Denoised Internal Models: A Brain-inspired Autoencoder Against Adversarial Attacks
Machine Intelligence Research(2022)
摘要
Despite its great success, deep learning severely suffers from robustness; i.e., deep neural networks are very vulnerable to adversarial attacks, even the simplest ones. Inspired by recent advances in brain science, we propose the denoised internal models (DIM), a novel generative autoencoder-based model to tackle this challenge. Simulating the pipeline in the human brain for visual signal processing, DIM adopts a two-stage approach. In the first stage, DIM uses a denoiser to reduce the noise and the dimensions of inputs, reflecting the information pre-processing in the thalamus. Inspired by the sparse coding of memory-related traces in the primary visual cortex, the second stage produces a set of internal models, one for each category. We evaluate DIM over 42 adversarial attacks, showing that DIM effectively defenses against all the attacks and outperforms the SOTA on the overall robustness on the MNIST (Modified National Institute of Standards and Technology) dataset.
更多查看译文
关键词
Brain-inspired learning,autoencoder,robustness,adversarial attack,generative model
AI 理解论文
溯源树
样例
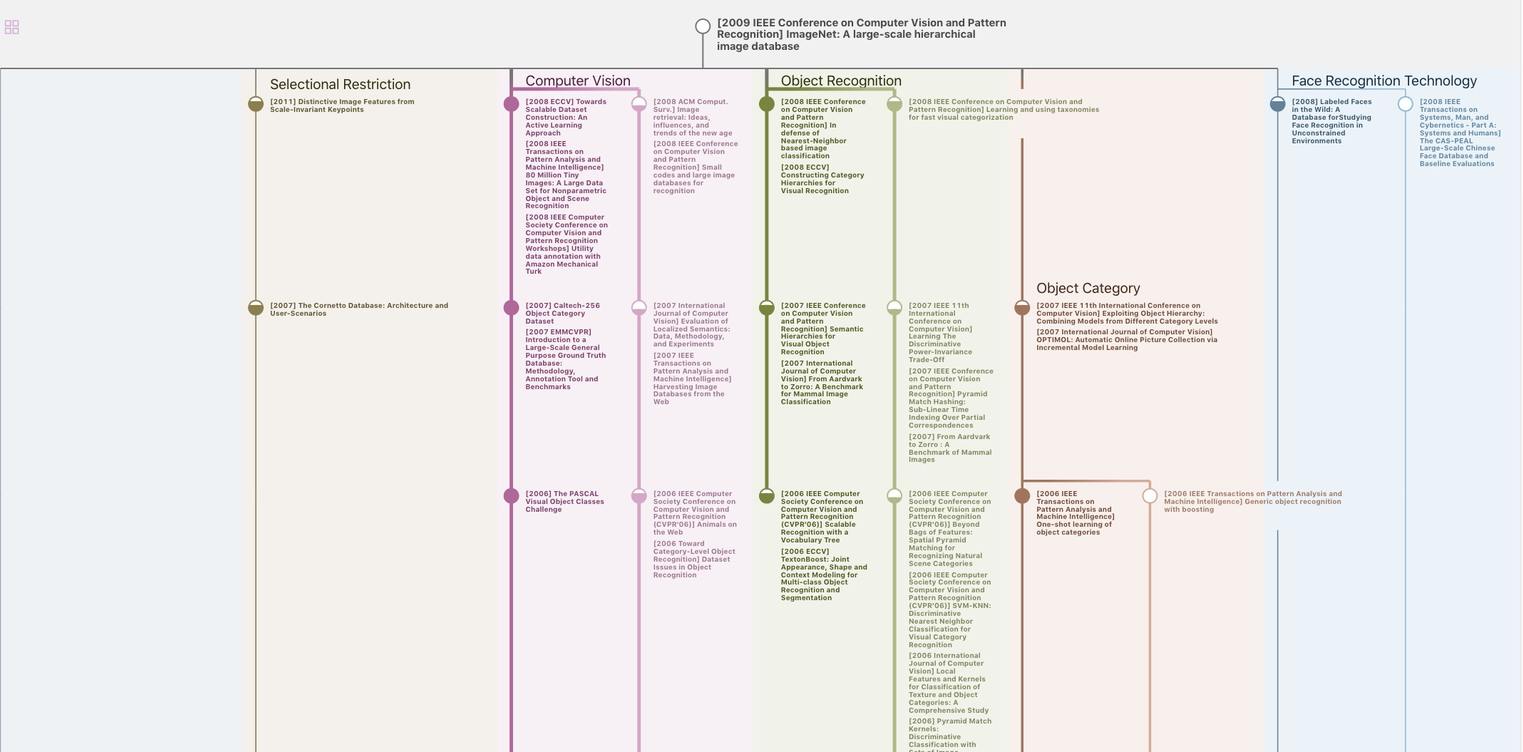
生成溯源树,研究论文发展脉络
Chat Paper
正在生成论文摘要