Randomized FIFO Mechanisms
Economics and Computation(2022)
摘要
BSTRACTWe study the matching of jobs to workers waiting in a queue, for example a ridesharing platform dispatching drivers to pick up riders at an airport. Under FIFO dispatching, the heterogeneity in earnings from different trips incentivizes drivers to cherrypick, increasing riders' waiting times for a match, and resulting in poor reliability for riders, low average earnings for drivers, and a loss of throughput and revenue for the platform. Simple fixes by limiting dispatching transparency or drivers' flexibility are neither desirable nor fully effective. Optimal origin-destination based prices are incentive aligned in theory, but are hard to implement in practice due to operational constraints. In this work, we design dispatching mechanisms that make use of drivers' waiting times in the queue to align incentives and to reduce the variability in drivers' total payoffs. We first propose the direct FIFO mechanism, which offers lower-earning trips directly to drivers further from the head of the queue. The option to skip the rest of the line incentivizes drivers to accept all dispatches, and the equilibrium outcome is envy-free and achieves the first best trip throughput and the second best net revenue. The mechanism, however, suffers from fairness concerns since drivers who have waited a long time (and are as a result close enough to the head of the queue) are no longer eligible for dispatches to most destinations. To preserve fairness of the dispatch rule in the sense that drivers closer to the head of the queue have higher priority for trips to all destinations, we introduce a family of randomized FIFO mechanisms. A randomized FIFO mechanism first offers each rider's trip request to a driver close to the head of the queue, and then sends the trip gradually down the queue in a randomized manner after each time the trip is declined by a driver. We prove that the randomized FIFO mechanisms achieve the first best throughput and second best revenue in equilibrium--- randomizing each dispatch among a small group of drivers increases each individual drivers' waiting times for the next dispatch, allowing the mechanism to prioritize drivers closer to the head of the queue for trips to every destination without creating incentives for excessive cherrypicking. Extensive counterfactual simulations using ridesharing data from the City of Chicago suggest that in comparison to the status quo strict FIFO dispatching, the randomized FIFO mechanism achieves substantial improvements in revenue, throughput, and driver earnings. Moreover, the variance in drivers' total payoffs is small, and diminishes rapidly as riders' patience increases since the randomized FIFO mechanism can more effectively match higher-earning trips with drivers who have incurred higher costs waiting in the queue. This demonstrates the desirable balance achieved by the randomized FIFO mechanisms between efficiency, fairness, and the variability in driver earnings, and highlights the effectiveness of using waiting times to align incentives and reduce inequity when the flexibility to set prices is limited due to operational constraints. Full Paper: https://arxiv.org/abs/2111.10706
更多查看译文
关键词
mechanisms
AI 理解论文
溯源树
样例
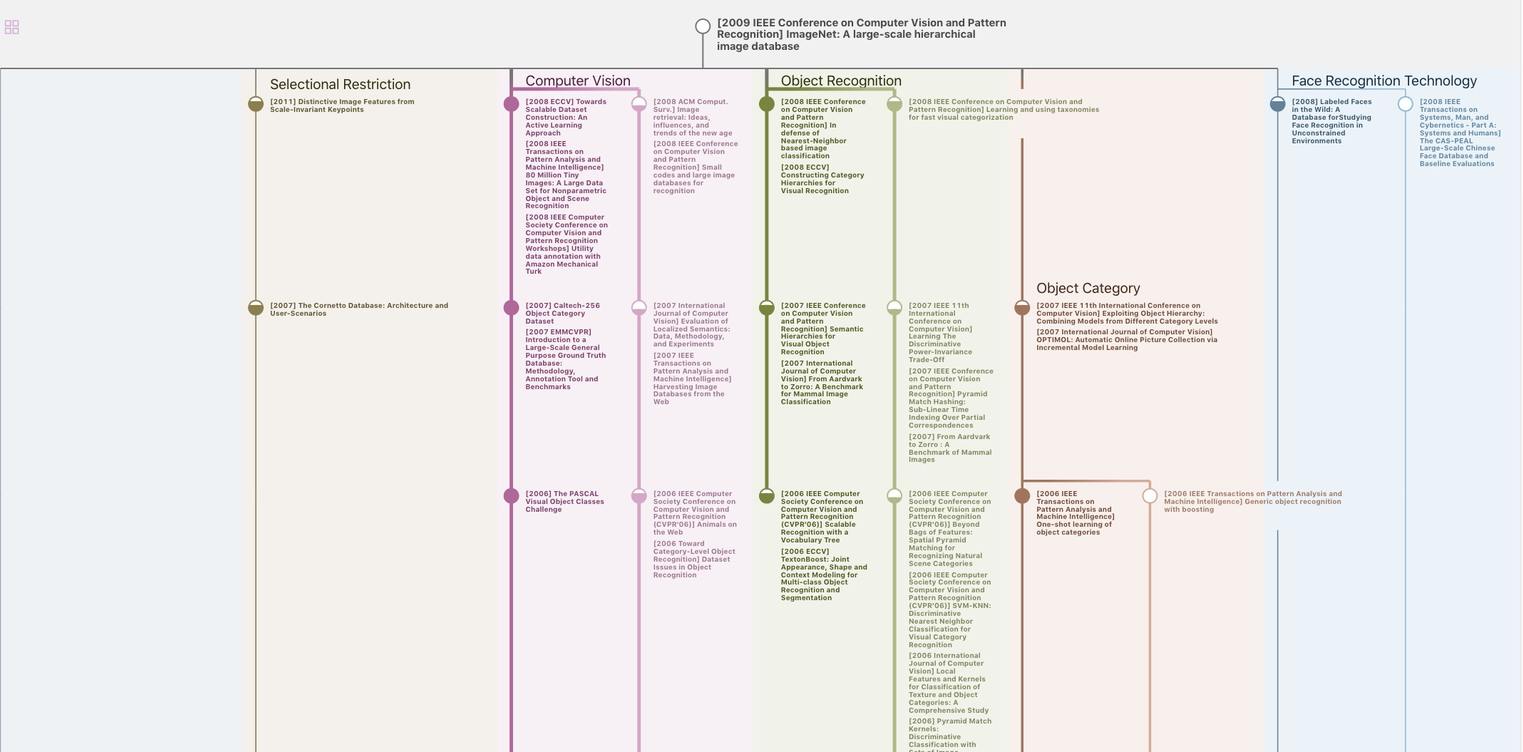
生成溯源树,研究论文发展脉络
Chat Paper
正在生成论文摘要