Interval modelling in optimization of k- N N classifiers for large number of attributes in data sets on an example of DNA microarrays
INTERNATIONAL JOURNAL OF INTELLIGENT SYSTEMS(2022)
摘要
In this contribution there are considered interval-valued methods of improving the quality of classification by the k- N N binary classifiers in the case of large number of attributes in data sets. One of the possible applications of the introduced method are the microarray data so the presented results may be applied in medical diagnosis support, for example, in identification of marker genes. The proposed algorithm involving reduction methods of time complexity and interval modeling of data using interval-valued aggregation functions has a significantly higher classification quality than the other considered algorithm with the aggregation method involving the numerical arithmetic mean. The former algorithm is based on the interval-valued aggregation of uncertainty intervals determined on the basis of certainty coefficients of individual k- N N classifiers while the latter is based on the aggregation of certainty coefficients of individual k- N N classifiers with the use of the numerical arithmetic mean. Moreover, the performance of the new algorithm based on interval-valued methods was compared with the known from the literature classifiers in cancer diagnosis based on gene expression microarrays. The obtained results prove that in practical applications the newly proposed algorithm can be successfully used.
更多查看译文
关键词
aggregation function, classifier, interval-valued fuzzy set, k-NN classifier, microarray data
AI 理解论文
溯源树
样例
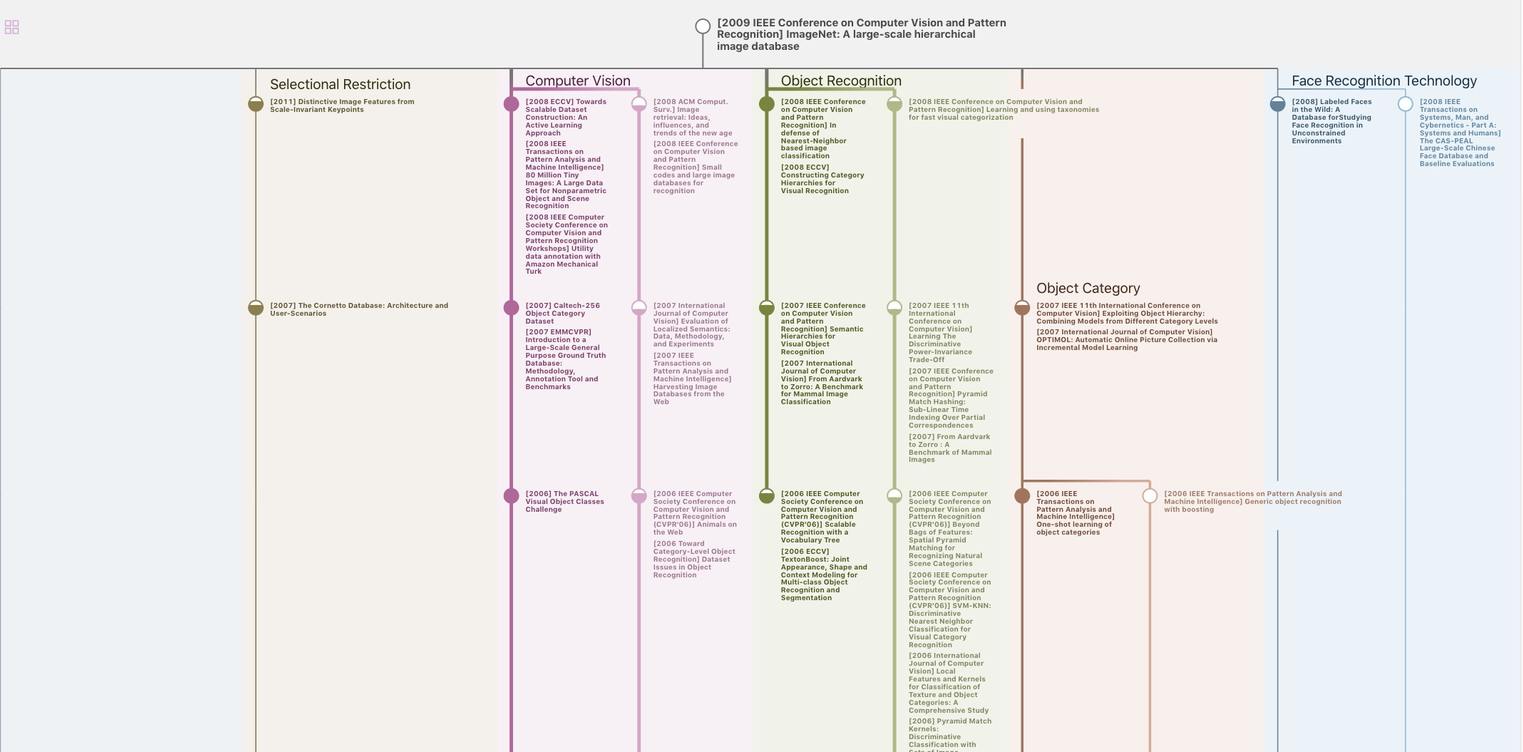
生成溯源树,研究论文发展脉络
Chat Paper
正在生成论文摘要