Dimension reduction for block-missing data based on sparse sliced inverse regression
Computational Statistics & Data Analysis(2022)
摘要
Due to the high demand of data compression in the big data era, efficient dimension reduction has become a hot statistical research topic recently. One particular challenge for dimension reduction is the block-missing problem, which is prevalent in multi-modality data. Sliced inverse regression as a classical method would not handle the data-missing issue generally. In this paper, we propose the convex sparse sliced inverse regression with elastic net, whose estimation of the central subspace and variable selection are performed simultaneously. This method can be directly applied to block-missing data without imputation. The algorithm called Adjusted Linearized Alternating Direction of Method of Multipliers (Adjusted L-ADMM) is addressed correspondingly. The asymptotic properties are investigated. Numerical results show that our method can effectively and robustly identify important covariates in the high-dimensional case.
更多查看译文
关键词
Sufficient dimension reduction,Sparse sliced inverse regression,Convex optimization,Block-missing data,Adjusted L-ADMM
AI 理解论文
溯源树
样例
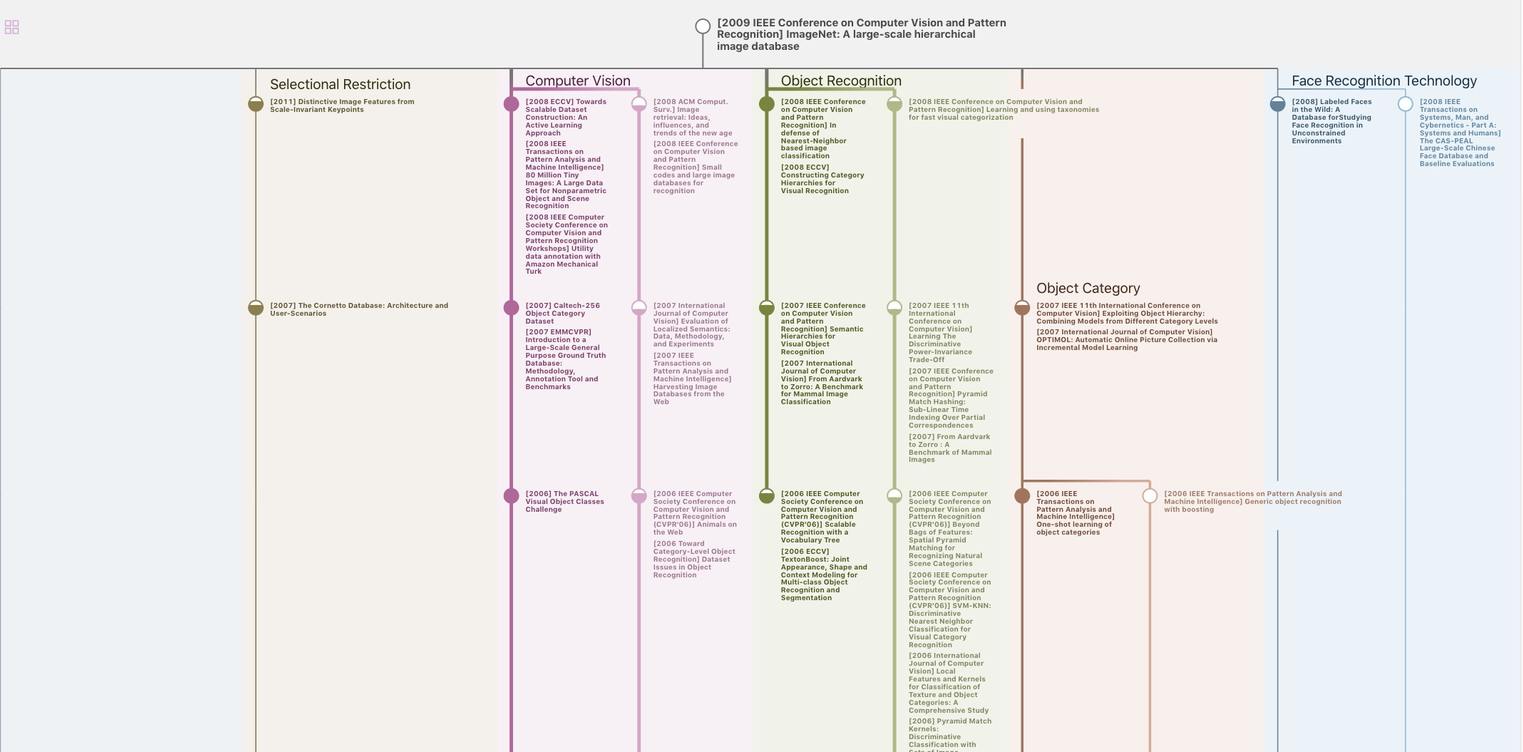
生成溯源树,研究论文发展脉络
Chat Paper
正在生成论文摘要