Linearized Admm Converges To Second-Order Stationary Points For Non-Convex Problems
IEEE TRANSACTIONS ON SIGNAL PROCESSING(2021)
摘要
In this work, a gradient-based primal-dual method of multipliers is proposed for solving a class of linearly constrained non-convex problems. We show that with random initialization of the primal and dual variables, the algorithm is able to compute second-order stationary points (SOSPs) with probability one. Further, we present applications of the proposed method in popular signal processing and machine learning problems such as decentralized matrix factorization and decentralized training of overparameterized neural networks. One of the key steps in the analysis is to construct a new loss function for these problems such that the required convergence conditions (especially the gradient Lipschitz conditions) can be satisfied without changing the global optimal points.
更多查看译文
关键词
Signal processing algorithms, Convex functions, Convergence, Machine learning algorithms, Training, Machine learning, Linear programming, First-order stationary points (FOSPs), second-order stationary points (SOSPs), alternating direction method of multipliers (ADMM), non-convex optimization, neural networks
AI 理解论文
溯源树
样例
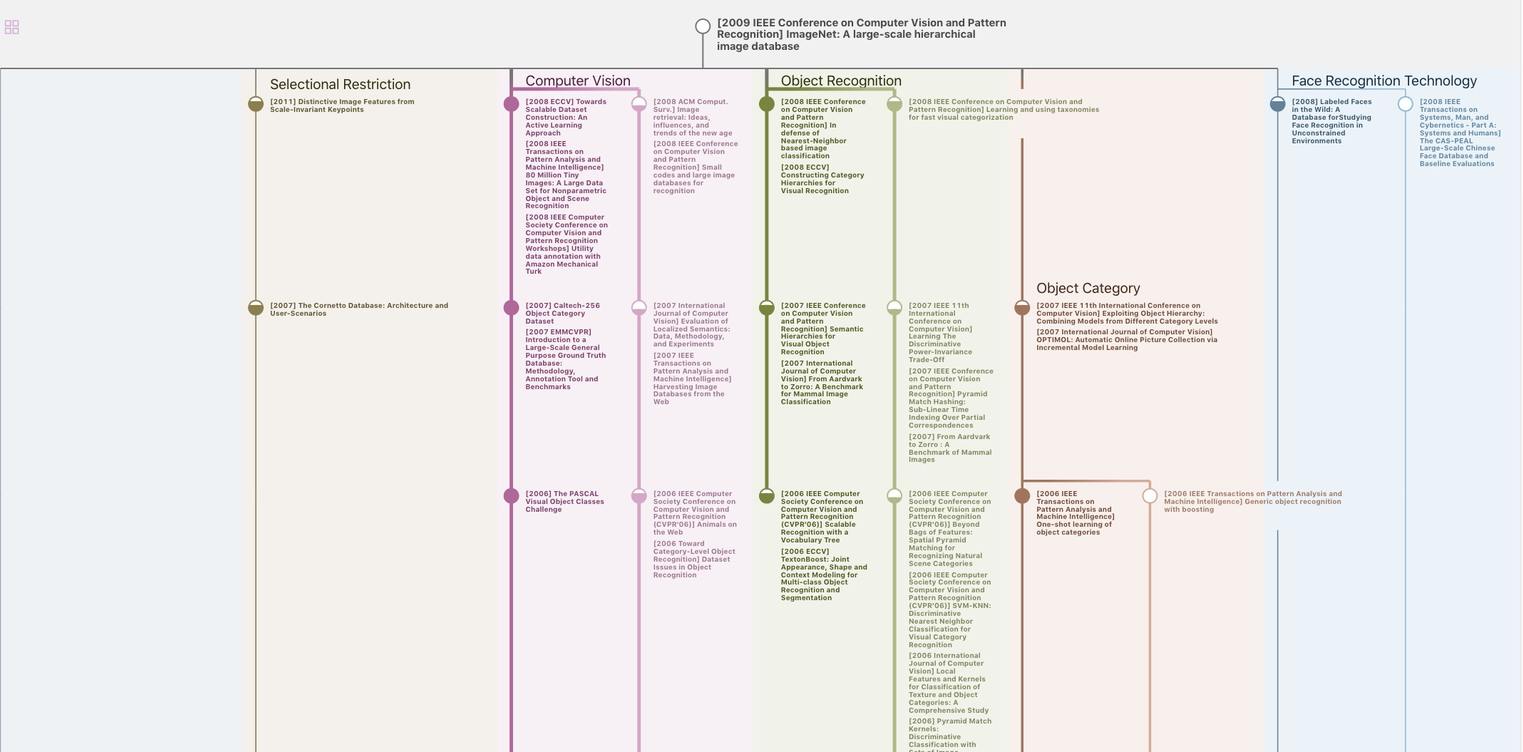
生成溯源树,研究论文发展脉络
Chat Paper
正在生成论文摘要