Improving Semi-Supervised Learning For Audio Classification With Fixmatch
ELECTRONICS(2021)
摘要
Including unlabeled data in the training process of neural networks using Semi-Supervised Learning (SSL) has shown impressive results in the image domain, where state-of-the-art results were obtained with only a fraction of the labeled data. The commonality between recent SSL methods is that they strongly rely on the augmentation of unannotated data. This is vastly unexplored for audio data. In this work, SSL using the state-of-the-art FixMatch approach is evaluated on three audio classification tasks, including music, industrial sounds, and acoustic scenes. The performance of FixMatch is compared to Convolutional Neural Networks (CNN) trained from scratch, Transfer Learning, and SSL using the Mean Teacher approach. Additionally, a simple yet effective approach for selecting suitable augmentation methods for FixMatch is introduced. FixMatch with the proposed modifications always outperformed Mean Teacher and the CNNs trained from scratch. For the industrial sounds and music datasets, the CNN baseline performance using the full dataset was reached with less than 5% of the initial training data, demonstrating the potential of recent SSL methods for audio data. Transfer Learning outperformed FixMatch only for the most challenging dataset from acoustic scene classification, showing that there is still room for improvement.
更多查看译文
关键词
semi-supervised learning, deep learning, industrial sound analysis, music information retrieval, acoustic scene classification
AI 理解论文
溯源树
样例
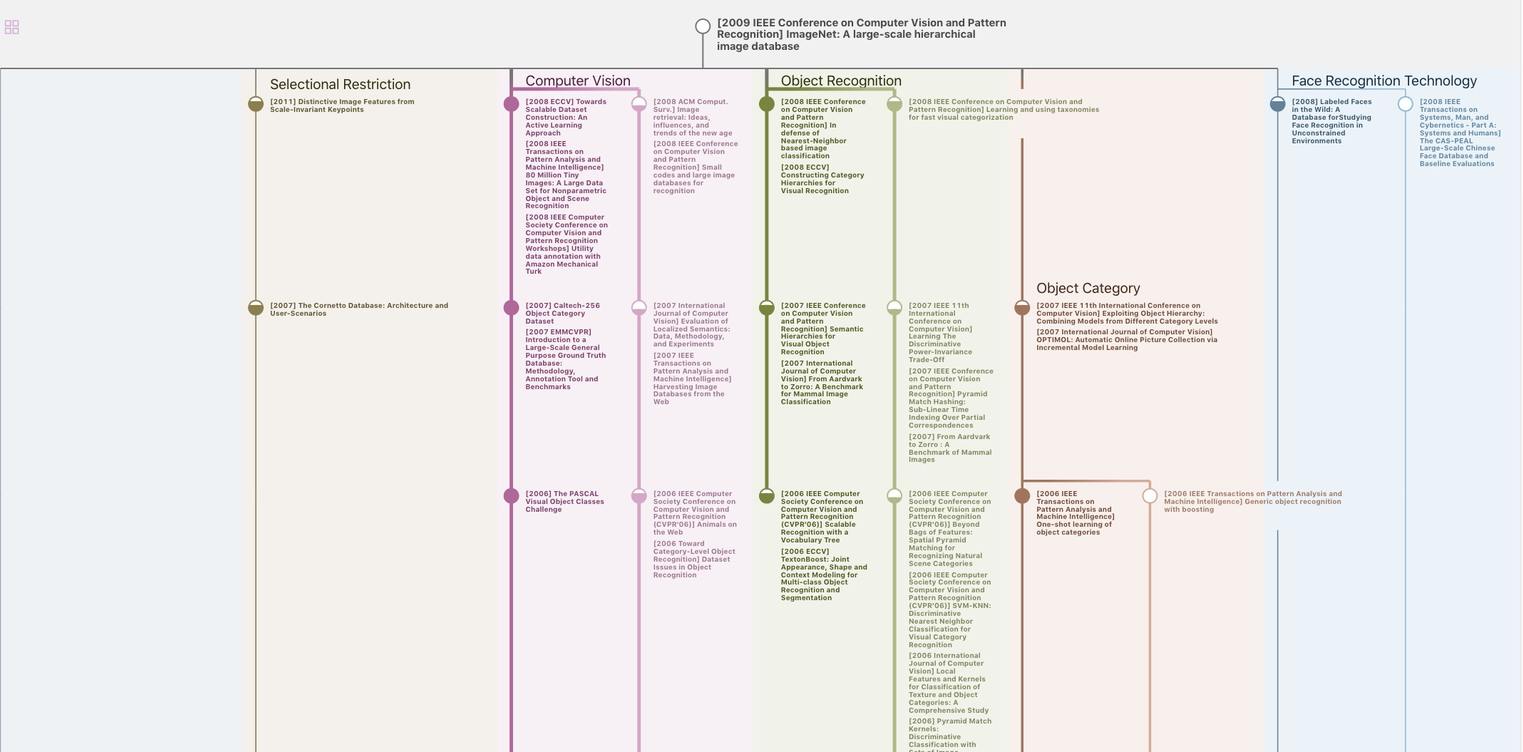
生成溯源树,研究论文发展脉络
Chat Paper
正在生成论文摘要