Estimating Average Treatment Effect On The Treated Via Sufficient Dimension Reduction
STAT(2021)
摘要
In this paper, we propose to use sufficient dimension reduction (SDR) in conjunction with nonparametric techniques to estimate the average treatment effect on the treated (ATT), a parameter of common interest in causal inference. The proposed method is applicable under a general low-dimensional structure in the data, and avoids both the risk of model misspecification and the "curse of dimensionality," for which it often outperforms the existing parametric and nonparametric methods. We develop the theoretical properties of the proposed method, including its asymptotic normality, its asymptotic super-efficiency, and its equivalent form as an augmented inverse probability weighting estimator. We also consider the impact of SDR estimation in the asymptotic studies. These theoretical results are further illustrated by the simulation studies at the end.
更多查看译文
关键词
asymptotic variance, causal inference, sufficient dimension reduction, treatment effect heterogeneity
AI 理解论文
溯源树
样例
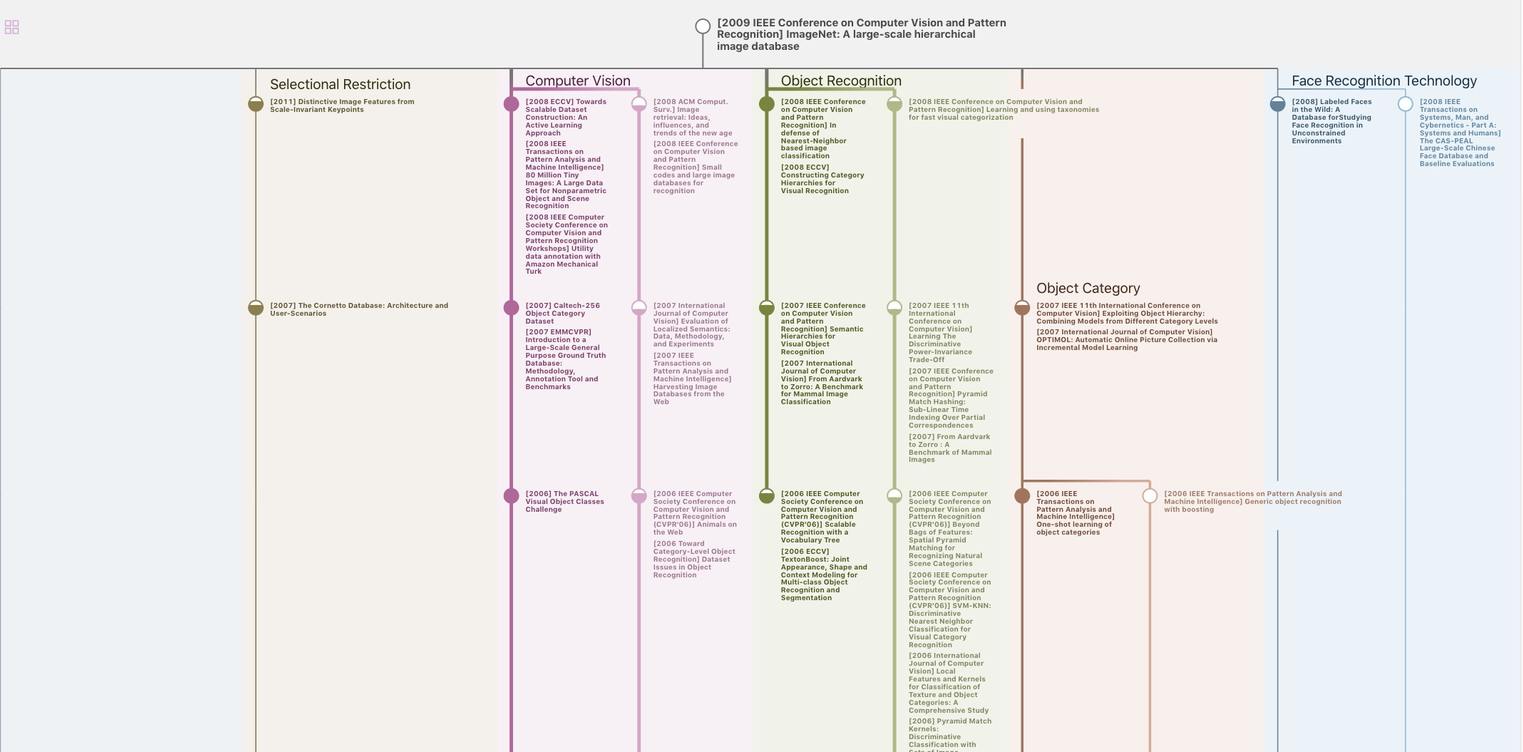
生成溯源树,研究论文发展脉络
Chat Paper
正在生成论文摘要