Dealing With Separation Or Near-To-Separation In The Model For Multinomial Response With Application To Childhood Health Seeking Behavior Data From A Complex Survey
JOURNAL OF APPLIED STATISTICS(2022)
摘要
Separation or monotone-likelihood can be observed in fitting process of a multinomial logistic model using maximum likelihood estimation (MLE) when sample size is small and/or one of the outcome categories is rare and/or there is one or more influential covariates, resulting in infinite or biased estimate of at least one regression coefficient of the model. This study investigated empirically to identify the optimal data condition to define both 'separation' and 'near-to-separation' (partial separation) and explored their consequences in MLE and provided a solution by applying a penalized likelihood approach, which has been proposed in the literature, by adding a Jeffreys prior-based penalty term to the original likelihood function to remove the first-order bias in the MLEs of the multinomial logit model via equivalent Poisson regression. Furthermore, the penalized estimating equation (PMLE) is extended to a weighted estimating equation allowing for survey-weight for analyzing data from a complex survey. The simulation study suggests that the PMLE outperforms the MLE by providing smaller amount of bias and mean squared of error and better coverage. The methods are applied to analyze data on choice of health facility for treatment of childhood diseases.
更多查看译文
关键词
Monotone-likelihood, Poisson log-linear model, penalty function, choice of health facilities
AI 理解论文
溯源树
样例
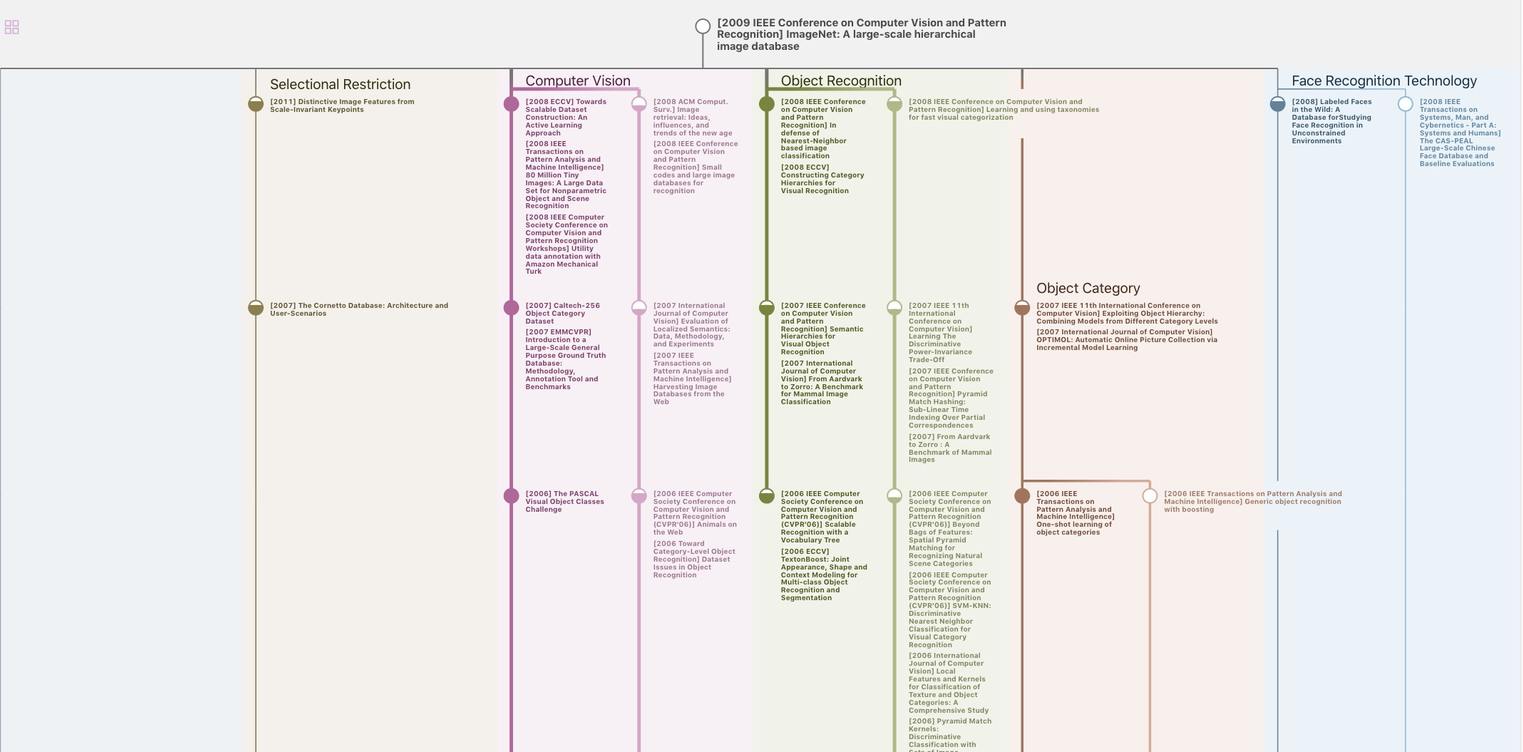
生成溯源树,研究论文发展脉络
Chat Paper
正在生成论文摘要