Calcium Scoring at Coronary CT Angiography Using Deep Learning
RADIOLOGY(2022)
摘要
Background: Separate noncontrast CT to quantify the coronary artery calcium (CAC) score often precedes coronary CT angiography (CTA). Quantifying CAC scores directly at CTA would eliminate the additional radiation produced at CT but remains challenging. Purpose: To quantify CAC scores automatically from a single CTA scan. Materials and Methods: In this retrospective study, a deep learning method to quantify CAC scores automatically from a single CTA scan was developed on training and validation sets of 292 patients and 73 patients collected from March 2019 to July 2020. Virtual noncontrast scans obtained with a spectral CT scanner were used to develop the algorithm to alleviate tedious manual annotation of calcium regions. The proposed method was validated on an independent test set of 240 CTA scans collected from three different CT scanners from August 2020 to November 2020 using the Pearson correlation coefficient, the coefficient of determination, or r(2), and the Bland-Altman plot against the semiautomatic Agatston score at noncontrast CT. The cardiovascular risk categorization performance was evaluated using weighted kappa based on the Agatston score (CAC score risk categories: 0-10, 11-100, 101-400, and >400). Results: Two hundred forty patients (mean age, 60 years 6 11 [standard deviation]; 146 men) were evaluated. The positive correlation between the automatic deep learning CTA and semiautomatic noncontrast CT CAC score was excellent (Pearson correlation = 0.96; r(2) = 0.92). The risk categorization agreement based on deep learning CTA and noncontrast CT CAC scores was excellent (weighted k = 0.94 [95% CI: 0.91, 0.97]), with 223 of 240 scans (93%) categorized correctly. All patients who were miscategorized were in the direct neighboring risk groups. The proposed method's differences from the noncontrast CT CAC score were not statistically significant with regard to scanner (P = .15), sex (P = .051), and section thickness (P = .67). Conclusion: A deep learning automatic calcium scoring method accurately quantified coronary artery calcium from CT angiography images and categorized risk. (c) RSNA, 2021
更多查看译文
AI 理解论文
溯源树
样例
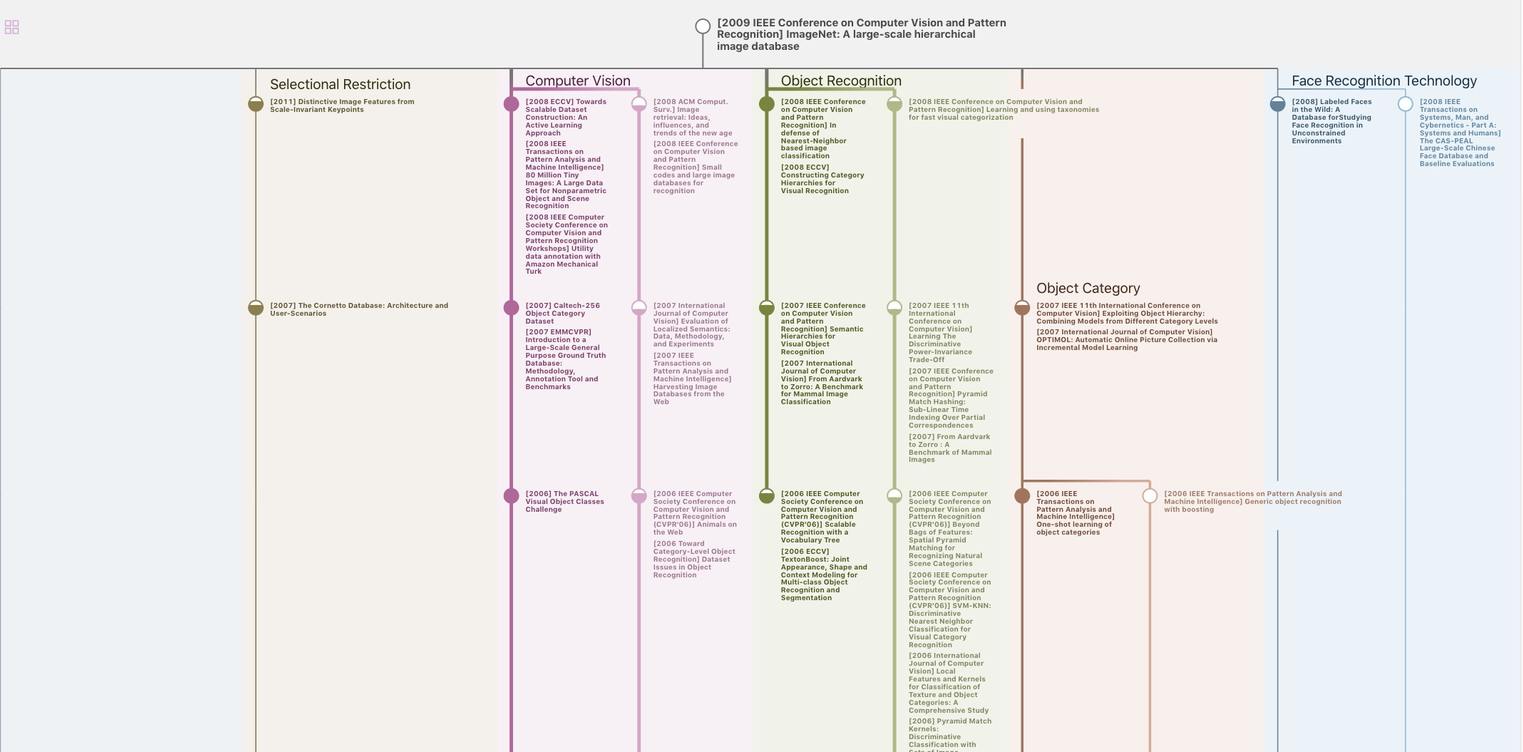
生成溯源树,研究论文发展脉络
Chat Paper
正在生成论文摘要