Inverting Binarizations Of Facial Templates Produced By Deep Learning (And Its Implications)
IEEE TRANSACTIONS ON INFORMATION FORENSICS AND SECURITY(2021)
摘要
We focus on attacks against a biometric authentication system aimed at reconstructing a biometric sample of the subject from the protected template. Such systems include three blocks: feature extraction, binarization, and protection. We propose a new white-box reversing attack on the binarization block that approximates a biometric template given the binary string obtained by the binarization block. The experiments show that the proposed attack reconstructs very accurate approximations that pass the verification threshold when compared to templates produced from the same and different samples of the subject. We then integrate this attack with known attacks on the other two blocks, namely, a variant of a guessing attack to extract the binary string and biometric inversion attack to reconstruct a sample from its template. We instantiate this end-to-end attack on a face authentication system using fuzzy commitments for protection. Facial images reconstructed by the end-to-end attack greatly resemble the original ones. In the simplest attack scenario, more than 83% of these reconstructed templates succeed in unlocking an account (when the system is configured to 0.1% FMR). Even in the "hardest" settings (in which we take a reconstructed image from one system and use it in a different system, with a different feature extraction process) the reconstructed image offers 170 to 210 times higher success rates than the system's FMR.
更多查看译文
关键词
Biometrics (access control), Image reconstruction, Feature extraction, Entropy, Authentication, Privacy, Password, Protection of biometric systems, entropy, deep face recognition, privacy
AI 理解论文
溯源树
样例
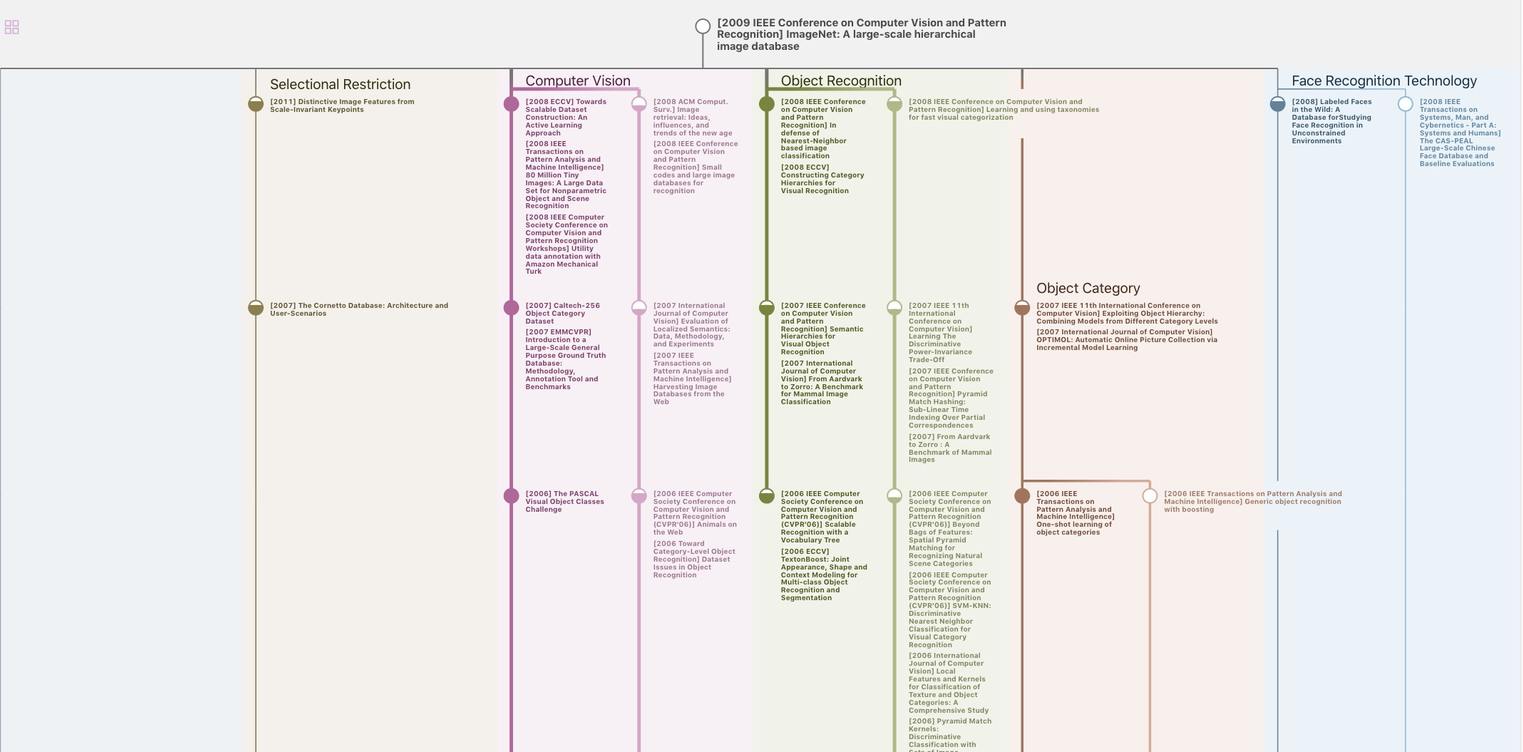
生成溯源树,研究论文发展脉络
Chat Paper
正在生成论文摘要