Prospective Validation Of Vesical Imaging-Reporting And Data System Using A Next-Generation Magnetic Resonance Imaging Scannerd-Is Denoising Deep Learning Reconstruction Useful?
JOURNAL OF UROLOGY(2021)
摘要
Purpose: The Vesical Imaging Reporting and Data System (VI-RADS) was launched in 2018 to standardize reporting of magnetic resonance imaging for bladder cancer. This study aimed to prospectively validate VI-RADS using a next-generation magnetic resonance imaging scanner and to investigate the usefulness of denoising deep learning reconstruction.Materials and Methods: We prospectively enrolled 98 patients who underwent bladder multiparametric magnetic resonance imaging using a next-generation magnetic resonance imaging scanner before transurethral resection of bladder tumor. Tumors were categorized according to VI-RADS, and we ultimately analyzed 68 patients with pathologically confirmed urothelial bladder cancer. We used receiving operating characteristic curve analyses to assess the predictive accuracy of VI-RADS for muscle invasion. Sensitivity, specificity, positive/negative predictive value, accuracy and area under the curve were calculated for different VI-RADS score cutoffs.Results: Muscle invasion was detected in the transurethral resection of bladder tumor specimens of 18 patients (26%). The optimal cutoff value of the VI-RADS score was determined as >= 4 based on the receiver operating curve analyses. The accuracy of diagnosing muscle invasion using a cutoff of VI-RADS >= 4 was 94% (AUC 0.92). Additionally, we assessed the utility of denoising deep learning reconstruction. Combination with denoising deep learning reconstruction significantly improved the AUC of category by T2-weighted imaging, and of the 4 patients who were misdiagnosed by the final VI-RADS score 3 were correctly diagnosed by T2-weighted imaging+denoising deep learning reconstruction.Conclusions: In this prospective validation study with a next-generation magnetic resonance imaging scanner, VI-RADS showed high predictive accuracy for muscle invasion in patients with bladder cancer before transurethral resection of bladder tumor. Combining T2-weighted imaging with denoising deep learning reconstruction might further improve the diagnostic accuracy of VI-RADS.
更多查看译文
关键词
urinary bladder neoplasms, deep learning, magnetic resonance imaging, prospective studies
AI 理解论文
溯源树
样例
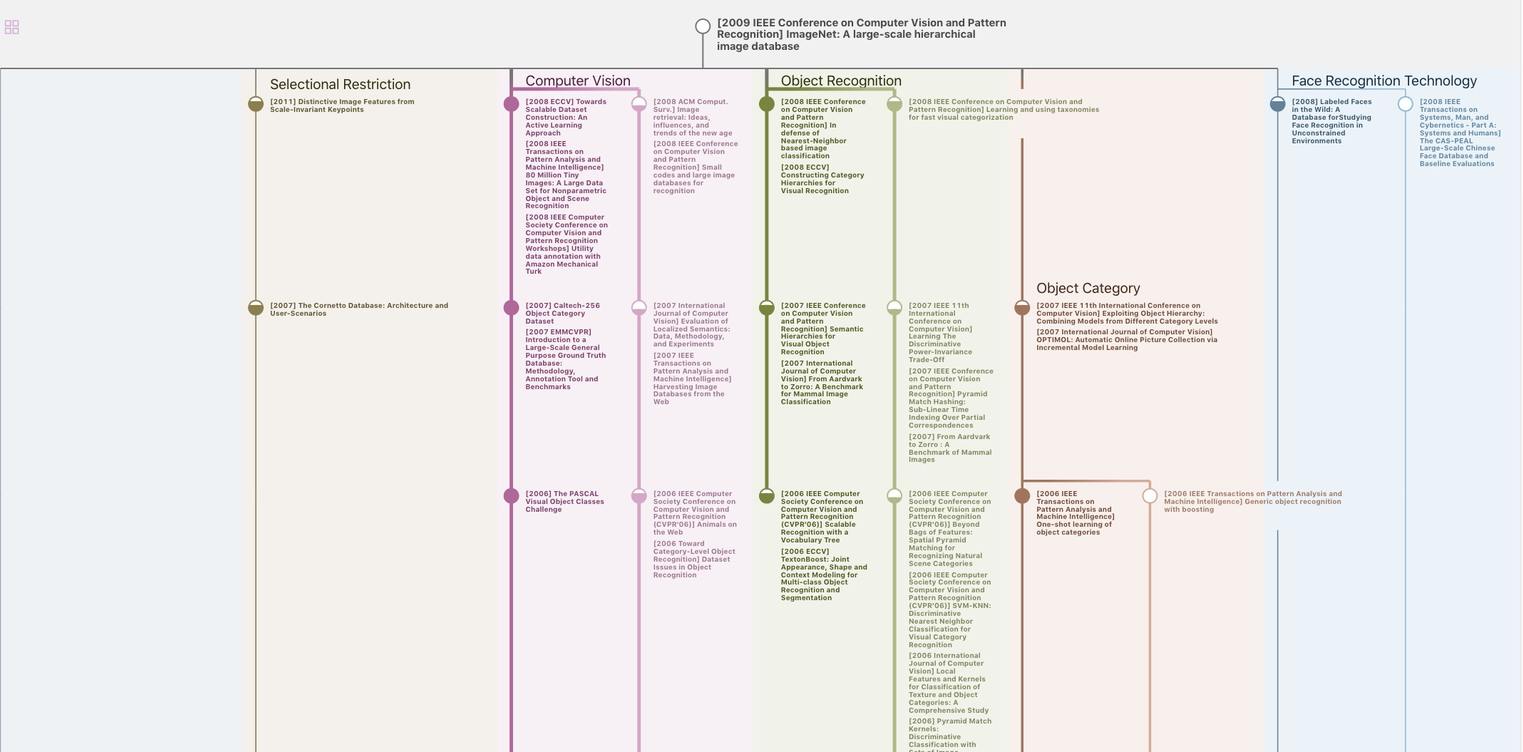
生成溯源树,研究论文发展脉络
Chat Paper
正在生成论文摘要