Quantitative Monitoring Of Bolt Looseness Using Multichannel Piezoelectric Active Sensing And Cbam-Based Convolutional Neural Network
FRONTIERS IN MATERIALS(2021)
摘要
The bolted connection is widely utilized in engineering to practically and rigidly couple structural components. The integrity of the connection is paramount to the safety of the structure and has prompted the development of many monitoring methods, including the piezoelectricity-based active sensing method. However, the active sensing method cannot quantify bolt looseness due to the unclear relationship between bolt looseness and the single monitoring index typically used in the active sensing method. Thus, the authors propose the unique combination of a one-dimensional convolutional neural network (1DCNN) and multichannel active sensing for quantitative monitoring of bolted connections. In an experiment, piezoelectric ceramic transducer (PZT) patches are bonded on steel plates connected by a bolt. Each patch is wired to a multichannel active sensing monitoring system. After obtaining multichannel stress wave signals at different looseness levels, a looseness vector is calculated to generate training and validation datasets. A baseline 1DCNN model and a novel model improved using the convolutional block attention module (CBAM) are used to monitor the bolt looseness. Finally, the authors verify that the multichannel active sensing method combined with the 1DCNN model can accurately perform quantitative monitoring of bolt looseness, and the monitoring accuracy of the baseline 1DCNN model is above 91.07% in three different specimens. Compared with the baseline 1DCNN model, the monitoring accuracy of the CBAMCNN model improved by approximately 5%. Overall, the method proposed in this article offers a new and highly accurate approach for quantitative monitoring of bolted connections.
更多查看译文
关键词
bolt looseness, quantitative monitoring, multichannel active sensing method, convolutional neural network, convolutional block attention module
AI 理解论文
溯源树
样例
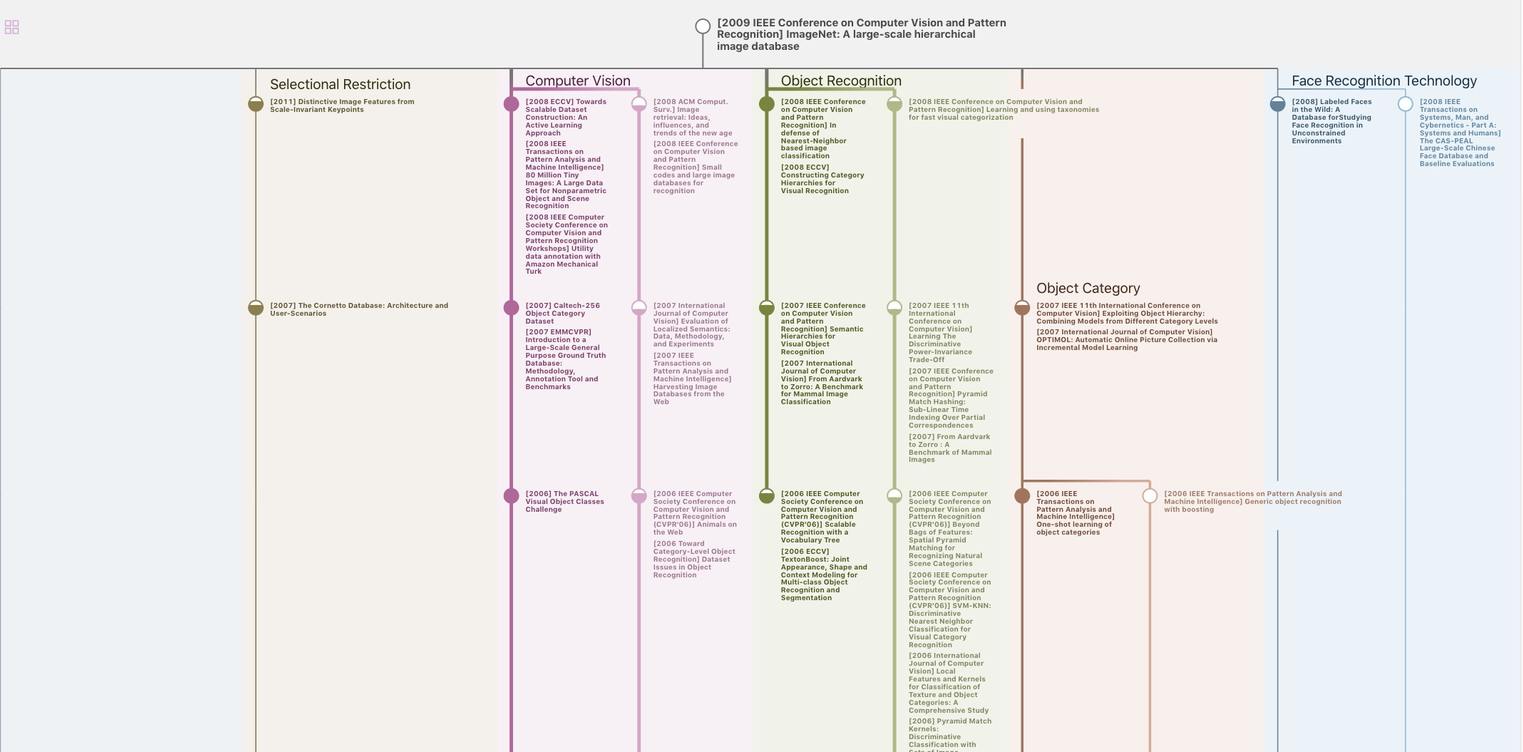
生成溯源树,研究论文发展脉络
Chat Paper
正在生成论文摘要