Semi-Supervision in ASR - Sequential MixMatch and Factorized TTS-Based Augmentation.
Interspeech(2021)
摘要
Semi and self-supervised training techniques have the potential to improve performance of speech recognition systems without additional transcribed speech data. In this work, we demonstrate the efficacy of two approaches to semi-supervision for automated speech recognition. The two approaches leverage vast amounts of available unspoken text and untranscribed audio. First, we present factorized multilingual speech synthesis to improve data augmentation on unspoken text. Next, we propose the Sequential MixMatch algorithm with iterative learning to learn from untranscribed speech. The algorithm is built on top of our online implementation of Noisy Student Training. We demonstrate the compatibility of these techniques yielding an overall relative reduction of word error rate of up to 14.4% on the voice search tasks on 4 Indic languages.
更多查看译文
关键词
Speech recognition,Computer science
AI 理解论文
溯源树
样例
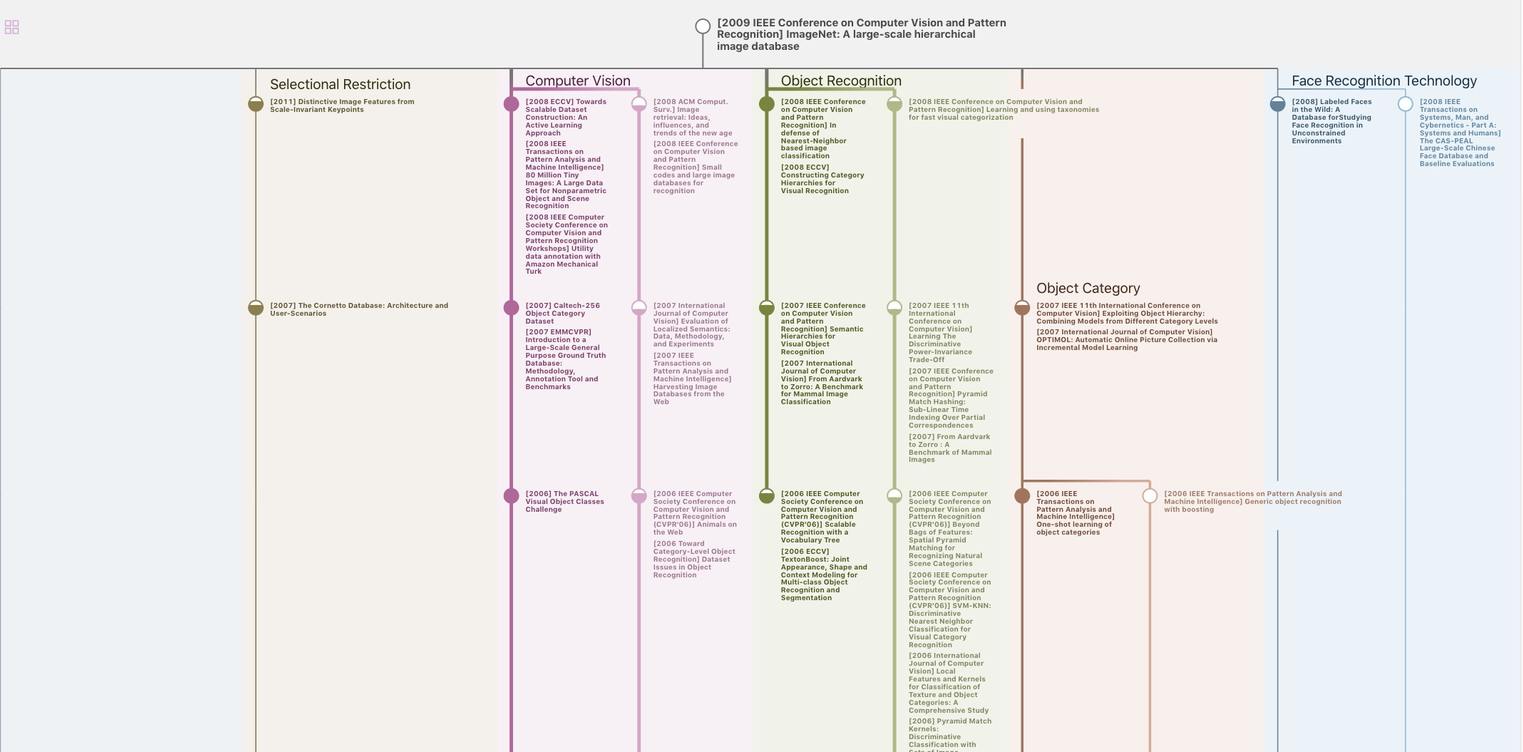
生成溯源树,研究论文发展脉络
Chat Paper
正在生成论文摘要