An Augmented Deep Learning Network with Noise Suppression Feature for Efficient Segmentation of Magnetic Resonance Images
IETE TECHNICAL REVIEW(2022)
摘要
The segmentation of cardiac MR images requires extensive attention as it needs a high level of care and analysis for the diagnosis of affected part. The advent of deep learning technology has paved the way for efficient, automatic and reliable segmentation of medical images for proper diagnosis. This manuscript proposes an augmented end to end trainable deep learning architecture for efficient segmentation of various domains of medical images. The model incorporates the depth wise separable convolution and group normalization as basic building blocks. Moreover, a noise stifler block is also induced between the encoder and decoder to counter the noise in the medical images. This path helps in delineating the precise boundary contours as the noise often reduces the boundary segmentation capability of the segmentation network. The network trained once produces exceedingly good results for the images of other datasets. An improvement of above (5 +/- 0.03) % and (3.5 +/- 0.02) % was observed in the Jaccard index and Dice score for cardiac MR images. The results are statistically validated as p < 0.05. The automatic computer investigated approach can help in reducing the burden on the medical system by producing accurate and reliable results. The algorithmic results were clinically verified by the senior radiologists by comparison with the manually segmented images. The training time of the network was about 30% less than U-net.
更多查看译文
关键词
Depth Wise Separable Convolution, CMRI, SELU, Group Normalization
AI 理解论文
溯源树
样例
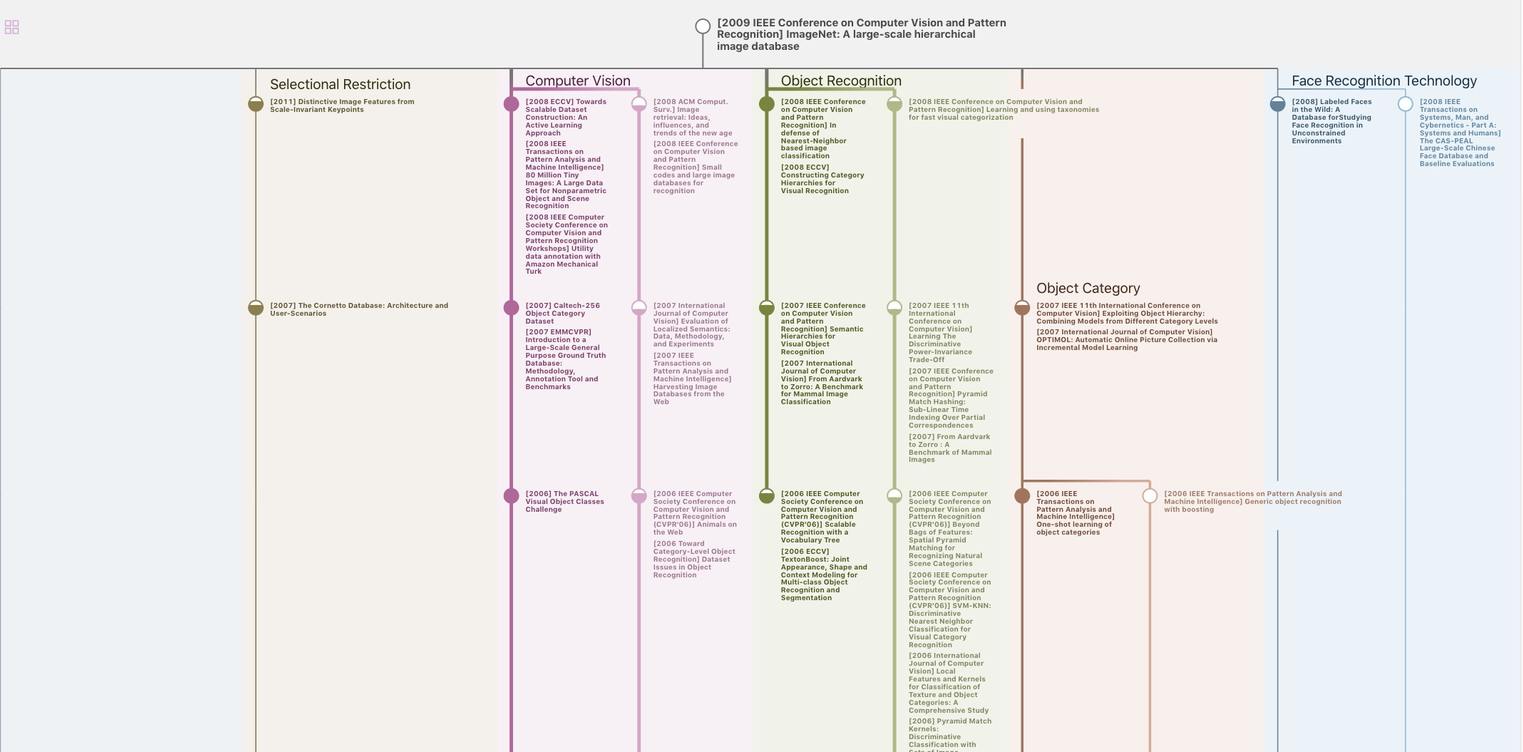
生成溯源树,研究论文发展脉络
Chat Paper
正在生成论文摘要