Construction of a deep sparse filtering network for rotating machinery fault diagnosis
PROCEEDINGS OF THE INSTITUTION OF MECHANICAL ENGINEERS PART D-JOURNAL OF AUTOMOBILE ENGINEERING(2022)
摘要
Deep neural networks (DNNs) have shown potential in intelligent fault diagnosis of rotating machinery. However, traditional DNNs such as the back-propagation neural network are highly sensitive to the initial weights and easily fall into the local optimum, which restricts the feature learning capability and diagnostic performance. To overcome the above problems, a deep sparse filtering network (DSFN) constructed by stacked sparse filtering is developed in this paper and applied to fault diagnosis. The developed DSFN is pre-trained by sparse filtering in an unsupervised way. The back-propagation algorithm is employed to optimize the DSFN after pre-training. Then, the DSFN-based intelligent fault diagnosis method is validated using two experiments. The results show that pre-training with sparse filtering and fine-tuning can help the DSFN search for the optimal network parameters, and the DSFN can learn discriminative features adaptively from rotating machinery datasets. Compared with classical methods, the developed diagnostic method can diagnose rotating machinery faults with higher accuracy using fewer training samples.
更多查看译文
关键词
Intelligent fault diagnosis, rotating machinery, deep neural network, deep learning, sparse filtering
AI 理解论文
溯源树
样例
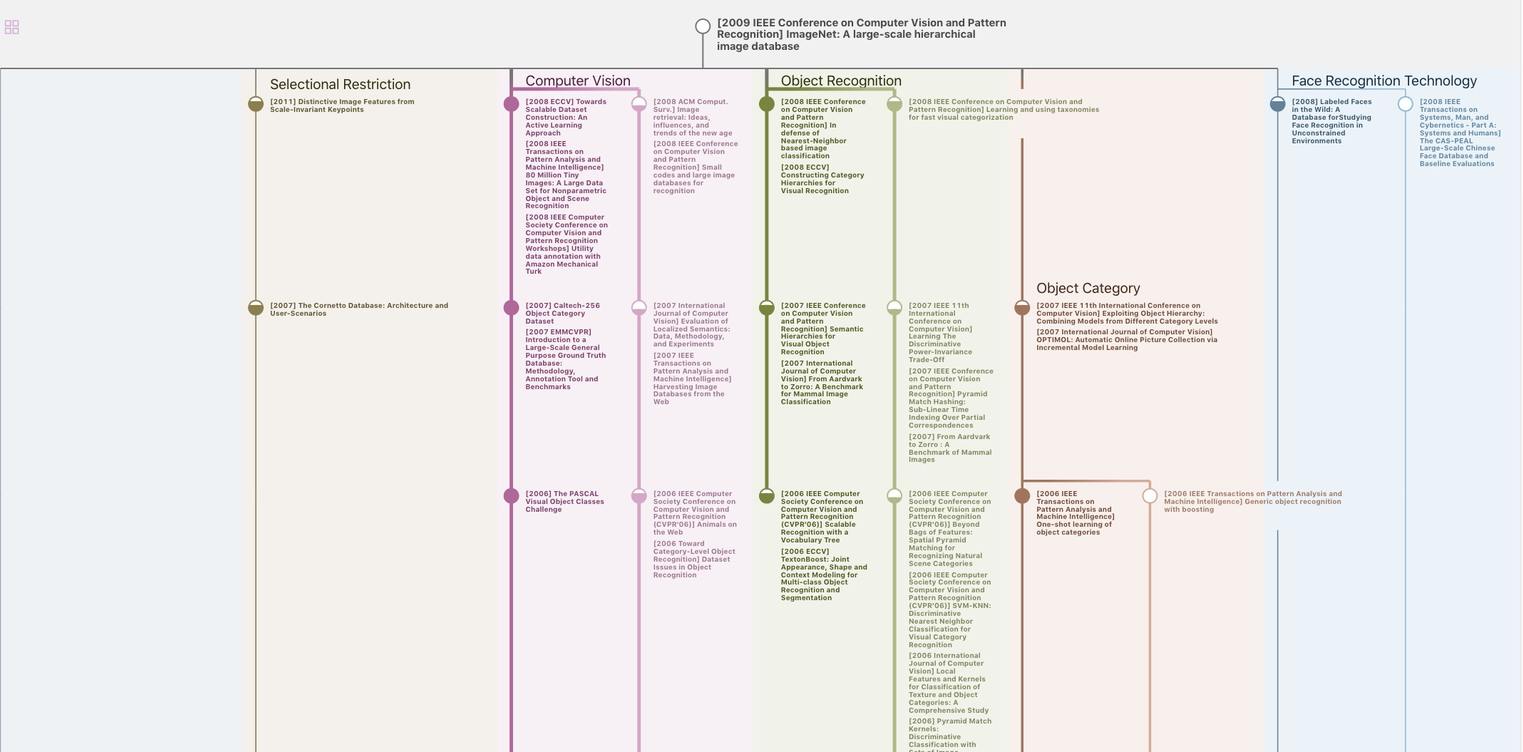
生成溯源树,研究论文发展脉络
Chat Paper
正在生成论文摘要