Uncertainty In Optimal Fingerprinting Is Underestimated
ENVIRONMENTAL RESEARCH LETTERS(2021)
摘要
Detection and attribution analyses of climate change are crucial in determining whether the observed changes in a climate variable are attributable to human influence. A commonly used method for these analyses is optimal fingerprinting, which regresses observed climate variables on the signals, climate model simulated responses under external forcings. The method scales the simulated response under each external forcing by a scaling factor to best match the observations. The method relies critically on the confidence intervals for the scaling factors. The coverage rate, the relative frequency a confidence interval containing the unknown true value of the corresponding scaling factor, in the prevailing practice has been noted to be lower than desired. The mechanism of this under-coverage and its impacts on detection and attribution analyses, however, have not been investigated. Here we show that the under-coverage is due to insufficient consideration of the uncertainty in estimating the natural variability when fitting the regression and making statistical inferences. The implication is that the ranges of uncertainties in important quantities such as attributable anthropogenic warming and climate sensitivity based on the optimal fingerprinting technique should be wider than what has been believed, especially when the signals are weak. As a remedy, we propose a calibration method to correct this bias in the coverage rate of the confidence levels. Its effectiveness is demonstrated in a simulation study with known ground truth. The use of a large sample of climate model simulations to estimate the natural variability helps to reduce the uncertainty of the scaling factor estimates, and the calibrated confidence intervals provide more valid uncertainty quantification than the uncalibrated. An application to detection and attribution of changes in mean temperature at the global, continental, and subcontinental scale demonstrates that weaker detection and attribution conclusions are obtained with calibrated confidence intervals.
更多查看译文
关键词
bootstrap calibration, climate change, confidence interval, detection and attribution, natural climate variability, uncertainty quantification
AI 理解论文
溯源树
样例
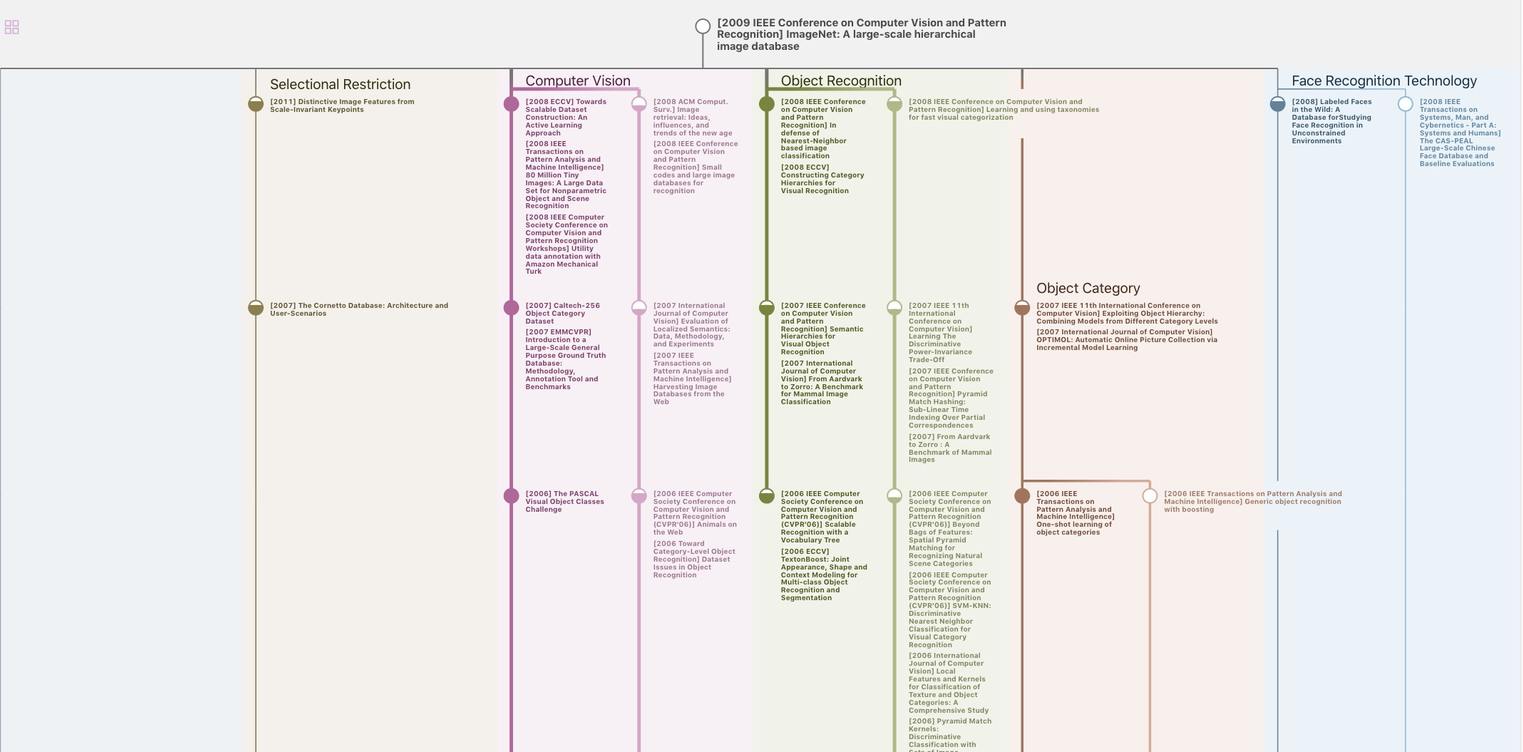
生成溯源树,研究论文发展脉络
Chat Paper
正在生成论文摘要