Machine Learning Techniques For Energy Efficiency And Anomaly Detection In Hybrid Wireless Sensor Networks
ENERGIES(2021)
摘要
Wireless sensor networks (WSNs) are among the most popular wireless technologies for sensor communication purposes nowadays. Usually, WSNs are developed for specific applications, either monitoring purposes or tracking purposes, for indoor or outdoor environments, where limited battery power is a main challenge. To overcome this problem, many routing protocols have been proposed through the last few years. Nevertheless, the extension of the network lifetime in consideration of the sensors capacities remains an open issue. In this paper, to achieve more efficient and reliable protocols according to current application scenarios, two well-known energy efficient protocols, i.e., Low-Energy Adaptive Clustering hierarchy (LEACH) and Energy-Efficient Sensor Routing (EESR), are redesigned considering neural networks. Specifically, to improve results in terms of energy efficiency, a Levenberg-Marquardt neural network (LMNN) is integrated. Furthermore, in order to improve the performance, a sub-cluster LEACH-derived protocol is also proposed. Simulation results show that the Sub-LEACH with LMNN outperformed its competitors in energy efficiency. In addition, the end-to-end delay was evaluated, and Sub-LEACH protocol proved to be the best among existing strategies. Moreover, an intrusion detection system (IDS) has been proposed for anomaly detection based on the support vector machine (SVM) approach for optimal feature selection. Results showed a 96.15% accuracy-again outperforming existing IDS models. Therefore, satisfactory results in terms of energy efficiency, end-to-end delay and anomaly detection analysis were attained.
更多查看译文
关键词
LEACH protocol, EESR protocol, neural networks, support vector machine, energy efficiency, end-to-end delay, intrusion detection system
AI 理解论文
溯源树
样例
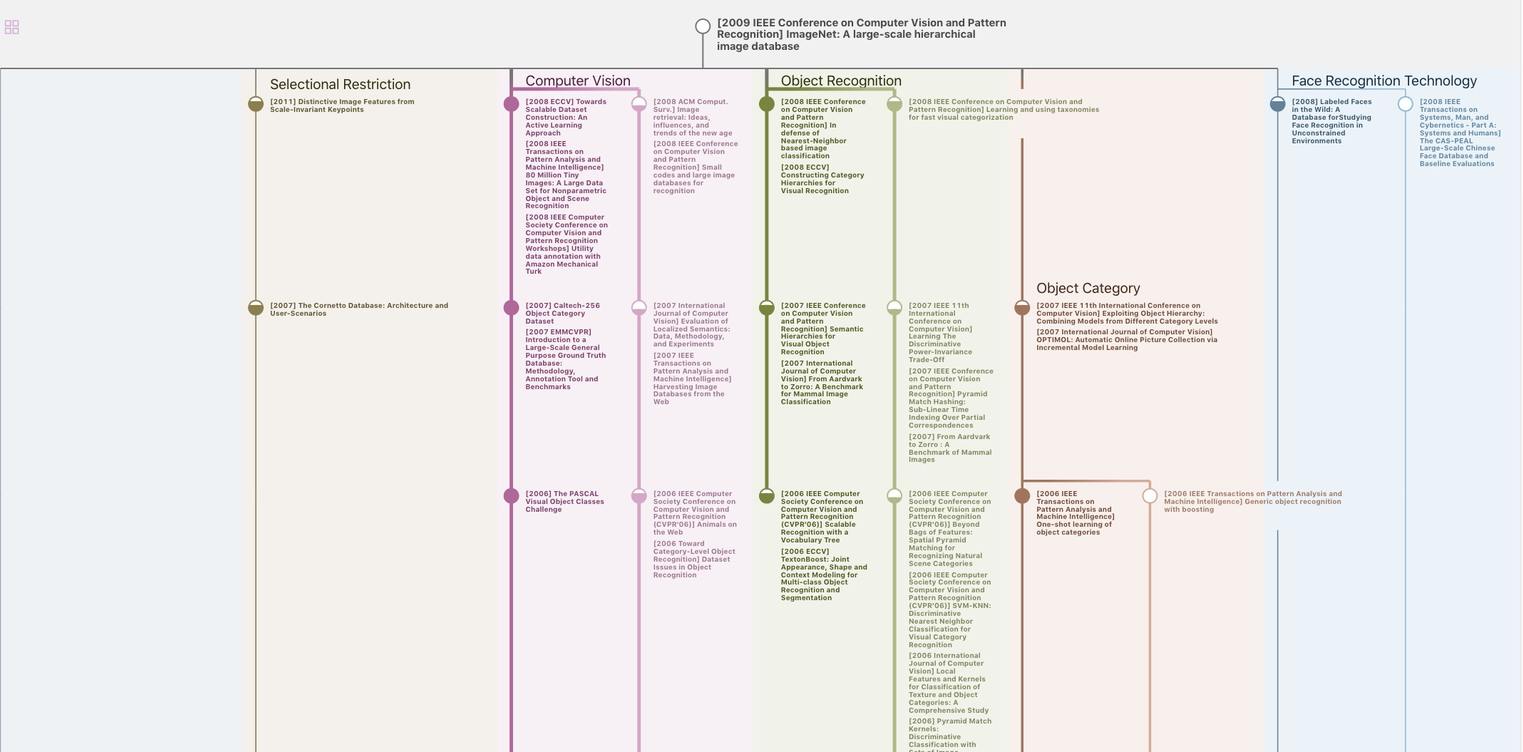
生成溯源树,研究论文发展脉络
Chat Paper
正在生成论文摘要