Improved orbit predictions using two-line elements through error pattern mining and transferring
Acta Astronautica(2021)
摘要
As the sole orbit data source of space objects for public access, the NORAD catalog provides the basic orbital information in the form of two-line element (TLE) for various space tasks. But the accuracy of orbit prediction (OP) using TLE with the analytical Simplified General Perturbations-4 (SGP4) propagator drops quickly with time, especially for low orbits, significantly limiting the capability of TLEs for advanced space situational awareness (SSA) applications. To enhance the TLE performance over long-duration OP, this paper proposes a data-driven method for improved TLE-based orbit predictions through mining and transferring the orbit error patterns. Two state-of-the-art learning methods, the gradient boosting decision tree (GBDT) and convolutional neural networks (CNN), are applied to model the underlying error patterns, and then the learned models are used as an error corrector to modify the future orbit predictions. Experimental results demonstrate that the time-varying orbit error patterns in the past can be captured by the developed learning framework. As a result, the accuracy of orbit predictions over the future 14 days can be improved by more than 75% in the along-track direction and 90% in the cross-track and radial directions, when the model-predicted errors are used. It also shows that the error pattern learning and application process is computationally efficient, and it could be implemented for near real-time applications. This study demonstrates the promising potential of machine learning (ML)/deep learning (DL) for enhanced SSA capability with the publicly available TLEs.
更多查看译文
关键词
Space objects,Two-line element,Orbit prediction error,Gradient boosting decision tree,Convolutional neural networks
AI 理解论文
溯源树
样例
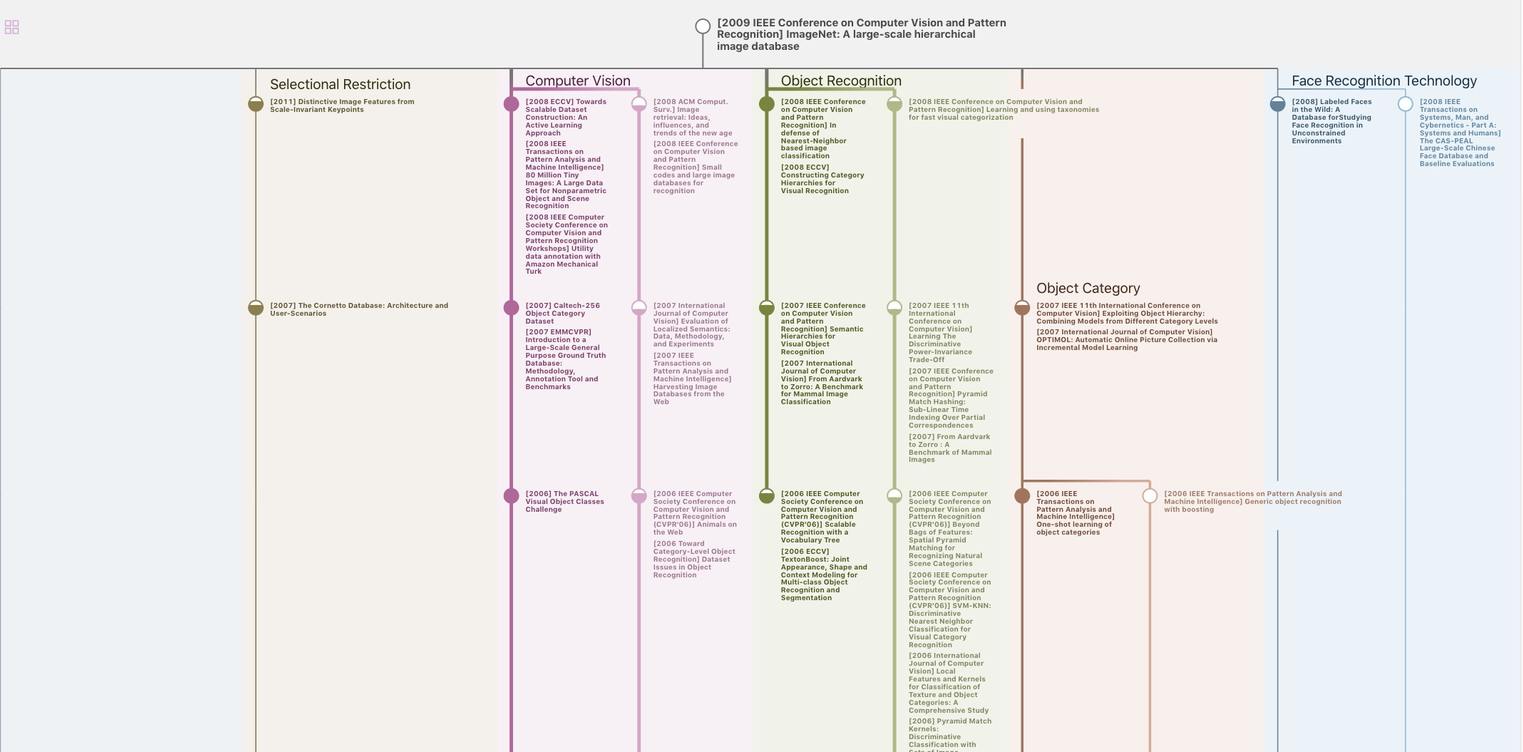
生成溯源树,研究论文发展脉络
Chat Paper
正在生成论文摘要