Forecasting the COVID-19 Pandemic: Lessons learned and future directions.
medRxiv : the preprint server for health sciences(2021)
摘要
I.The Coronavirus Disease 2019 (COVID-19) has demonstrated that accurate forecasts of infection and mortality rates are essential for informing healthcare resource allocation, designing countermeasures, implementing public health policies, and increasing public awareness. However, there exist a multitude of modeling methodologies, and their relative performances in accurately forecasting pandemic dynamics are not currently comprehensively understood. In this paper, we introduce the non-mechanistic MIT-LCP forecasting model, and assess and compare its performance to various mechanistic and non-mechanistic models that have been proposed for forecasting COVID-19 dynamics. We performed a comprehensive experimental evaluation which covered the time period of November 2020 to April 2021, in order to determine the relative performances of MIT-LCP and seven other forecasting models from the United States' Centers for Disease Control and Prevention (CDC) Forecast Hub. Our results show that there exist forecasting scenarios well-suited to both mechanistic and non-mechanistic models, with mechanistic models being particularly performant for forecasts that are further in the future when recent data may not be as informative, and non-mechanistic models being more effective with shorter prediction horizons when recent representative data is available. Improving our understanding of which forecasting approaches are more reliable, and in which forecasting scenarios, can assist effective pandemic preparation and management.
更多查看译文
关键词
pandemic,future directions
AI 理解论文
溯源树
样例
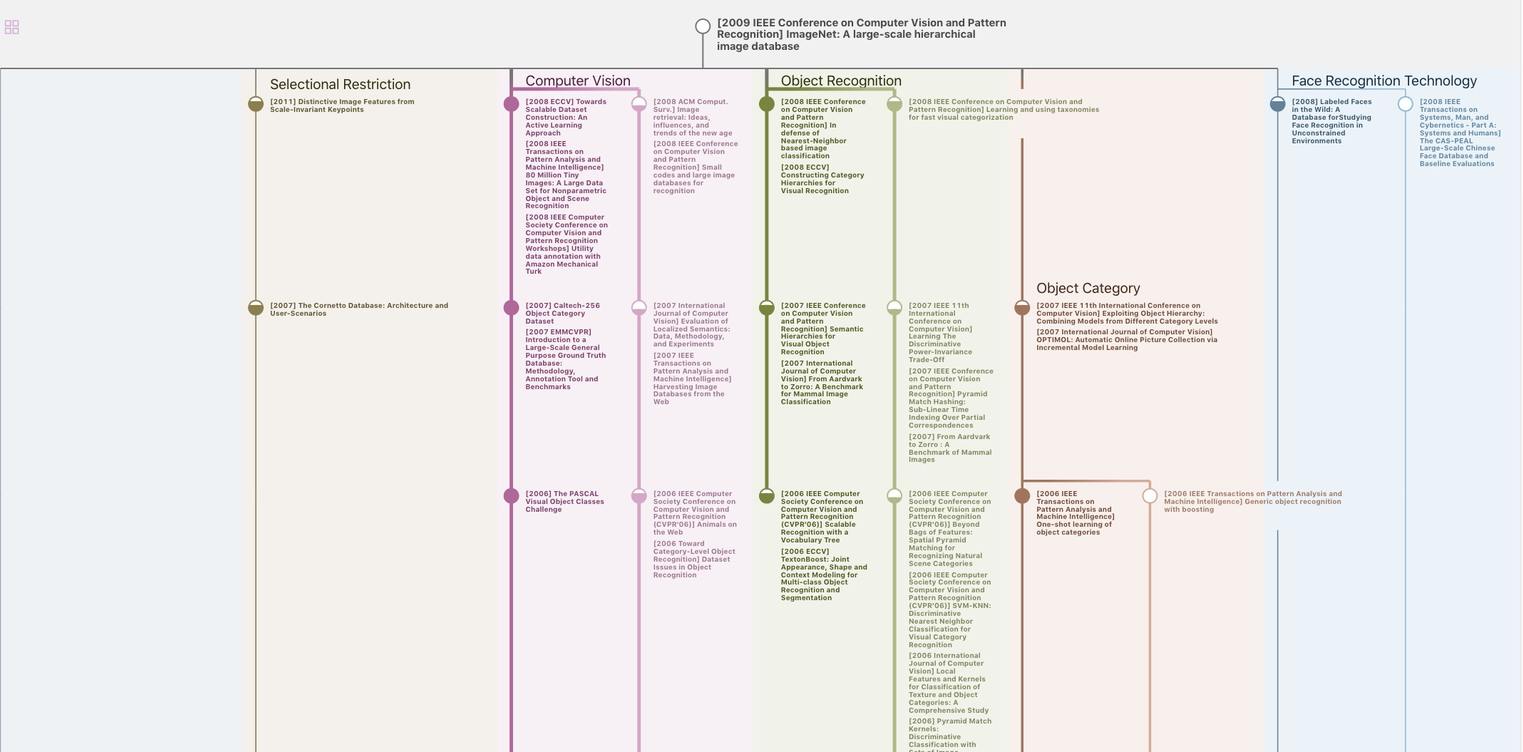
生成溯源树,研究论文发展脉络
Chat Paper
正在生成论文摘要