Vehicle Lane Change Prediction On Highways Using Efficient Environment Representation And Deep Learning
IEEE ACCESS(2021)
摘要
This paper introduces a novel method of lane-change and lane-keeping detection and prediction of surrounding vehicles based on Convolutional Neural Network (CNN) classification approach. Context, interaction, vehicle trajectories, and scene appearance are efficiently combined into a single RGB image that is fed as input for the classification model. Several state-of-the-art classification-CNN models of varying complexity are evaluated to find out the most suitable one in terms of anticipation and prediction. The model has been trained and evaluated using the PREVENTION dataset, a specific dataset oriented to vehicle maneuver and trajectory prediction. The proposed model can be trained and used to detect lane changes as soon as they are observed, and to predict them before the lane change maneuver is initiated. Concurrently, a study on human performance in predicting lane-change maneuvers using visual inputs has been conducted, so as to establish a solid benchmark for comparison. The empirical study reveals that humans are able to detect the 83.9% of lane changes on average 1.66 seconds in advance. The proposed automated maneuver detection model increases anticipation by 0.43 seconds and accuracy by 2.5% compared to human results, while the maneuver prediction model increases anticipation by 1.03 seconds with an accuracy decrease of only 0.5%.
更多查看译文
关键词
Predictive models, Global Positioning System, Trajectory, Roads, Robot sensing systems, Laser radar, Visualization, Automated highways, CNNs, deep learning, intelligent vehicles, interaction-based, lane-change prediction, prediction algorithms, surrounding vehicles
AI 理解论文
溯源树
样例
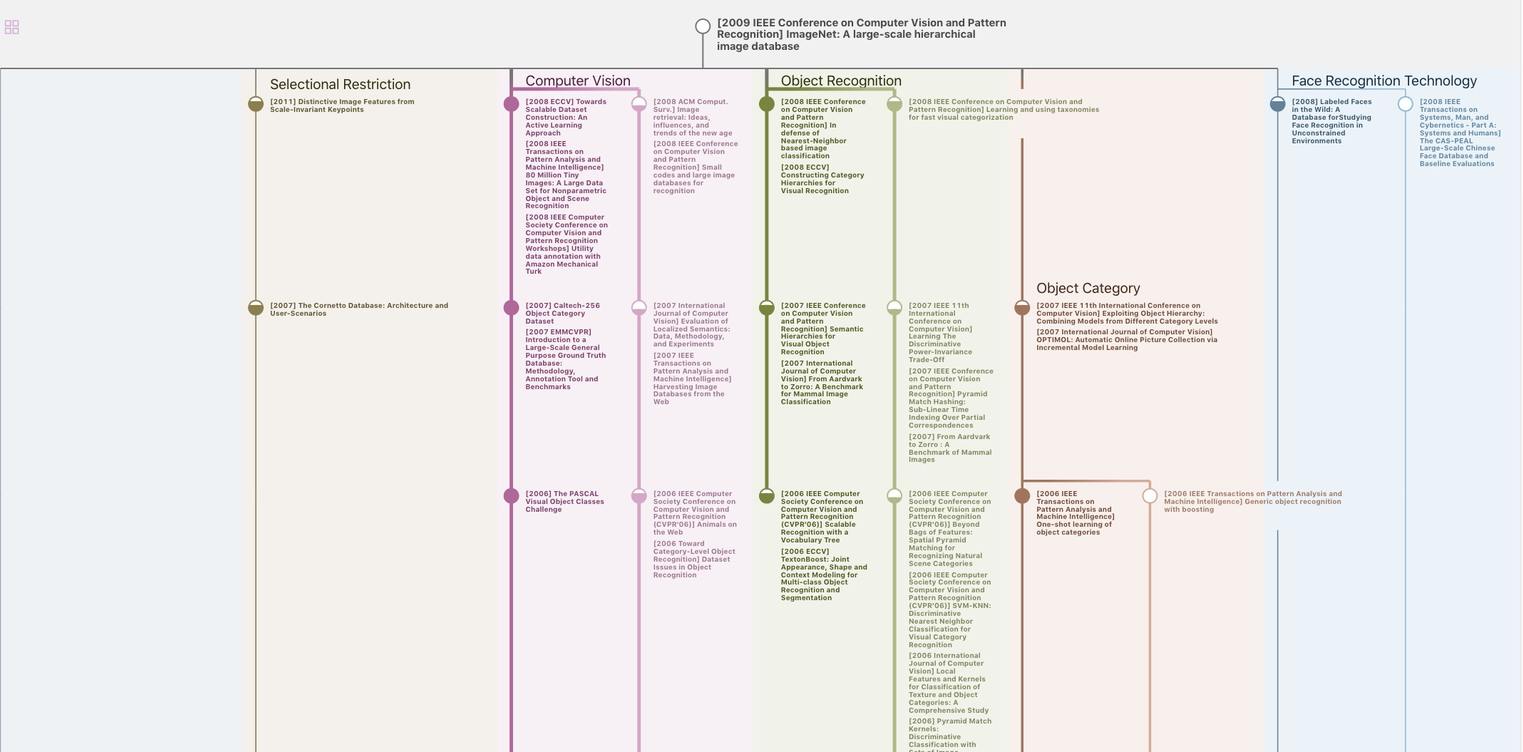
生成溯源树,研究论文发展脉络
Chat Paper
正在生成论文摘要