Gaskt: A Graph-Based Attentive Knowledge-Search Model For Knowledge Tracing
KNOWLEDGE SCIENCE, ENGINEERING AND MANAGEMENT, PT I(2021)
摘要
Knowledge tracking (KT) is a fundamental tool to customize personalized learning paths for students so that they can take charge of their own learning pace. The main task of KT is to model the learning state of the students, however the process is quite involved. First, due to the sparsity of real-world educational data, the previous KT models ignore the high-order information in question-skill; second, the long sequence of student interactions poses a demanding challenge for KT models when dealing with long-term dependencies, and the last, due to the complexity of the forgetting mechanism. To address these issues, in this paper, we propose a Graph-based Attentive Knowledge-Search Model for Knowledge Tracing (GASKT). The model divides problems and skills into two types of nodes, utilizing R-GCN to thoroughly incorporate the relevance of problem-skill through embedding propagation, which reduces the impact of sparse data. Besides, it employs the modified attention mechanism to address the long-term dependencies issue. For the attention weight score between questions, on the basis of using the scaled dot-product, the forgetting mechanism is fully considered. We conduct extensive experiments on several real-world benchmark datasets, and our GASKT outperforms the state-of-the-art KT models, with at least 1% AUC improvement.
更多查看译文
关键词
Knowledge tracing, Relational graph convolutional networks, Attention, LSTM, Forgetting mechanism
AI 理解论文
溯源树
样例
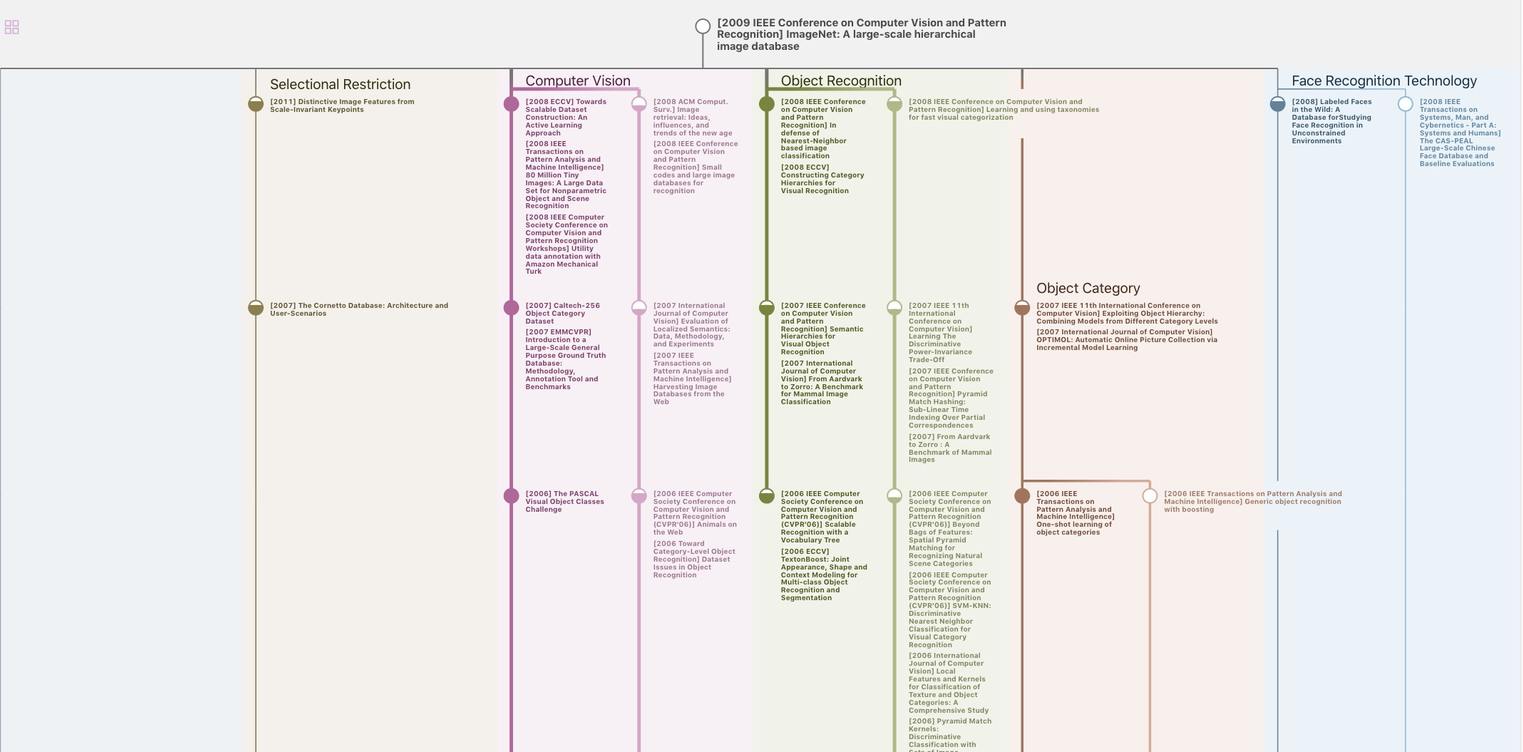
生成溯源树,研究论文发展脉络
Chat Paper
正在生成论文摘要