A Learning-Based Fault Localization Approach Using Subset Of Likely And Dynamic Invariants
INTELLIGENT AUTOMATION AND SOFT COMPUTING(2022)
摘要
Fault localization is one of the main tasks of software debugging. Developers spend a lot of time, cost, and effort to locate the faults correctly manually. For reducing this effort, many automatic fault localization techniques have been proposed, which inputs test suites and outputs a sorted list of faulty entities of the program. For further enhancement in this area, we developed a system called SILearning, which is based on invariant analysis. It learns from some existing fixed bugs to locate faulty methods in the program. It combines machine-learned ranking, program invariant differences, and spectrum-based fault localization (SBFL). Using the execution of test cases and code coverage analysis, it obtains each method's invariant differences and the suspiciousness value based on the program spectral location and uses them as features for ranking the faulty methods. The experimental analysis of SILearning has been performed on the dataset of real fault which is extracted from the database Defects4J. The tools used in this research are Daikon and cobertura for detection of the invariants and code coverage, respectively. The results show that SILearning performs better when combined features are utilized and can successfully locate the faulty methods on average for 76.1, 90.4, 108.2, 123, and 143.5 at the top positions of 1, 2, 3, 4, and 5.
更多查看译文
关键词
Automatic fault localization, program invariant, spectrum-based fault localization, learning to rank
AI 理解论文
溯源树
样例
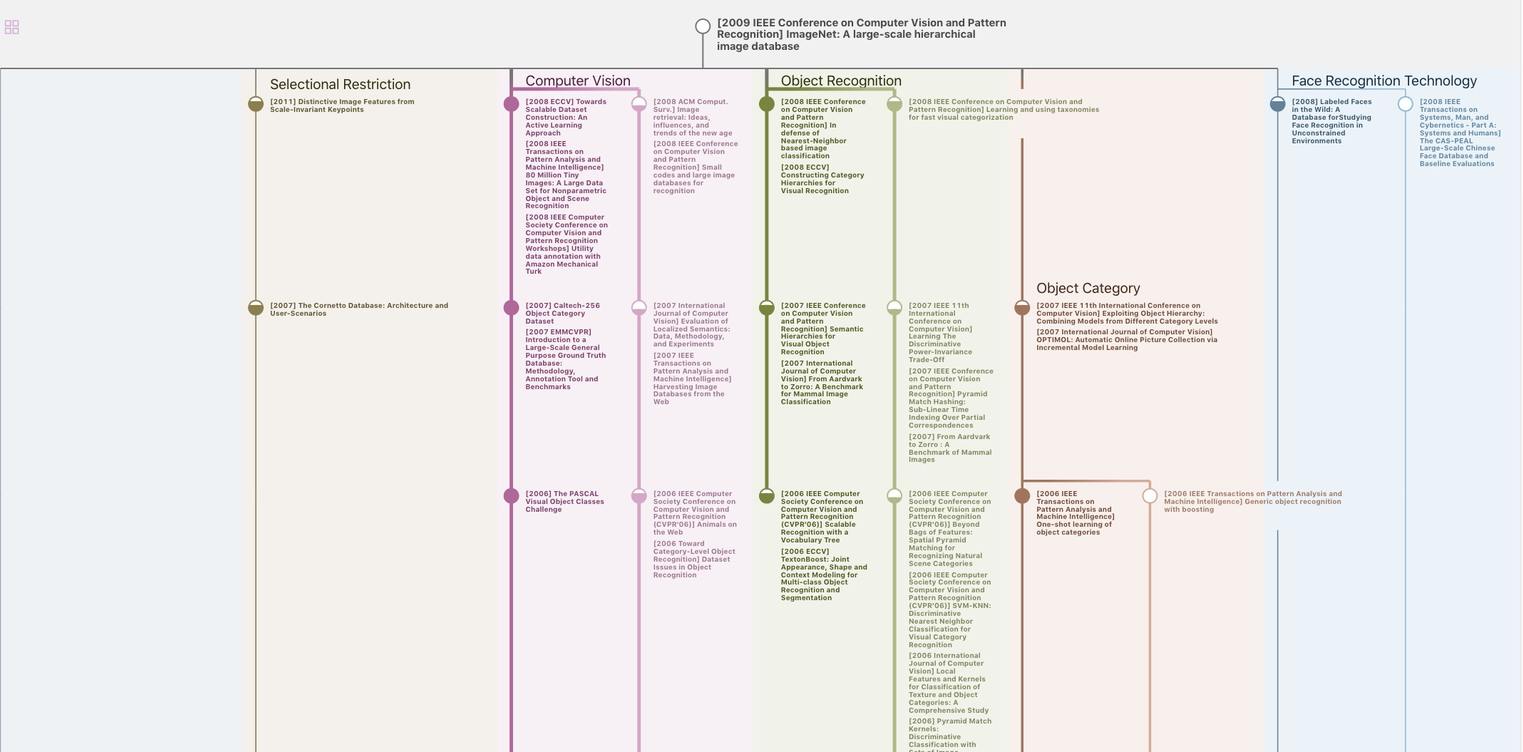
生成溯源树,研究论文发展脉络
Chat Paper
正在生成论文摘要