A Novel, Adaptive Hierarchical Clustering Approach For Independent Component Sorting For Block-Wise Ica Filtering
MEDICAL IMAGING 2021: ULTRASONIC IMAGING AND TOMOGRAPHY(2021)
摘要
Non-contrast ultrasound blood flow imaging is difficult at slow blood flow rates. Singular value decomposition (SVD) and independent component analysis (ICA) are useful for separating tissue, blood, and noise sources for Doppler filtering. In addition, it has been shown that applying SVD and ICA in a block-wise manner further improves source separation; noise within a small block is theoretically more stationary, and thus easier to separate. Yet, there is much discussion on how to select independent components; several methods have been introduced with some success. We present a novel, adaptive hierarchical clustering approach for selecting appropriate independent components for blood flow image filtering that utilizes Kurtosis and Normalized Cross Correlation. Components are clustered based on the Kurtosis and NCC of each component; an optimal number of clusters is chosen using the Silhouette Method. Appropriate clusters are selected based on the Autocorrelation Function of each cluster. Our method was tested on 1 mm/s and 5 mm/s flowrate phantoms containing a 0.6 mm vessel and resulted in average SNR and CNR increases of 6.2 dB and 3.7 dB, respectively, for 1 mm/s blood flow velocities. We demonstrate that our method improves tissue and noise suppression throughout the field of view while maintaining blood flow information.
更多查看译文
关键词
ultrasound, independent component analysis, kurtosis, normalized cross-correlation, hierarchical clustering, block-wise, blood flow imaging
AI 理解论文
溯源树
样例
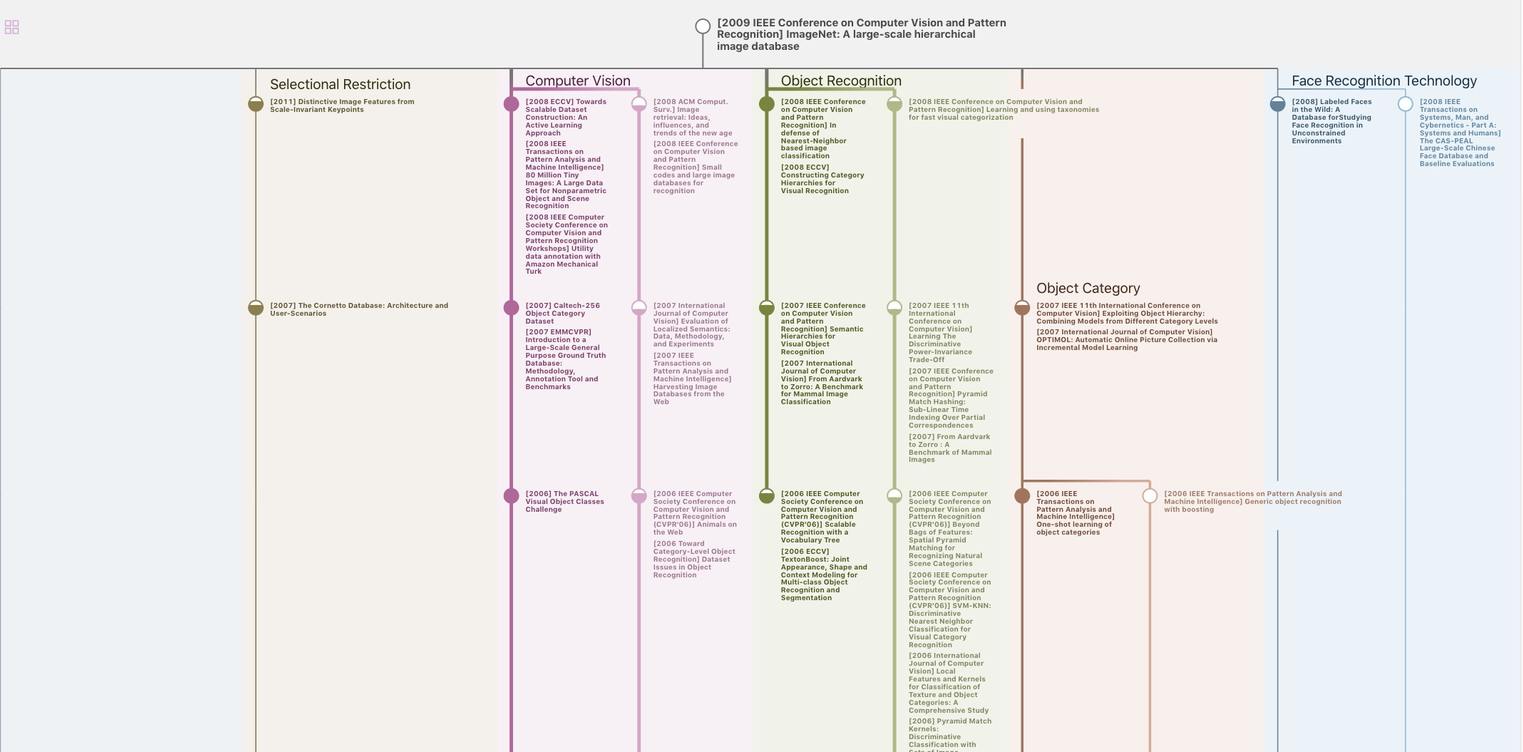
生成溯源树,研究论文发展脉络
Chat Paper
正在生成论文摘要