An efficient pruning scheme of deep neural networks for Internet of Things applications
EURASIP JOURNAL ON ADVANCES IN SIGNAL PROCESSING(2021)
摘要
Nowadays, deep neural networks (DNNs) have been rapidly deployed to realize a number of functionalities like sensing, imaging, classification, recognition, etc. However, the computational-intensive requirement of DNNs makes it difficult to be applicable for resource-limited Internet of Things (IoT) devices. In this paper, we propose a novel pruning-based paradigm that aims to reduce the computational cost of DNNs, by uncovering a more compact structure and learning the effective weights therein, on the basis of not compromising the expressive capability of DNNs. In particular, our algorithm can achieve efficient end-to-end training that transfers a redundant neural network to a compact one with a specifically targeted compression rate directly. We comprehensively evaluate our approach on various representative benchmark datasets and compared with typical advanced convolutional neural network (CNN) architectures. The experimental results verify the superior performance and robust effectiveness of our scheme. For example, when pruning VGG on CIFAR-10, our proposed scheme is able to significantly reduce its FLOPs (floating-point operations) and number of parameters with a proportion of 76.2% and 94.1%, respectively, while still maintaining a satisfactory accuracy. To sum up, our scheme could facilitate the integration of DNNs into the common machine-learning-based IoT framework and establish distributed training of neural networks in both cloud and edge.
更多查看译文
关键词
Deep neural networks,Deep learning,Internet of Things,Resource-limited edge computing,Pruning,Efficiency
AI 理解论文
溯源树
样例
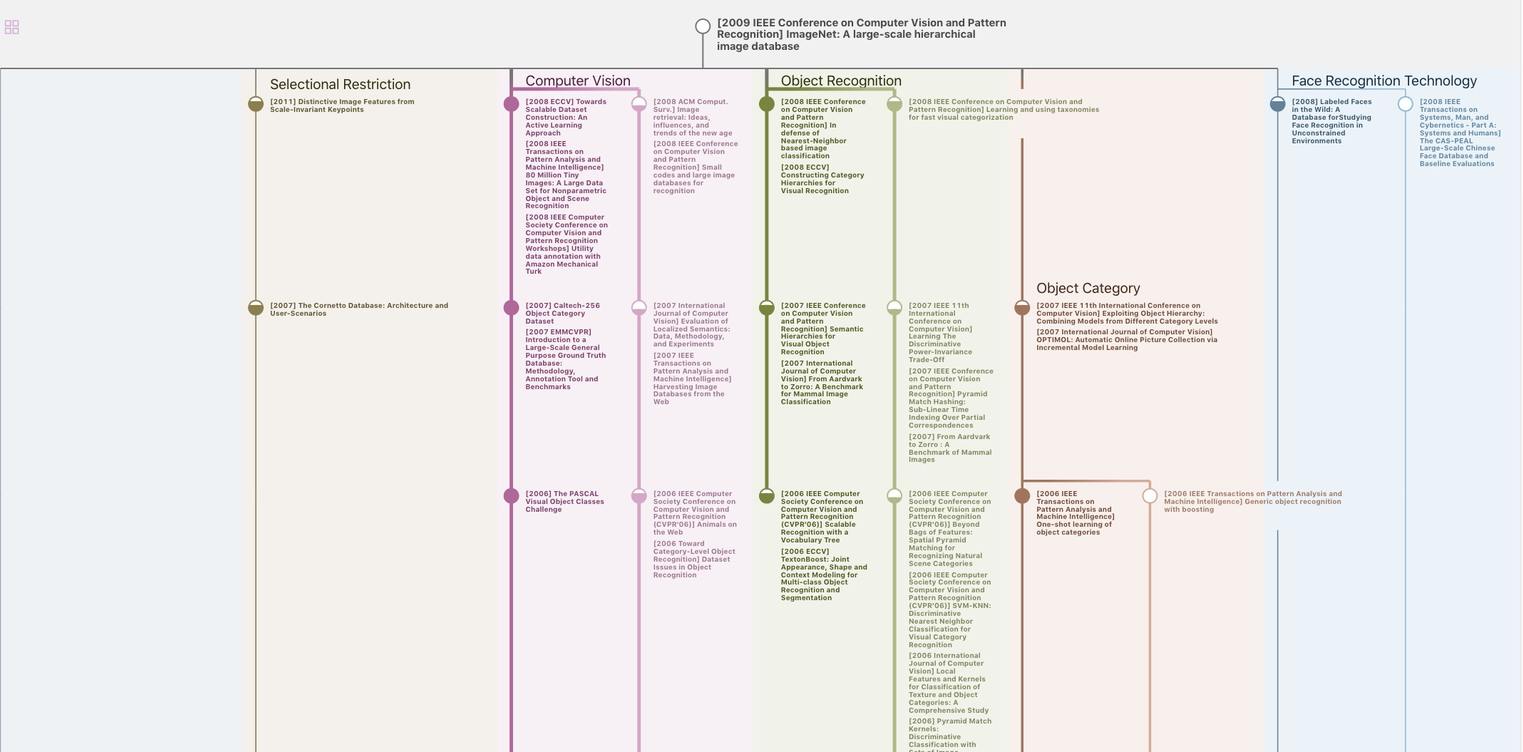
生成溯源树,研究论文发展脉络
Chat Paper
正在生成论文摘要