Euclidean Distance Approximations From Replacement Product Graphs
IEEE Transactions on Image Processing(2022)
摘要
We introduce a new chamfering paradigm, locally connecting pixels to produce path distances that approximate Euclidean space by building a small network (a replacement product) inside each pixel. These “
$RE$
-grid graphs” maintain near-Euclidean polygonal distance contours even in noisy data sets, making them useful tools for approximation when exact numerical solutions are unobtainable or impractical. The
$RE$
-grid graph creates a modular global architecture with lower pixel-to-pixel valency and simplified topology at the cost of increased computational complexity due to its internal structure. We present an introduction to chamfering replacement products with a number of case study examples to demonstrate the potential of these graphs for path-finding in high frequency and low resolution image spaces which motivate further study. Possible future applications include morphology, watershed segmentation, halftoning, neural network design, anisotropic image processing, image skeletonization, dendritic shaping, and cellular automata.
更多查看译文
关键词
Chamfer,euclidean distance,distance approximation,replacement product,graph theory,path-finding,eikonal equation,Dijkstra’s algorithm
AI 理解论文
溯源树
样例
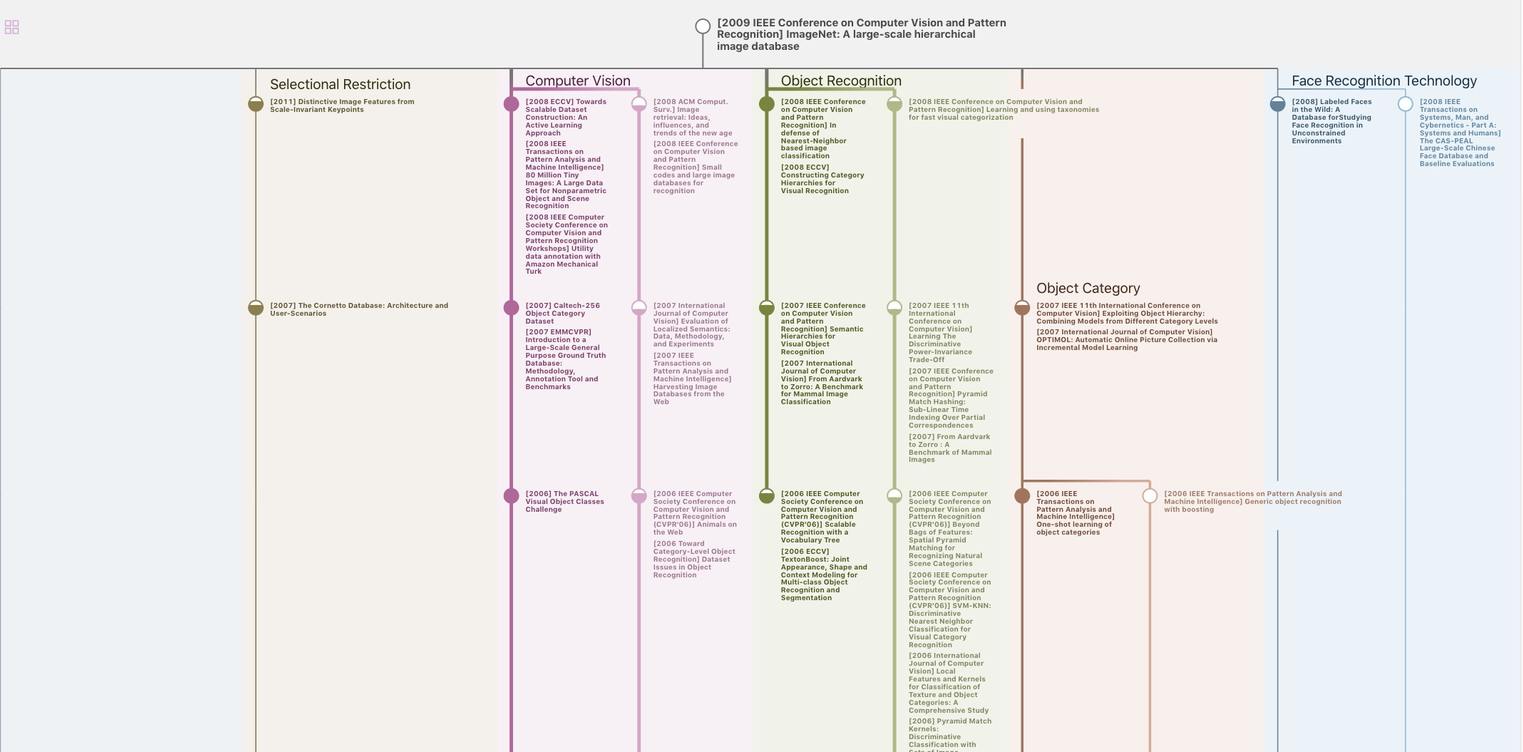
生成溯源树,研究论文发展脉络
Chat Paper
正在生成论文摘要