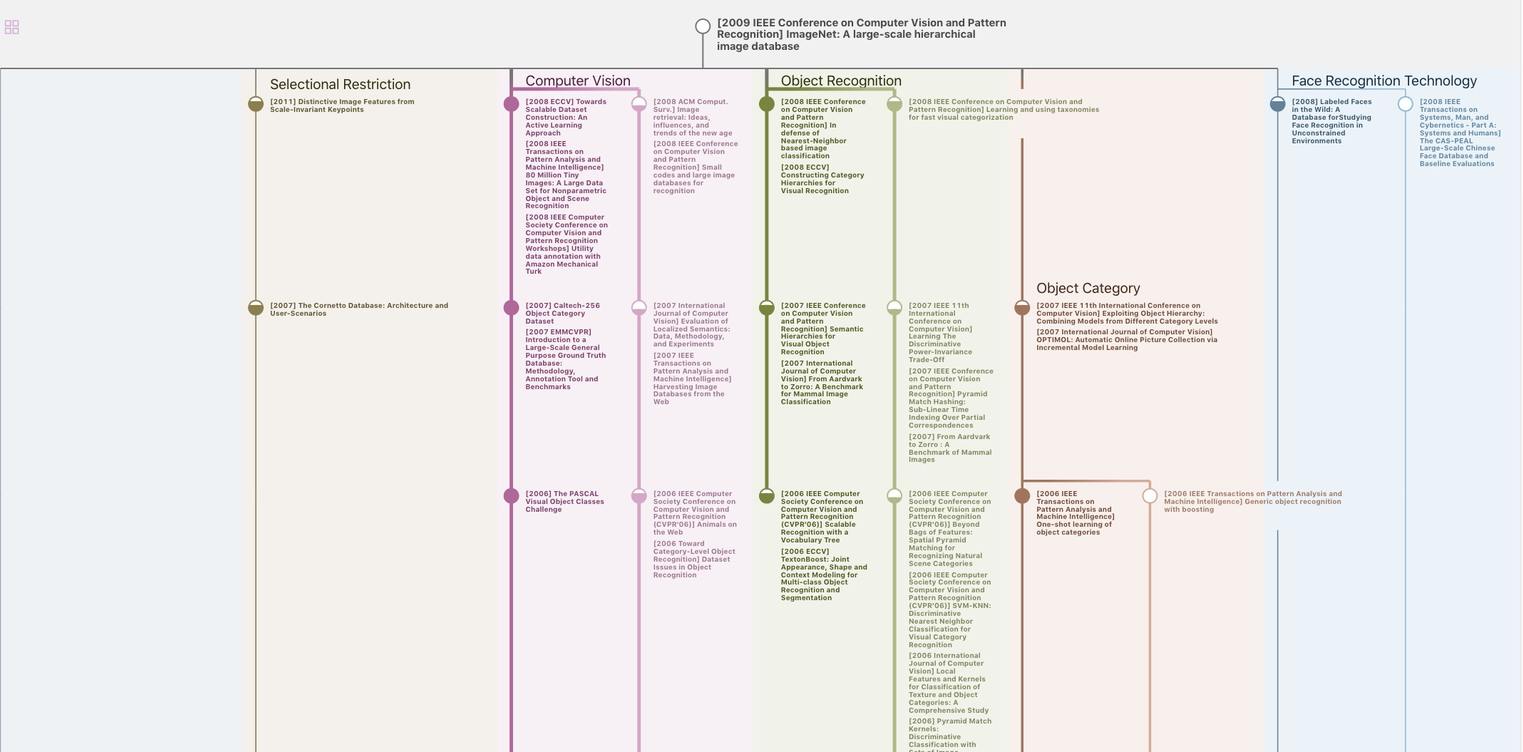
生成溯源树,研究论文发展脉络
Chat Paper
正在生成论文摘要