Studying critical frequency bands and channels for EEG-based automobile sound recognition with machine learning
Applied Acoustics(2022)
摘要
As for subjective methods (such as scale scores) for evaluation of automobile sound quality, electroencephalogram (EEG) can objectively and real-time reflect human mental states. Thus, EEG was introduced here to study the method of automobile sound recognition in view of frequency bands and channels. An EEG acquisition instrument was utilized to simultaneously acquire the EEGs of subjects stimulated by engine, exhaust and in-car sounds, and the power spectra of EEG were extracted by means of Welch Algorithm to construct a feature matrix; Then, the accuracies of automobile sound recognition based on five machine learning models were compared to determine the optimal model and the best frequency band range; What’ more, considering the differences between subjects, the m-ReliefF algorithm was applied here to investigate the universality channel with the optimal weight. Our results indicated that the differences between the three kinds of automobile sounds can be effectively identified by means of the KNN model, whose mean of accuracy peaks up to 87.93%; In addition, the recognition accuracies of beta and gamma bands were far higher than that of alpha and theta bands. Also, the accuracies of top universality 9 and 12 channels were up to 89.9% and 92.8% respectively, which were even better than that of the original 63 channels. This study revealed that recognition accuracy and computational efficiency can be significantly improved through the precise localization of the frequency bands and channels related with the automobile sounds, and demonstrated that EEG-based evaluation of the automobile sound quality shall be feasible.
更多查看译文
关键词
Automobile sound,EEG,Machine learning,Critical frequency bands,Critical channels
AI 理解论文
溯源树
样例
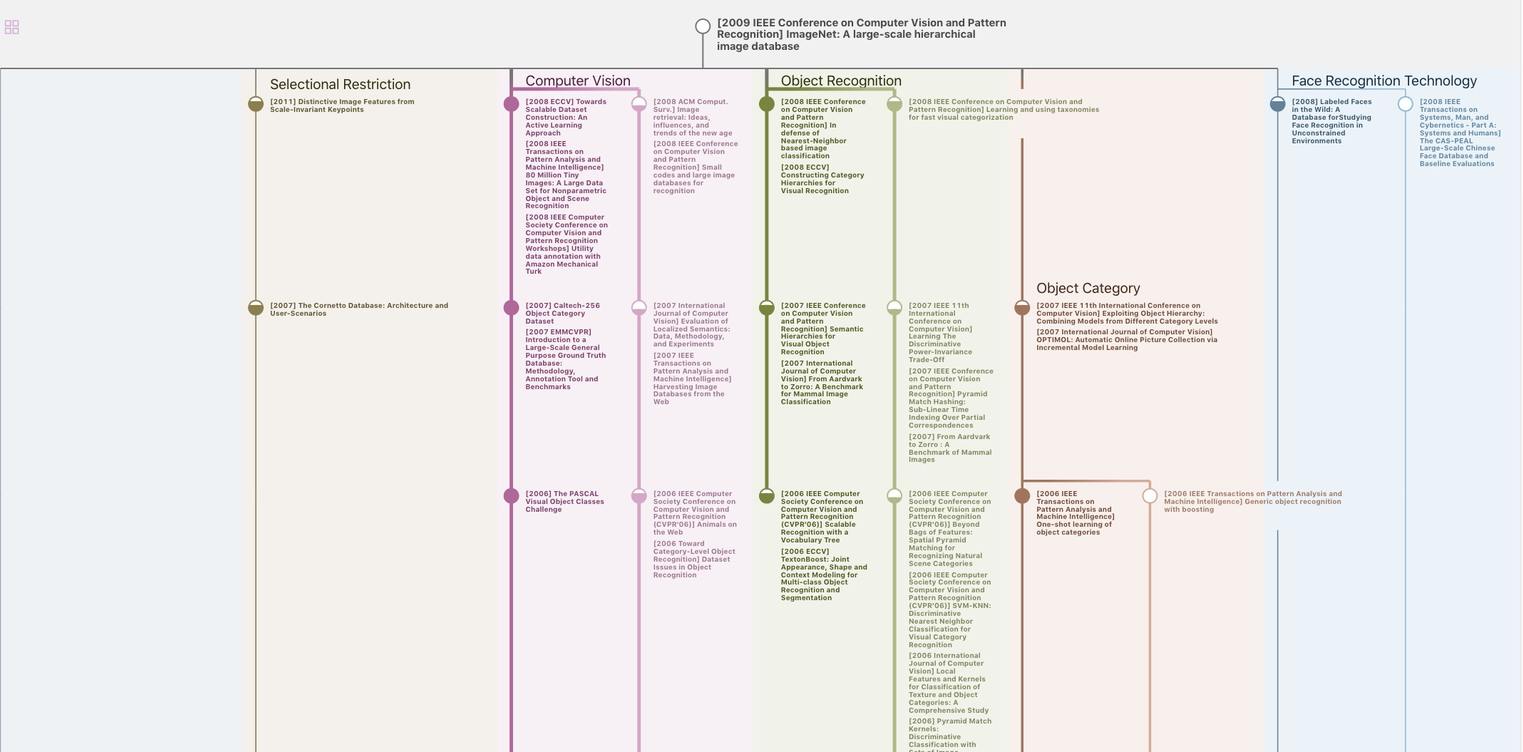
生成溯源树,研究论文发展脉络
Chat Paper
正在生成论文摘要