Keratoconus Severity Detection From Elevation, Topography And Pachymetry Raw Data Using A Machine Learning Approach
IEEE ACCESS(2021)
摘要
Keratoconus (KCN) is an eye condition that affects the cornea. The main objective of this study is to evaluate the accuracy of keratoconus detection from corneal parameters including elevation, topography and pachymetry using machine learning algorithms. We developed several machine learning models to detect keratoconus from corneal elevation, topography and pachymetry parameters that were obtained from 5881 eyes of 2800 patients in Brazil using a Pentacam Scheimpflug instrument. Elevation parameters provided the highest area under the curve (AUC) parameter of 0.99 in detecting normal from keratoconus cases and an AUC of 0.88 in detecting different severity levels when using only three most promising corneal parameters including minimum curvature radius, eccentricity of the cornea and asphericity of the cornea. The developed algorithm can distinguish early KCN eyes from healthy eyes with a high accuracy obtaining an AUC of 0.97. From a clinical point of view the detection of early KCN is very important because KCN patients are usually misdiagnosed due to early symptoms. Results suggest that elevation parameters may retain more useful information for detecting keratoconus than historically believed.
更多查看译文
关键词
Cornea, Surfaces, Diseases, Support vector machines, Machine learning algorithms, Machine learning, Visualization, Artificial intelligence, elevation, topography and pachymetry raw data, machine learning, keratoconus, support vector machine
AI 理解论文
溯源树
样例
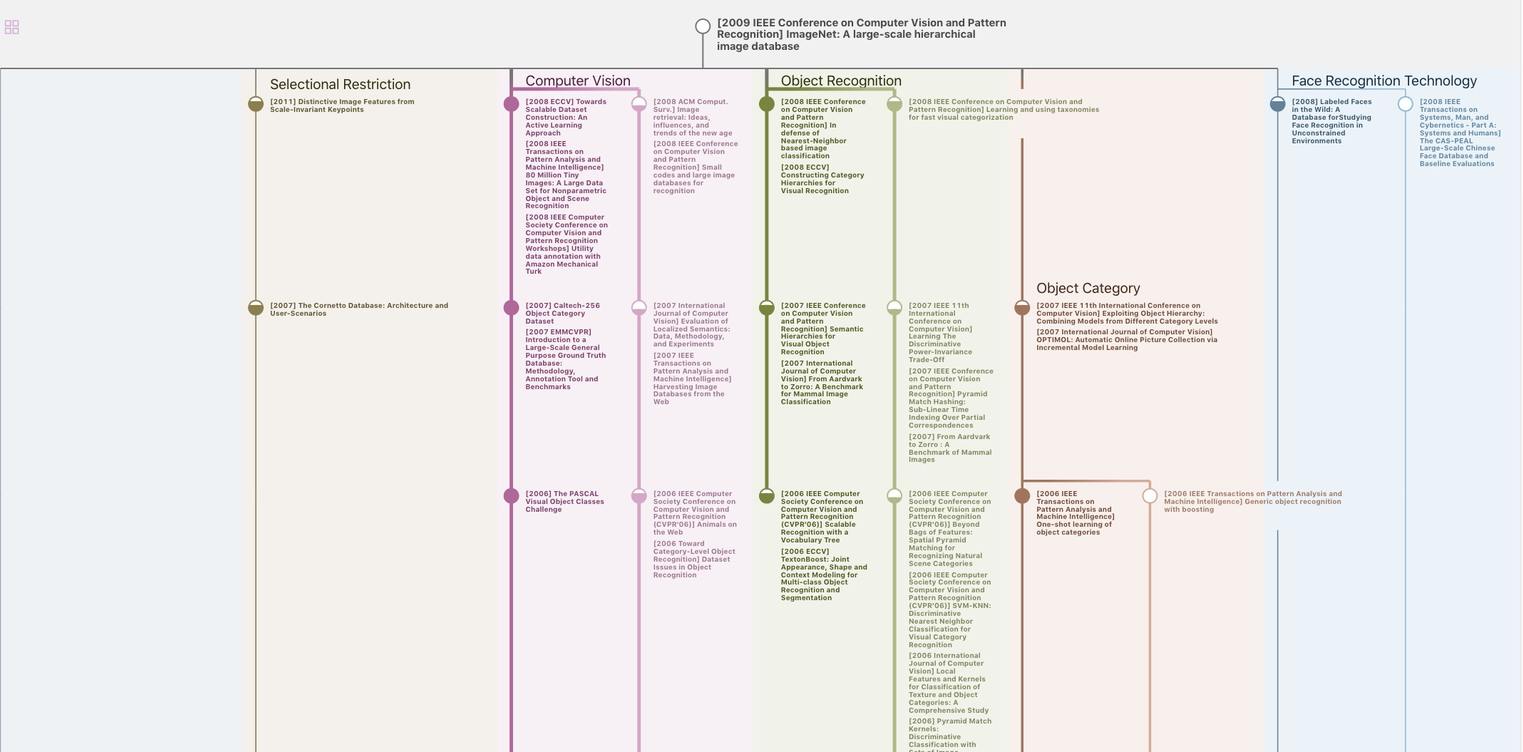
生成溯源树,研究论文发展脉络
Chat Paper
正在生成论文摘要