Gemma: Reinforcement Learning-Based Graph Embedding And Mapping For Virtual Network Applications
IEEE ACCESS(2021)
摘要
Virtual network mapping (VNM) is a challenge in the field of network virtualization. As VNM variants have been formalized depending on substrate network structures, virtual network specifications, mapping optimization objectives, and other factors, a number of VNM heuristic methods have been introduced. On the other hand, reinforcement learning (RL) algorithms have been incorporated into deep learning frameworks and recognized as a promising solution for solving complex resource allocation problems. In this paper, we present an RL-based graph embedding and mapping framework, Gemma, for tackling various VNM problems in a unified end-to-end manner. In the framework, we employ an encoder-decoder deep learning architecture and propose several optimization schemes such as two-stage mapping and model-based selective embedding. Aiming to deal with large-scale VNM problems in both online and offline scheduling systems, the proposed schemes explore the trade-off between inference accuracy and mapping function runtimes, enhancing scalability and timeliness. Gemma shows robust performance under various problem conditions, outperforming other heuristic and learning-based methods.
更多查看译文
关键词
Substrates, Task analysis, Resource management, Optimization, Computational modeling, Virtualization, Runtime, Graph Embedding, graph convolution network, reinforcement learning, virtual network mapping, virtual network embedding
AI 理解论文
溯源树
样例
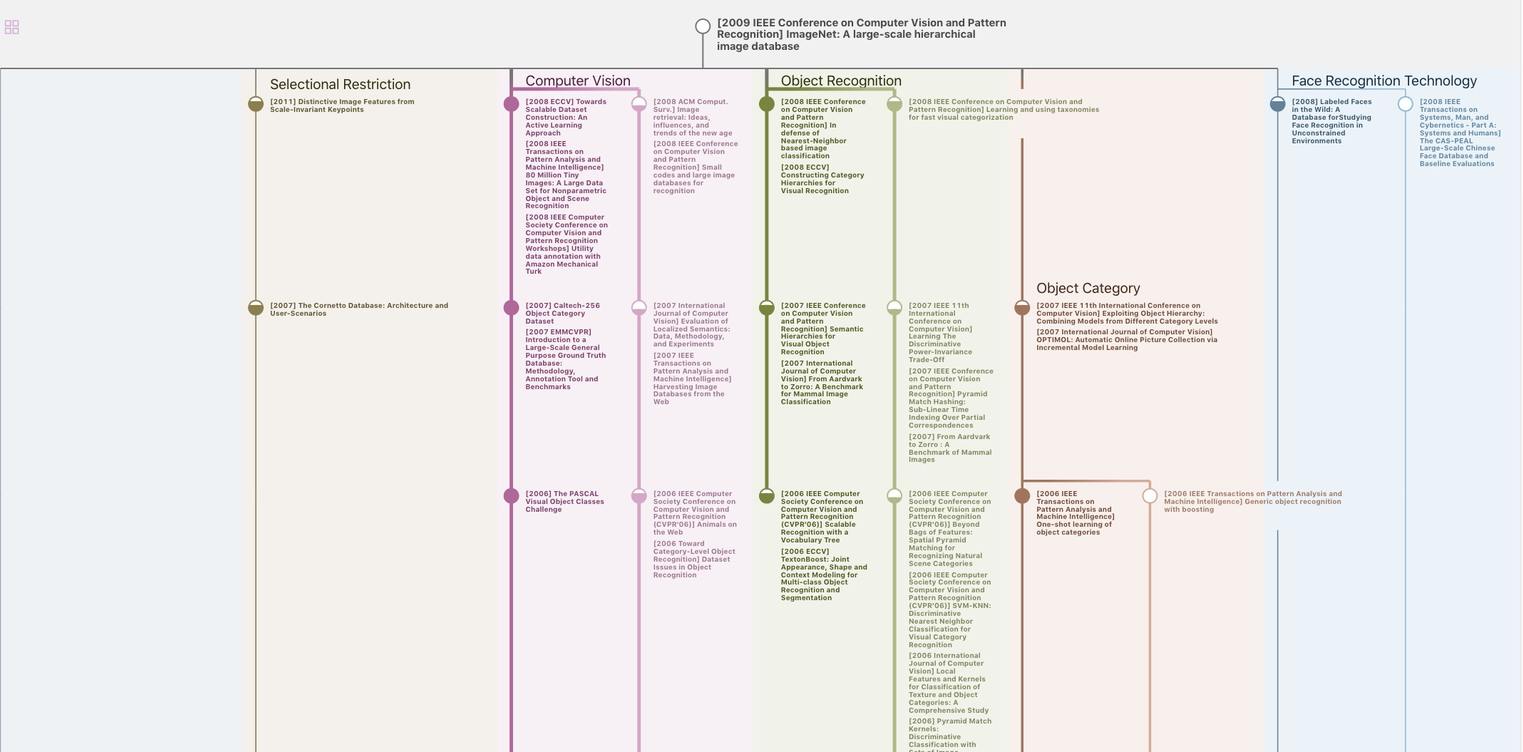
生成溯源树,研究论文发展脉络
Chat Paper
正在生成论文摘要