Fair candidate ranking with spatial partitioning: Lessons from the SIOP ML competition
HRRecSys(2021)
摘要
The ranking and selection of candidates in hiring process is an important function in human resources systems and one that is increasingly become a site for the integration of new technologies. As such implementations have grown, concerns have emerged that unwanted biases may be codified in such systems, enshrining disadvantages for minoritized groups, and therefore algorithm fairness has become an urgent concern. The recent Society for Industrial and Organizational Psychology conference organized a competition in which researchers could explore fairness in candidate ranking for hiring decisions using a data set from a large retail chain. This paper describes our solution, detailing the datamanagement (including feature engineering and missing data imputation), predictive modeling of candidate characteristics, and the multi-criteria spatial ranking algorithm that led to our successful entry.
更多查看译文
AI 理解论文
溯源树
样例
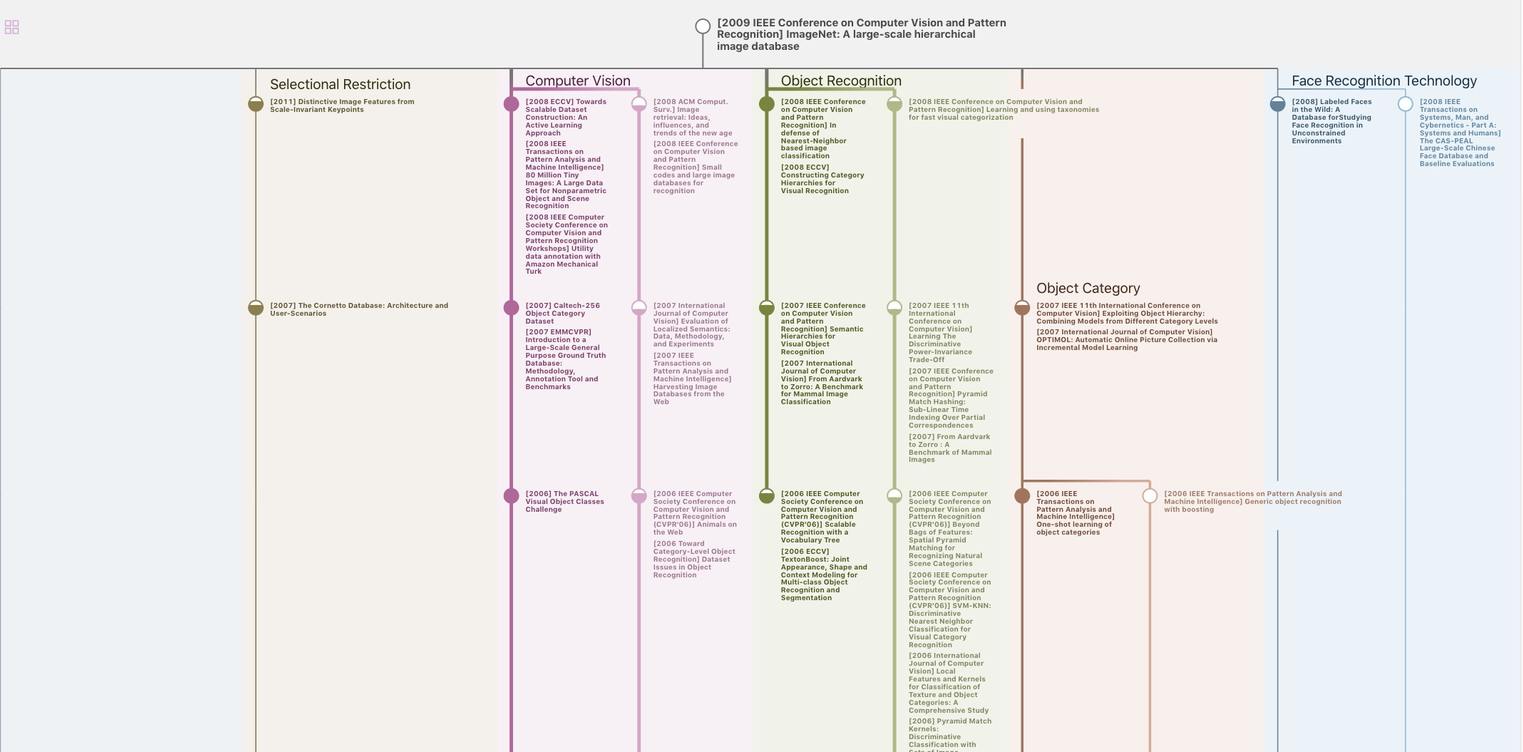
生成溯源树,研究论文发展脉络
Chat Paper
正在生成论文摘要