Recurrent Learning With Clique Structures For Prostate Sparse-View Ct Artifacts Reduction
IET IMAGE PROCESSING(2021)
摘要
In recent years, convolutional neural networks have achieved great success in streak artifacts reduction. However, there is no special method designed for the artifacts reduction of the prostate. To solve the problem, the artifacts reduction CliqueNet (ARCliqueNet) to reconstruct dense-view computed tomography images form sparse-view computed tomography images is proposed. In detail, first, the proposed ARCliqueNet extracts a set of feature maps from the prostate sparse-view CT image by Clique Block. Second, the feature maps are sent to ASPP with memory to be refined. Thenanother Clique Block is applied to the output of ASPP with memory and reconstruct the dense-view CT images. Later on, reconstructed dense-view CT images are used as new input of the original network. This process is repeated recurrently with memory delivering information between these recurrent stages. The final reconstructed dense-view CT images are the output of the last recurrent stage. Our proposed ARCliqueNet outperforms the SOTA (state-of-the-art) general artifacts reduction methods on the prostate dataset in terms of PSNR (peak signal-to-noise ratio) and SSIM (structural similarity). Therefore, we can draw the conclusion that Clique structures, ASPP with memory and recurrent learning are useful for prostate sparse-view CT Artifacts here.
更多查看译文
关键词
X‐rays and particle beams (medical uses),Patient diagnostic methods and instrumentation,Optical, image and video signal processing,X‐ray techniques: radiography and computed tomography (biomedical imaging/measurement),Computer vision and image processing techniques,Biology and medical computing
AI 理解论文
溯源树
样例
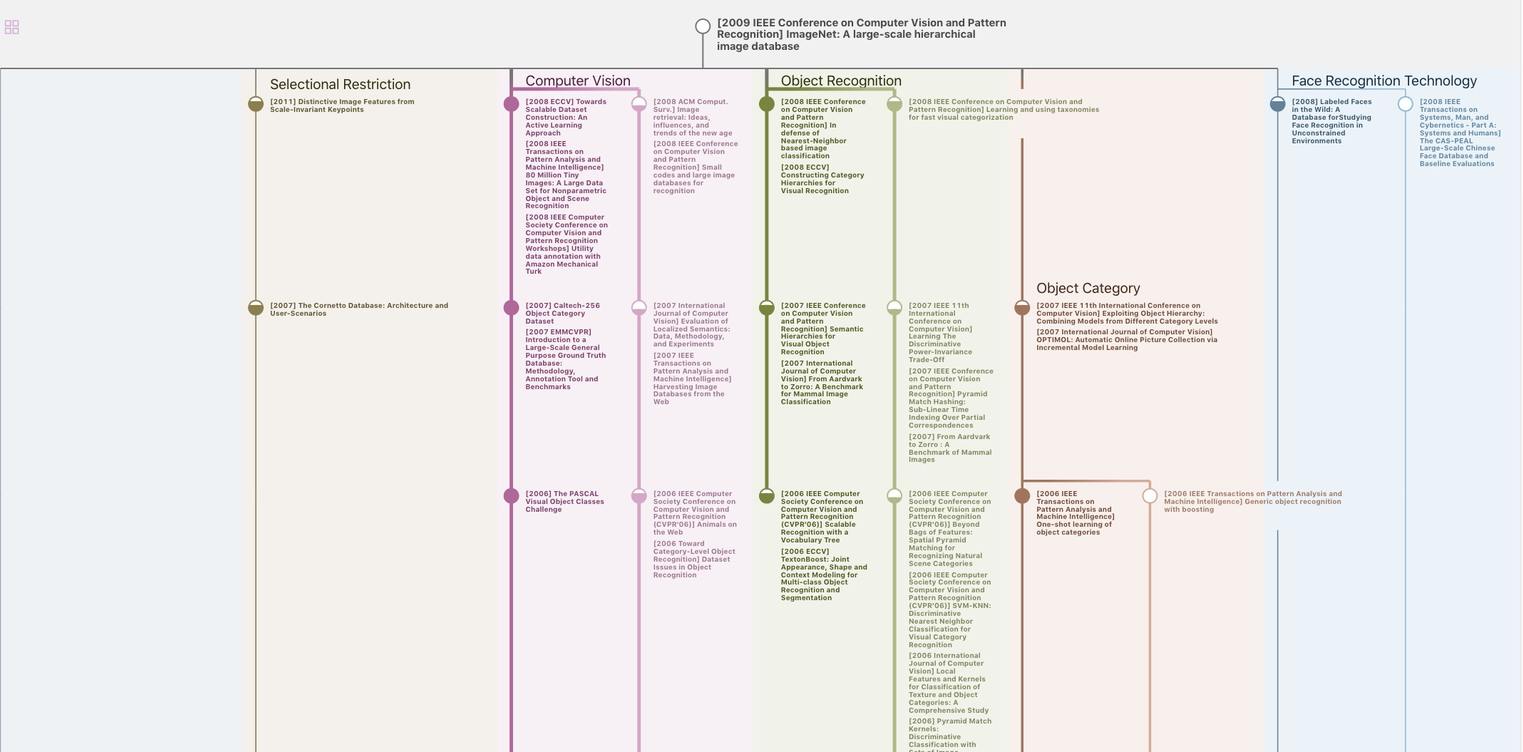
生成溯源树,研究论文发展脉络
Chat Paper
正在生成论文摘要