Multistart global optimization with tunnelling and an evolutionary strategy supervised by a martingale
ENGINEERING OPTIMIZATION(2022)
摘要
Global optimization is one of the most difficult research topics in the optimization area. In the past few decades, many stochastic methods have been proposed that proved to be effective. In this article, a new multistart global optimization method, using tunnelling and an evolutionary strategy and supervised by a martingale (MGO-TEM) is proposed, which also belongs to the stochastic family. MGO-TEM is under a multistart framework such that a local search method will be called when a new sample point is generated. A sampling technique that is a hybrid of tunnelling and an evolutionary strategy is proposed in MGO-TEM with a set of stopping criteria whose derivation is based on martingale theory. Through experiments, MGO-TEM is compared with 10 other well-known algorithms on 55 single-objective test functions, and the results show that MGO-TEM is superior to all of them. Moreover, MGO-TEM is user-friendly since there are only two parameters to be tuned.
更多查看译文
关键词
Global optimization, martingale, stochastic process, multistart
AI 理解论文
溯源树
样例
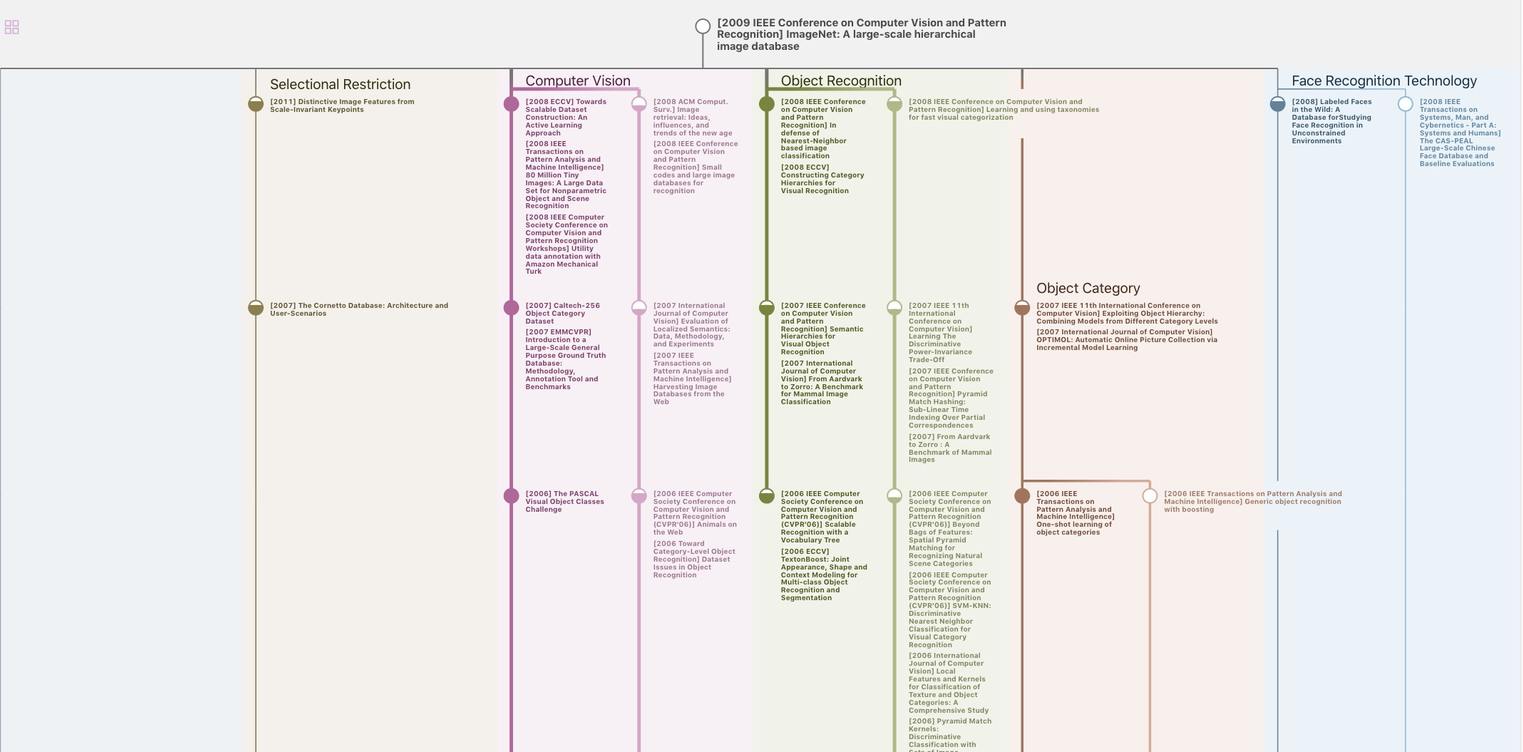
生成溯源树,研究论文发展脉络
Chat Paper
正在生成论文摘要