A Deep Learning Approach For Fast Detection And Classification Of Concrete Damage
AUTOMATION IN CONSTRUCTION(2021)
摘要
The detection and classification of concrete damage are essential for keeping the infrastructure in good condition which have become a focused area. It is worth noting that the inference speed and accuracy of object detection algorithms are the key issues in concrete damage detection tasks. This research has made the following contributions to solving these problems. Firstly, a concrete damage data set is prepared. The data set consists of 5000 images containing the types of crack, spot, rebar exposure and spalling damage. Secondly, the object detection algorithm is optimized by depthwise separable convolution, inverse residual network and linear bottleneck structure. The experimental results show that the inference speed of the improved object detection algorithm is 24.1% and 53.5% higher than that of the original network, respectively. Compared with the original YOLO-v3 and SSD object detection algorithms, the complete detection accuracy of the network reaches 64.81% and 64.12%, which increased by 3.25% and 4.04%, respectively.
更多查看译文
关键词
Concrete damage, Object detection, Image classification, YOLO network, SSD network
AI 理解论文
溯源树
样例
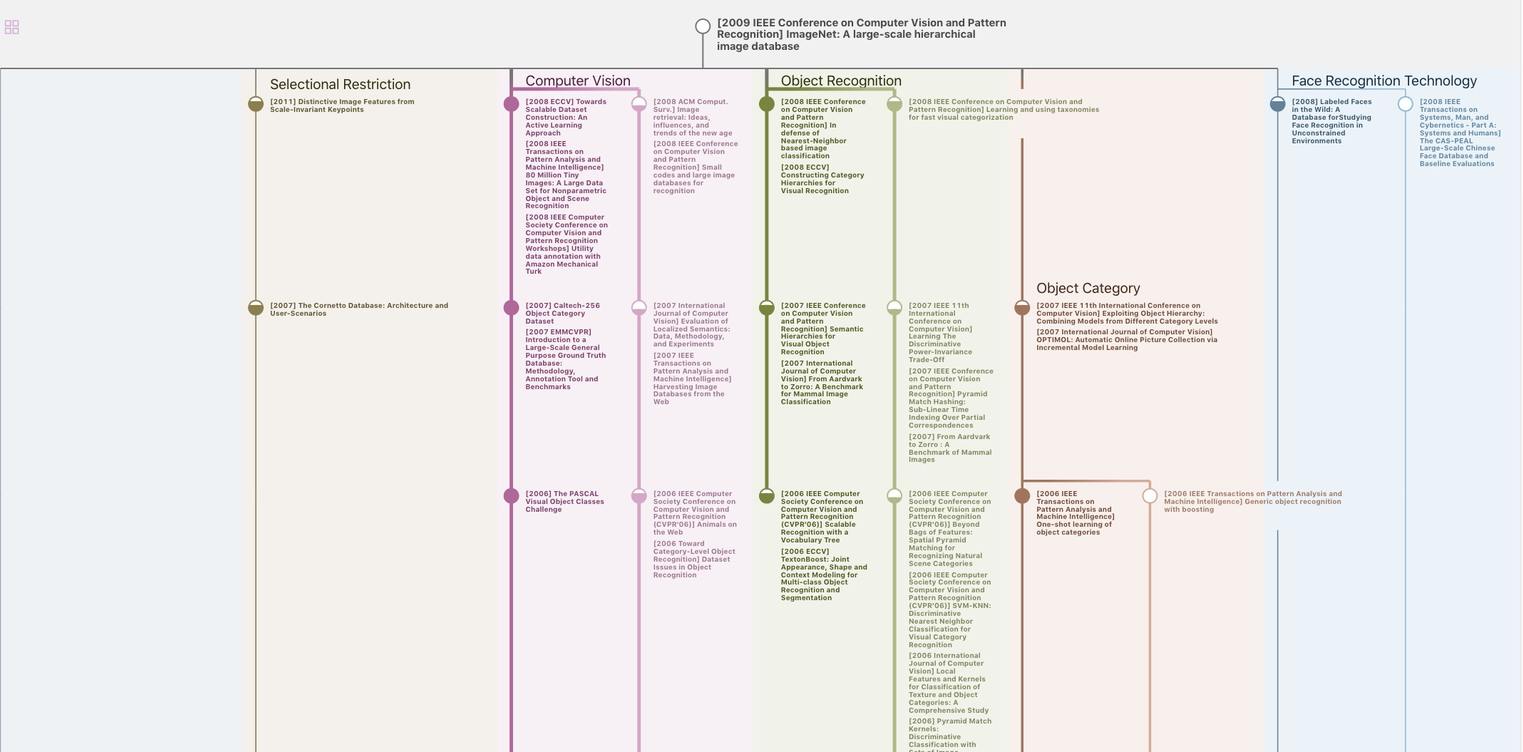
生成溯源树,研究论文发展脉络
Chat Paper
正在生成论文摘要