Traffic state classification and prediction based on trajectory data
JOURNAL OF INTELLIGENT TRANSPORTATION SYSTEMS(2022)
摘要
With the rapid increasing of car usage, congestion is becoming more and more severe in metropolitans. To tackle this problem, the classification and prediction of traffic states are vital for the authority to quickly react. This study proposes a machine learning method to identify and predict traffic states at small region levels. In this paper, we compare two different spatial division methods, namely rectangular grids and hexagonal grids and measure the traffic characteristics through aggregating floating car trajectory data. Then a dimensionality reduction technique is applied to the traffic parameter, followed by a clustering model for traffic state recognition and labeling. From the analysis in this study, speed is found to be an important feature for classifying the traffic state. Therefore, two Convolutional Neural Network (CNN) models using different architecture were used for both speed and traffic state prediction, which could consider the spatial and temporal features simultaneously. Random forest regression, Gradient boosting decision tree and Long-short term memory neural network model are used as baseline models. The case study performed in Chengdu demonstrates that the proposed model outperforms the baseline models.
更多查看译文
关键词
convolutional neural network,floating car data,k-means,traffic state estimation,traffic state prediction
AI 理解论文
溯源树
样例
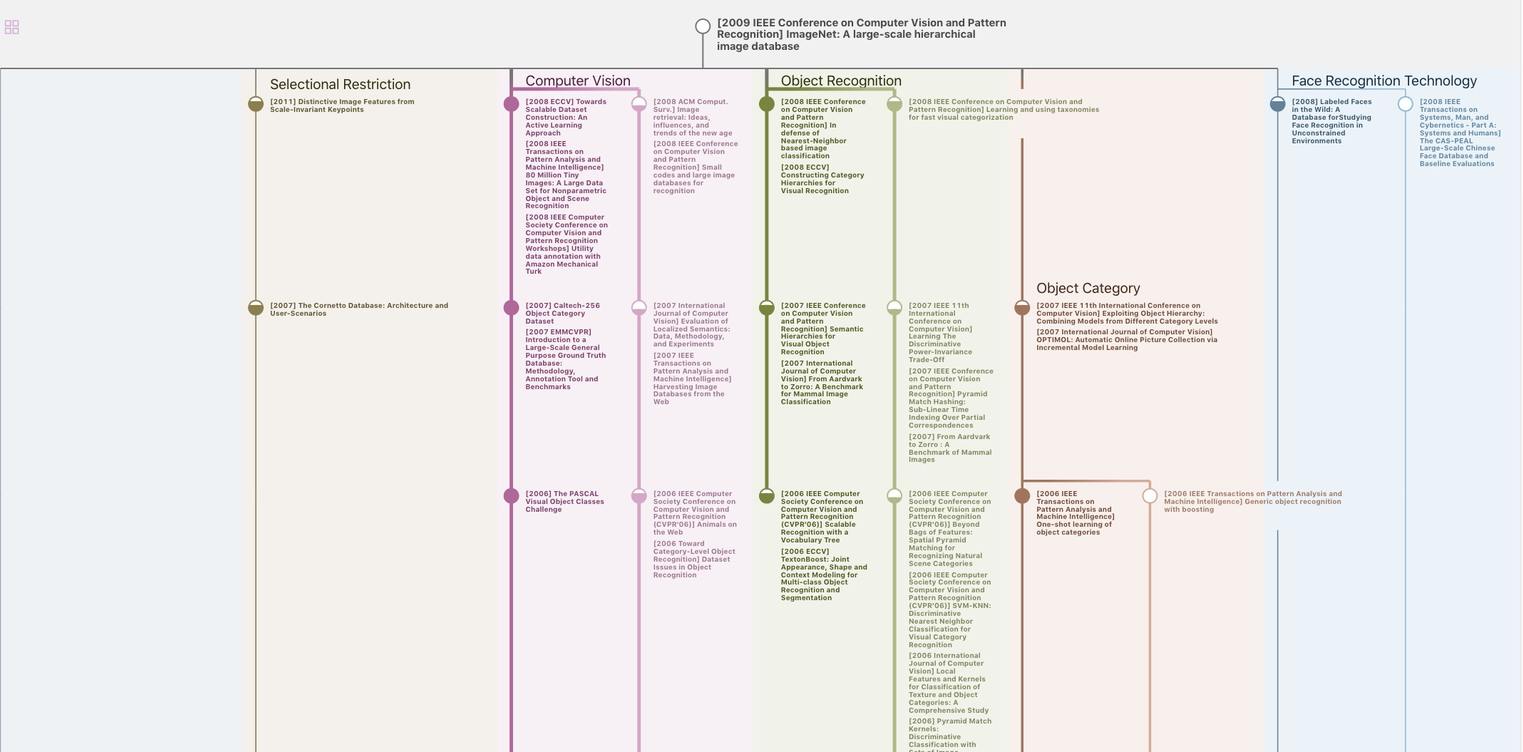
生成溯源树,研究论文发展脉络
Chat Paper
正在生成论文摘要