Leveraging Selective Prediction for Reliable Image Geolocation
MULTIMEDIA MODELING, MMM 2022, PT II(2022)
摘要
Reliable image geolocation is crucial for several applications, ranging from social media geo-tagging to media verification. State-of-the-art geolocation methods surpass human performance on the task of geolocation estimation from images. However, no method assesses the suitability of an image for this task, which results in unreliable and erroneous estimations for images containing ambiguous or no geolocation clues. In this paper, we define the task of image localizability, i.e. suitability of an image for geolocation, and propose a selective prediction methodology to address the task. In particular, we propose two novel selection functions that leverage the output probability distributions of geolocation models to infer localizability at different scales. Our selection functions are benchmarked against the most widely used selective prediction baselines, outperforming them in all cases. By abstaining from predicting non-localizable images, we improve geolocation accuracy from 27.8% to 70.5% at the city-scale, and thus make current geolocation models reliable for real-world applications.
更多查看译文
关键词
Image Localizability, Selective Prediction, Geolocation Estimation, Spatial Entropy, Prediction Density
AI 理解论文
溯源树
样例
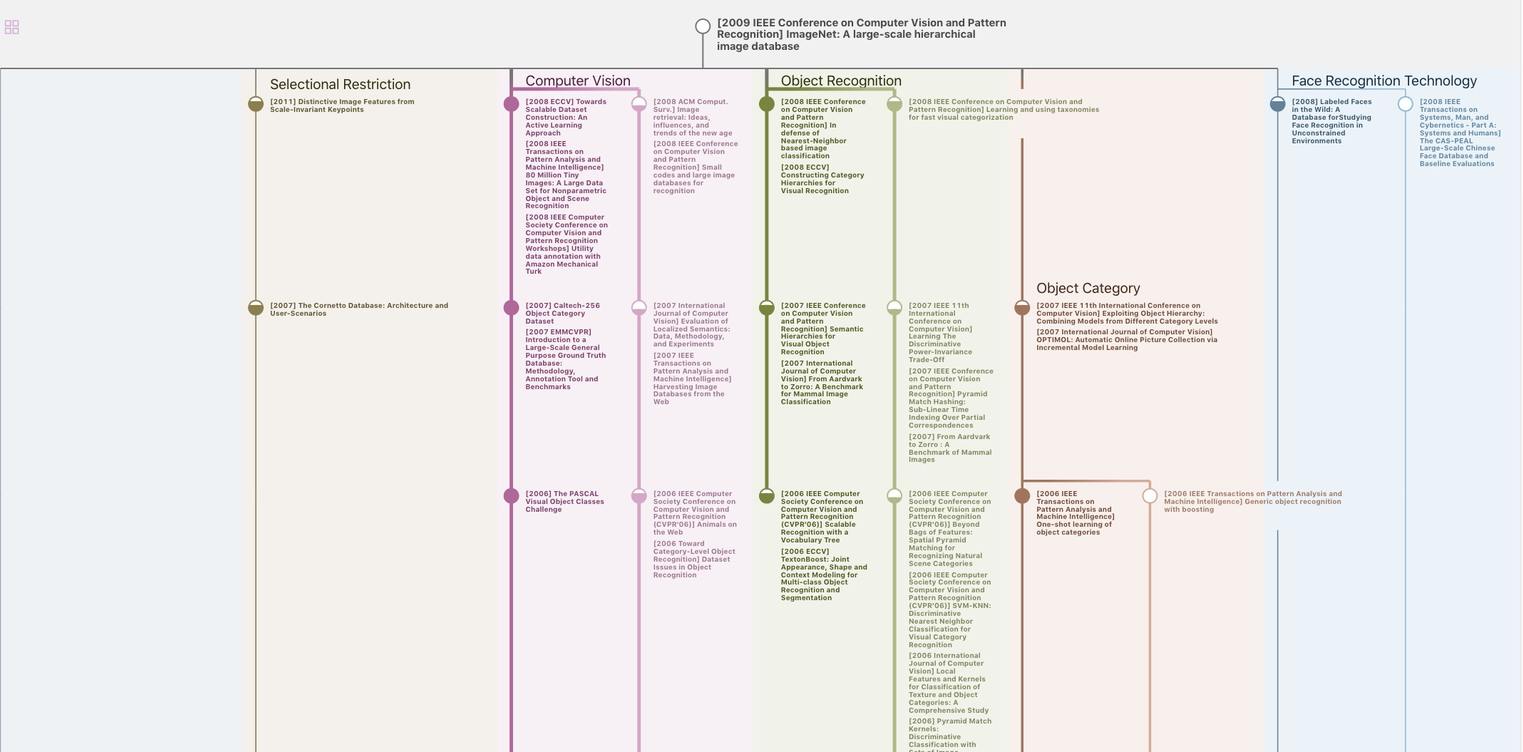
生成溯源树,研究论文发展脉络
Chat Paper
正在生成论文摘要