Binned multinomial logistic regression for integrative cell-type annotation
ANNALS OF APPLIED STATISTICS(2023)
摘要
Categorizing individual cells into one of many known cell-type cate-gories, also known as cell-type annotation, is a critical step in the analy-sis of single-cell genomics data. The current process of annotation is time intensive and subjective, which has led to different studies describing cell types with labels of varying degrees of resolution. While supervised learn-ing approaches have provided automated solutions to annotation, there re-mains a significant challenge in fitting a unified model for multiple datasets with inconsistent labels. In this article we propose a new multinomial lo-gistic regression estimator which can be used to model cell-type probabil-ities by integrating multiple datasets with labels of varying resolution. To compute our estimator, we solve a nonconvex optimization problem using a blockwise proximal gradient descent algorithm. We show through simu-lation studies that our approach estimates cell-type probabilities more accu-rately than competitors in a wide variety of scenarios. We apply our method to 10 single-cell RNA-seq datasets and demonstrate its utility in predicting fine resolution cell-type labels on unlabeled data as well as refining cell -type labels on data with existing coarse resolution annotations. Finally, we demonstrate that our method can lead to novel scientific insights in the con-text of a differential expression analysis comparing peripheral blood gene expression before and after treatment with interferon-a. An R package im-plementing the method is available in the Supplementary Material and at https://github.com/keshav-motwani/IBMR, and the collection of datasets we analyze is available at https://github.com/keshav-motwani/AnnotatedPBMC.
更多查看译文
关键词
Integrative analysis,multinomial logistic regression,group lasso,nonconvex opti-mization,single-cell genomics,cell-type annotation
AI 理解论文
溯源树
样例
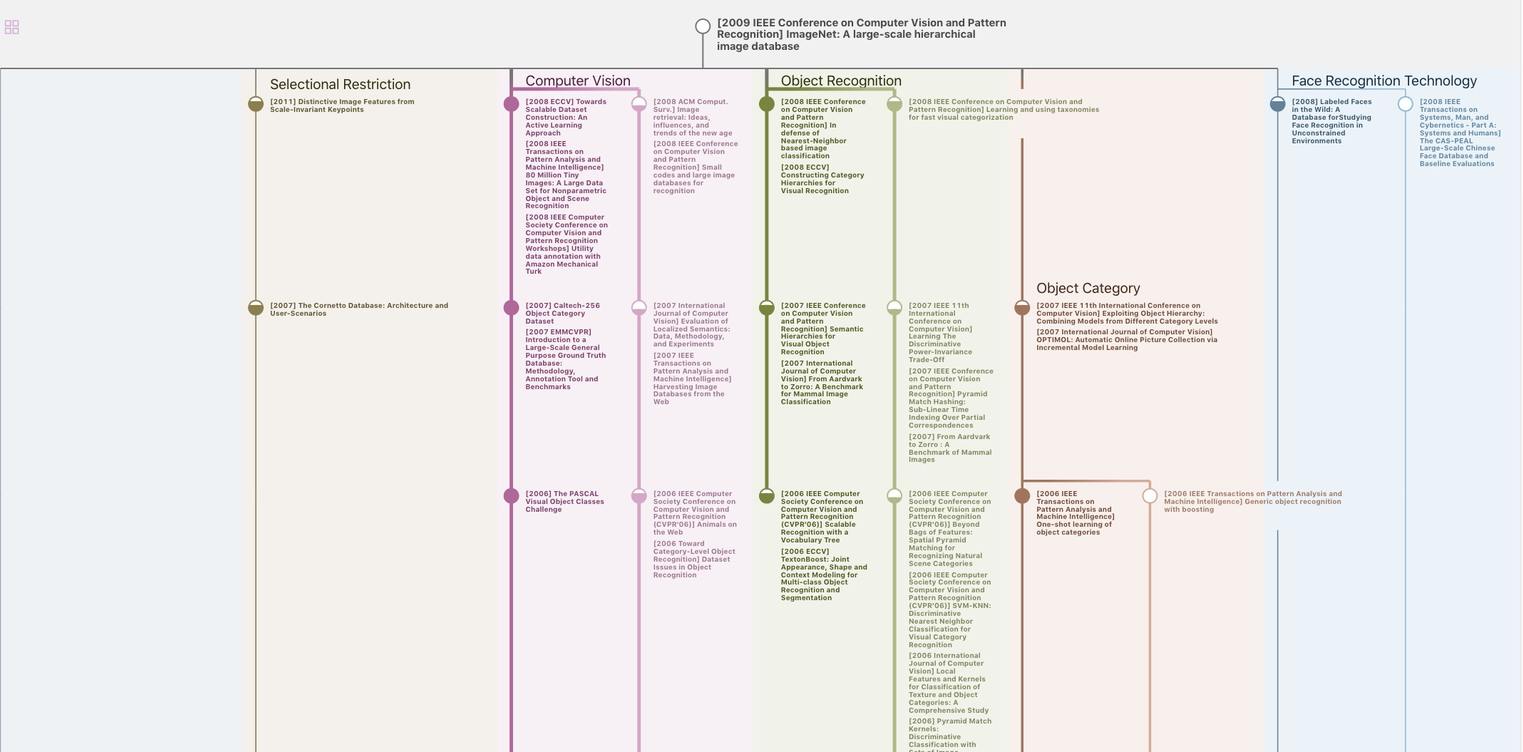
生成溯源树,研究论文发展脉络
Chat Paper
正在生成论文摘要